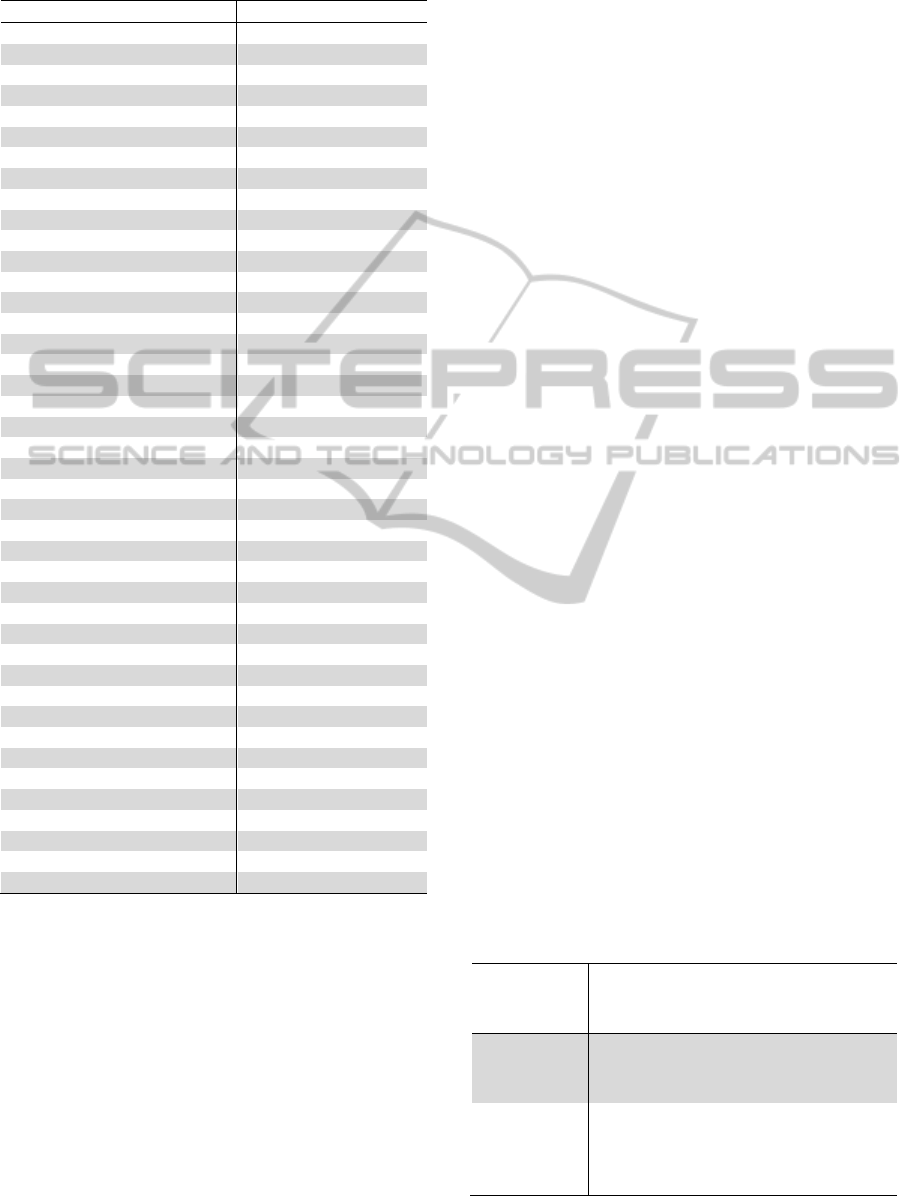
Table 3: Main results of analysis of all participating
households.
