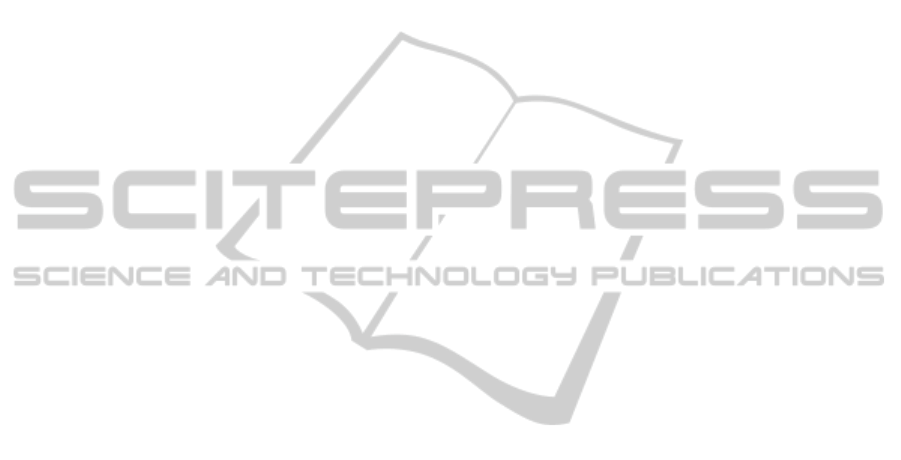
ACKNOWLEDGEMENTS
The Low Carbon Vehicle Technology Project
(LCVTP) was a collaborative research project be-
tween leading automotive companies and research
partners, revolutionising the way vehicles are pow-
ered and manufactured. The project partners included
Jaguar Land Rover, Tata Motors European Techni-
cal Centre, Ricardo, MIRA LTD., Zytek, WMG and
Coventry University. The project included 15 au-
tomotive technology development work-streams that
will deliver technological and socio-economic out-
puts that will benefit the West Midlands Region. The
19 million project was funded by Advantage West
Midlands (AWM) and the European Regional Devel-
opment Fund (ERDF).
The authors would like to thank the anonymous
reviewers for their insightful comments.
REFERENCES
Alahmer, A., Mayyas, A., Mayyas, A., Omar, M., and
Shan, D. (2011). Vehicular thermal comfort models; a
comprehensive review. Applied Thermal Engineering,
31:995–1002.
Arens, E., Zhang, H., and Huizenga, C. (2006). Partial
and whole-body thermal sensation and comfort, part
ii: Non-uniform environmental conditio. Journal of
Thermal Biology, 31:60–66.
Bogdan, A. (2011). Case study assessment of local and gen-
eral thermal comfort by means of local skin temper-
ature. International Journal of Ventilation, 10:291–
300.
Busl, M. (2011). Design of an energy-efficient climate con-
trol algorithm for electric cars. Master’s thesis, Lund
University.
Cheng, Y., Niu, J., and Gao, N. (2012). Thermal comfort
models: A review and numerical investigation. Build-
ing and Environment, 47:13–22.
Cisternino, M. (1999). Thermal climate in cabs and mea-
surement problems. In CABCLI Consortium.
Fanger, P. (1970). Thermal Comfort. Danish Technical
Press.
Fanger, P. (1973). Assessment of man’s thermal comfort
in practice. British Journal of Industrial Medicine,
30:313–324.
Farzaneh, Y. and Tootoonchi, A. (2008). Controlling au-
tomobile thermal comfort using optimized fuzzy con-
troller. Applied Thermal Engineering, 28:1906–1917.
ISO (2005). Ergonomics of the Thermal Environment.
Luo, X., Hou, W., Li, Y., and Wang, Z. (2007). A fuzzy
neural network model for predicting clothing thermal
comfort. Computers and Mathematics with Applica-
tions, 53:1840–1846.
Matsunaga, K., Sudo, F., Tanabe, S., and Madsen, T. (1993).
Evaluation and measurement of thermal comfort in the
vehicles with a new thermal manikin. Technical re-
port, SAE Paper.
Nakamura, M., Yoda, T., Crawshaw, L., Yasuhara, S., Saito,
Y., Kasuga, M., Nagashima, K., and Kanosue, K.
(2008). Regional differences in temperature sensation
and thermal comfort in humans. Journal of Applied
Physiology, 105:1897–1906.
Nilsson, H. (2004). Comfort Climate Evaluation with Ther-
mal Manikin Methods and Computer Simulation Mod-
els. PhD thesis, Royal Institute of Technology.
Nilsson, H. and Holmer, I. (2002). Definitions and mea-
surements of equivalent temperature. Technical re-
port, The Climate Group, National Institute for Work-
ing Life, Solna, Sweden.
Orosa, J. (2009). Research on general thermal comfort mod-
els. European Journal of Scientific Research, 2:217–
227.
Taniguchi, Y., Hiroshi, A., and Kenji, F. (1992). Study
on car air conditioning system controlled by car oc-
cupants’ skin temperatures - part 1: research on a
method of quantitative evaluation of car occupants.
Technical report, SAE Paper.
Ueda, M. and Taniguchi, Y. (2000). The prediction of the
passenger’s thermal sensation level using a neural net-
work and its application to the automobile hvac con-
trol.
van Hoof, J. (2008). Forty years of fanger’s model of ther-
mal comfort:l comfort for all? Indoor Air Journal,
18:182–201.
Wang, D., Zhang, H., Arens, E., and Huizenga, C. (2007).
Observations of upper-extremity skin temperature and
corresponding overall-body thermal sensations and
comfort. Building and Environment, 42:3933–3943.
Zhang, H. (2003). Human Thermal Sensation and Comfort
in Transient and Non-Uniform Thermal Environments.
PhD thesis, University of California, Berkeley.
ICINCO2014-11thInternationalConferenceonInformaticsinControl,AutomationandRobotics
776