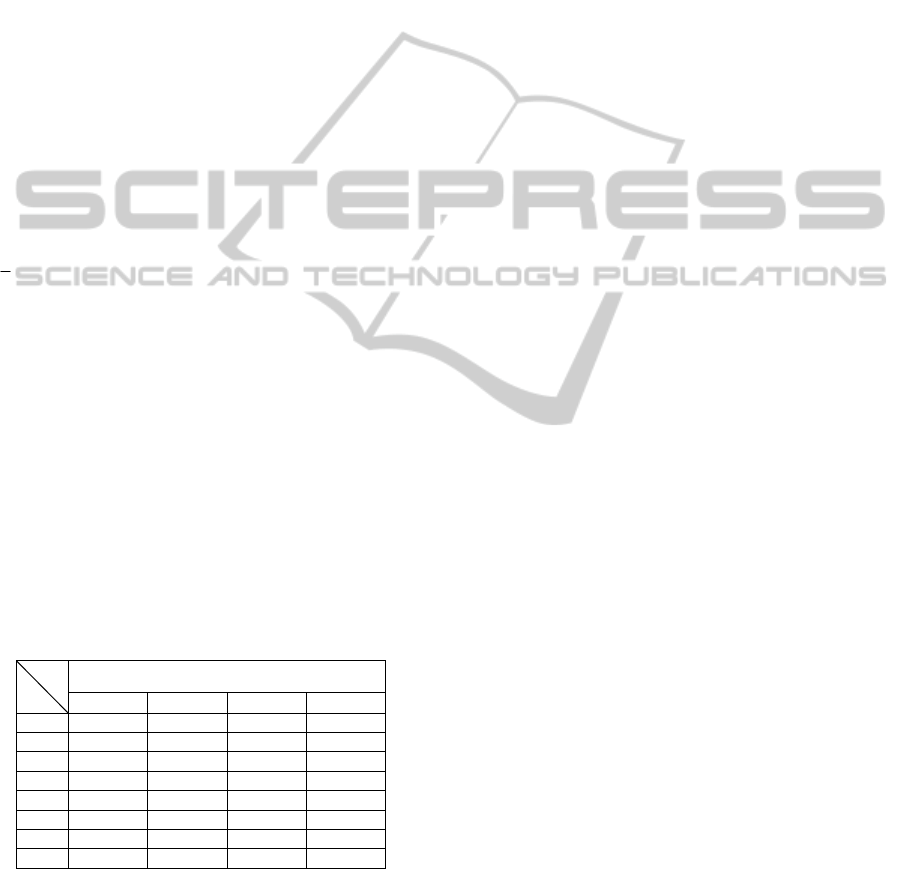
characteristics for supplier selection. In the case, the
LED assembly products require a minimal
capability. The minimal requirement of the LED
assembly characteristic is
T
k
S = 1.20.
For the product type we investigated, the upper
and lower specification limits of the distance
between LEDs are set to 12.2 and 15.4 millimeter. In
addition, the upper and lower specification limits of
the length of LED assembly are set to 19.5 and 21.5
millimeter. The millimeter is used as the unit for the
two specifications.
To determine whether the new Supplier (Supplier
II) provides a better process capability of the LED
assembly products than current Supplier (Supplier I),
we perform the hypothesis testing:
02 1
:
TT
kpk
SS≤
versus
12 1
:
TT
kpk
SS> . We collected two data sets
from suppliers I and II with
1
n =
2
n =100. Based on
the observations, we compute the sample estimate
ˆ
T
k
S of
T
k
S
for both suppliers. The sample average
(
j
), sample standard deviation (
j
) and
ˆ
kj
S for
each characteristic are also calculated. Thus, we can
obtain that
1
ˆ
T
k
S = 1.104 and
2
ˆ
T
k
S =1.415.
We calculated the test statistic W =
2
ˆ
T
pk
S
1
ˆ
T
k
S =
0.311 for the proposed supplier selection method. In
the paper, we used a commercial computation
software to compute the critical value (see Table 2)
that is very useful to help us to make the decision for
the hypothesis testing.
The input parameters of the
program involving the values of
1
T
k
S
,
2
T
k
S
,
the
corresponding sample sizes n
1
, n
2
, C, and α.
Table 2: Critical values for rejecting
21
TT
kpk
SS≤ with
12
nn= =30(10)100 and
=0.05.
n
12
TT
pk pk
SSC
=
1.0 1.2 1.4 1.6
30 0.3003 0.3604 0.4204 0.4805
40 0.2601 0.3121 0.3641 0.4161
50 0.2326 0.2791 0.3257 0.3722
60 0.2123 0.2548 0.2973 0.3398
70 0.1966 0.2359 0.2752 0.3146
80 0.1839 0.2207 0.2575 0.2942
90 0.1734 0.2081 0.2427 0.2774
100 0.1645 0.1974 0.2303 0.2632
In the case, we use the Pearson-Correlation test
to justify the correlation. The result shows the
relationship among the two characteristics can be
regarded as independent. In addition, we run the
developed program with
1
n =
2
n =100,
12
TT
kpk
SS= =1.20, and α= 0.05 to obtain the critical
value as 0.1974 for the presented supplier selection
method (it also can be found in Table 2).
Since the testing statistic W = 0.311 > 0.1974, we
can conclude that the new supplier is superior than
the current supplier with 95% confidence level.
5 CONCLUSIONS
Supplier selection problem in light emitting diode
assembly process is very important and frequently
occurred. Since the multiple characteristics should
be considered in the light emitting diode (LED)
assembly process for supplier selection, in the paper,
we presented and applied a supplier selection
method based on process yield index to provide
exact measures on process yield with multiple
characteristics. For users’ convenience in applying
the supplier selection method in LED assembly
process, the critical values of the hypothesis testing
with various sample sizes are presented and
tabulated. The supplier selection method which is
applied in the LED assembly process is very useful
for factory in-plant applications.
ACKNOWLEDGEMENTS
The authors would like to express their gratitude to
the anonymous referees for their valuable comments
and careful readings, which greatly improved the
presentation of this paper. This paper was supported
in part by the National Science Council, Taiwan,
ROC, under the contracts NSC 100-2410-H-424-011
and NSC 100-2628-E-011-013-MY3.
REFERENCES
Aissaoui, N., Haouari, M., Hassini, E., (2007). Supplier
selection and order lot sizing modeling: A review.
Computers & Operations Research, 34, 3516-3540.
Boyles, R. A., (1994). Process capability with asymmetric
tolerances. Communication in Statistics: Simulation
and Computation, 23(3), 615-643.
Chan, L. K., Cheng, S. W., Spiring, F. A., (1988). A new
measure of process capability Cpm. Journal of Quality
Technology, 20(3), 162-175.
Chen, K. S., Pearn, W. L., Lin, P. C., (2003). Capability
measures for processes with multiple characteristics.
Quality and Reliability Engineering International,
19(2), 101-110.
Degraeve, Z., Labro, E., Roodhooft, F., (2000). An
SupplierSelectionbasedonProcessYieldforLEDManufacturingProcesses
597