Variable Coefficient t value
DUM1_1305 (*1) -1,838 *** -9.212
DUM1_1306 (*1) -2,632 *** -12.06
DUM1_1309 (*1) -1,992 *** -9.230
DUM1_1312 138.0 0.7339
DUM1_1403 -87.23 -0.693
DUM2_1307 (*1) -1,898 *** -6.437
DUM2_1310 (*1) -2,267 *** -14.51
DUM2_1401 343.3 ** 1.961
DUM2_1404 -1,852 *** -12.57
DUM3_1308 118.6 0.3208
DUM3_1311 -1,027 *** -8.146
DUM3_1402 285.4 * 1.753
DUM3_1405 (*2) -2,510 *** -16.72
DUM3_1406 (*2) -2,612 *** -14.52
DUM3_1407 (*2) -1,623 *** -6.625
DUM3_1408 -344.6 -1.296
DUM3_1409 (*2) -2,600 *** -13.06
DUM3_1410 (*2) -2,662 *** -15.32
ln(VC+1)×DUM1_1305 59.94 0.8199
ln(VC+1)×DUM1_1306 59.31 0.8148
ln(VC+1)×DUM1_1309 -151.4 -1.457
ln(VC+1)×DUM1_1312 661.9 *** 3.476
ln(VC+1)×DUM1_1403 601.3 ** 4.175
ln(VC+1)×DUM2_1307 -497.9 *** -4.127
ln(VC+1)×DUM2_1310 21.97 0.1480
ln(VC+1)×DUM2_1401 342.5 1.498
ln(VC+1)×DUM2_1404 949.6 *** 5.801
ln(VC+1)×DUM3_1308 -189.4 -1.251
ln(VC+1)×DUM3_1311 468.8 ** 2.565
ln(VC+1)×DUM3_1402 646.4 *** 3.919
ln(VC+1)×DUM3_1405 805.6 *** 5.213
ln(VC+1)×DUM3_1406 -14.78 -0.1529
ln(VC+1)×DUM3_1407 (*3) -640.4 *** -4.173
ln(VC+1)×DUM3_1408 (*3) -1,303 *** -8.063
ln(VC+1)×DUM3_1409 (*3) -382.4 *** -3.384
ln(VC+1)×DUM3_1410 (*3) -560.1 *** -5.152
DUM20P 29.51 0.0766
DUM30P 281.1 0.9736
DUM40P (*4) 1,123 *** 4.998
ln(VC+1)×DUM20P 150.7 0.5276
ln(VC+1)×DUM30P -123.2 -0.444
ln(VC+1)×DUM40P (*4) -1,049 *** -3,653
between the frequency of viewing a tablet PC and
the dummy variable for the feedback pattern are
statistically significant at the 1 percent level and
have negative value from July to October, 2014,
which are indicated in (*3) in Table 3. Those
coefficients range from approximately -380 to -
1,300.
However, particularly in the winter and spring
seasons, the significant coefficients of the same
cross terms are sometimes positive. It is considered
to be difficult to reduce electric power consumption
in winter, despite the fact that the actively
participating households confirmed their
consumption level frequently via the tablet PCs. The
differing effects between summer and winter may be
related to consumers’ perception gap between
subjective savings and real savings, as reported by
Attari et al. (2010).
On the other hand, only the coefficients of
DUM40P and ln(VC+1)×DUM40P are statistically
significant among the variables related to dynamic
pricing, which are indicated in (*4) in Table 3. The
result in which DUM40P has a positive coefficient
and ln(VC+1)×DUM40P has a negative coefficient
indicates that households that viewed their tablet PC
frequently reduced electric power consumption
when the deduction rate was 40 points.
Table 4 shows the estimated effect of real-time
feedback and dynamic pricing compared with the
mean daily consumption of the participating
households during the dynamic pricing experiment.
Households viewing electric power consumption on
the tablet PC three times per day are estimated to
have reduced power consumption by 20.1 percent
through real-time feedback and 2.1 percent through
dynamic pricing.
Regarding the long-term effect of electric power
consumption reduction by real-time information
feedback, we confirmed almost the same level of
coefficients of feedback pattern dummy variables
between May/June/July/September/October of 2013
(DUM1_1305, DUM1_1306, DUM2_1307,
DUM1_1309, DUM2_1310: -1,838 to -2.632, which
are indicated in (*1) in Table 3) and the same
months of 2014 (DUM3_1405, DUM3_1406,
DUM3_1407, DUM3_1409, DUM3_1410: -1,623 to
-2,662, which are indicated in (*2) in Table 3). At
least for these two years, except in August, real-time
information feedback was found to work
continuously as an effective electric power demand
reduction measure. These results are quite different
from those of the previous studies such as Houde et
al. (2013) that indicated significant reductions
Table 4: Estimated reduction rate achieved by feedback
and dynamic pricing during the dynamic pricing
experiment.
Frequency of viewing
tablet PC per day
0 1 2 3
Real-time
feedback
Pattern 3
-
16.7%
-
18.4%
-
19.4%
-
20.1%
Dynamic
pricing
Deduction
rate: 40
+7.2% +2.5% -0.2% -2.1%
SMARTGREENS2015-4thInternationalConferenceonSmartCitiesandGreenICTSystems
206