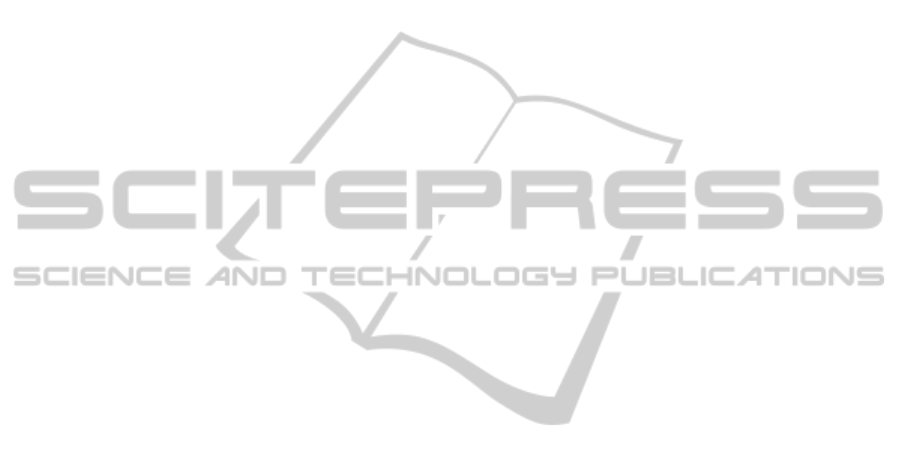
diseases. Therefore, quantification of MRS signals
was performed evaluating the influence of the damp-
ing factor constraint and the number of components
used in the metabolite basis set used for quantifica-
tion. We observed in particular, that the damping fac-
tor in the quantification method AQSES plays an im-
portant role in amplitude estimation. From the quan-
tification results, we examined the residual and ana-
lyzed the fit of the individual components which are
sensible to quantification constraints. The selection of
the metabolites for the basis set is important for quan-
tification, thus an incomplete basis set will provide fits
where one metabolite fits the region that corresponds
to its neighbor. The residual is used to determine the
goodness of the estimates. It is assumed that a good
estimate will lead to residuals resembling pure white
noise. A test of normality would also help to analyze
the residual and determine how close it is to white
noise.
ACKNOWLEDGEMENTS
Maria I. Osorio Garcia and Dr. Flemming U. Nielsen
are Marie Curie research fellows in the EU train-
ing network FAST (www.fast-mrs.eu). Dr. Diana M.
Sima is a postdoctoral fellow of the Fund for Sci-
entific Research-Flanders. Prof. Dr. Uwe Himmelre-
ich and Prof. Dr. ir Sabine Van Huffel are full pro-
fessors at the Katholieke Universiteit Leuven, Bel-
gium. Research supported by: Research Council
KUL: GOA Ambiorics, GOA MaNet, CoE EF/05/006
Optimization in Engineering (OPTEC), IDO 05/010
EEG-fMRI, IDO 08/013 Autism, IOF-KP06/11 Fun-
Copt, several PhD/postdoc & fellow grants; Flem-
ish Government: FWO: PhD/postdoc grants, projects:
FWO G.0302.07 (SVM), G.0341.07 (Data fusion),
G.0427.10N (Integrated EEG-fMRI) research com-
munities (ICCoS, ANMMM); IWT: TBM070713-
Accelero, TBM070706-IOTA3, TBM080658-MRI
(EEG-fMRI), PhD Grants; Belgian Federal Science
Policy Office: IUAP P6/04 (DYSCO, ‘Dynami-
cal systems, control and optimization’, 2007-2011);
ESA PRODEX No 90348 (sleep homeostasis), EU:
FAST (FP6-MC-RTN-035801), Neuromath (COST-
BM0601), KU Leuven center of Excellence ’MO-
SAIC’.
REFERENCES
Bottomley, P. (1984). Selective volume method for perform-
ing localized NMR spectroscopy. in U.S patent, (4 480
228).
Cudalbu, C., Cavassila, S., Ratiney, H., Grenier, D.,
Briguet, A., and Graveron-Demilly, D. (2006). Esti-
mation of metabolite concentrations of healthy mouse
brain by magnetic resonance spectroscopy at 7T.
Comptes Rendus Chimie, 9(3-4):534 – 538.
Grage, H. and Akke, M. (2003). A statistical analysis of
NMR spectrometer noise. Journal of Magnetic Reso-
nance, 162(1):176 – 188.
Gruetter, R. (1993). Automatic localized in vivo adjustment
of all first- and second- order shim coils. Magnetic
Resonance in Medicine, 29(6):804–811.
Klose, U. (1990). In vivo proton spectroscopy in presence
of eddy currents. Magnetic Resonance in Medicine,
14(1):26 – 30.
Laudadio, T., Mastronardi, N., Vanhamme, L., Van Hecke,
P., and Van Huffel, S. (2002). Improved Lanczos algo-
rithms for blackbox MRS data quantitation. Jorunal
of Magnetic Resonance, 157(2):292 – 297.
Pfeuffer, J., Tkc, I., Provencher, S. W., and Gruetter, R.
(1999). Toward an in vivo neurochemical profile:
Quantification of 18 metabolites in short-echo-time
1
H NMR spectra of the rat brain. Journal of Magnetic
Resonance, 141(1):104 – 120.
Pijnappel, W. W. F., van den Boogaart, A., de Beer, R., and
van Ormondt, D. (1992). SVD-based quantification
of magnetic resonance signals. Journal of Magnetic
Resonance, 97(1):122 – 134.
Poullet, J.-B., Sima, D., Simonetti, A., De Neuter, B.,
Vanhamme, L., Lemmerling, P., and Van Huffel, S.
(2007). An automated quantitation of short echo time
MRS spectra in an open source software environment:
AQSES. NMR in Biomedicine, 20(5):493 – 504.
Provencher, S. (2001). Automatic quantitation of local-
ized in vivo
1
H spectra with LCModel. NMR in
Biomedicine, 14(4):260–264.
Ratiney, H., Coenradie, Y., Cavassila, S., van Ormondt,
D., and Graveron-Demilly, D. (2004). Time-domain
quantitation of
1
H short echo-time signals: back-
ground accommodation. MAGMA, 16(6):284 – 296.
Slotboom, J., Nirkko, A., Brekenfeld, C., and van Ordmont,
D. (2009). Reliability testing of in vivo magnetic reso-
nance spectroscopy (MRS) signals and signal artifact
reduction by order statistics filtering. Measurement
Science and Technology, 20(104030):14pp.
Stefan, D., Di Cesare, F., Andrasescu, A., Popa, E.,
Lazariev, A., Vescovo, E., Strbak, O., Williams, S.,
Starcuk, Z., Cabanas, M., van Ormondt, D., and
Graveron-Demilly., D. (2009). Quantitation of mag-
netic resonance spectroscopy signals: the jMRUI soft-
ware package. Measurement Science and Technology,
20(10):104035(9pp).
Tk´aˇc, I., Starˇcuk, Z., Choi, I.-Y., and Gruetter, R. (1999). In
vivo
1
H NMR spectroscopy of rat brain at 1ms echo
time. Magnetic Resonance in Medicine, 41(4):649–
656.
DAMPING FACTOR CONSTRAINTS AND METABOLITE PROFILE SELECTION INFLUENCE MAGNETIC
RESONANCE SPECTROSCOPY DATA QUANTIFICATION
181