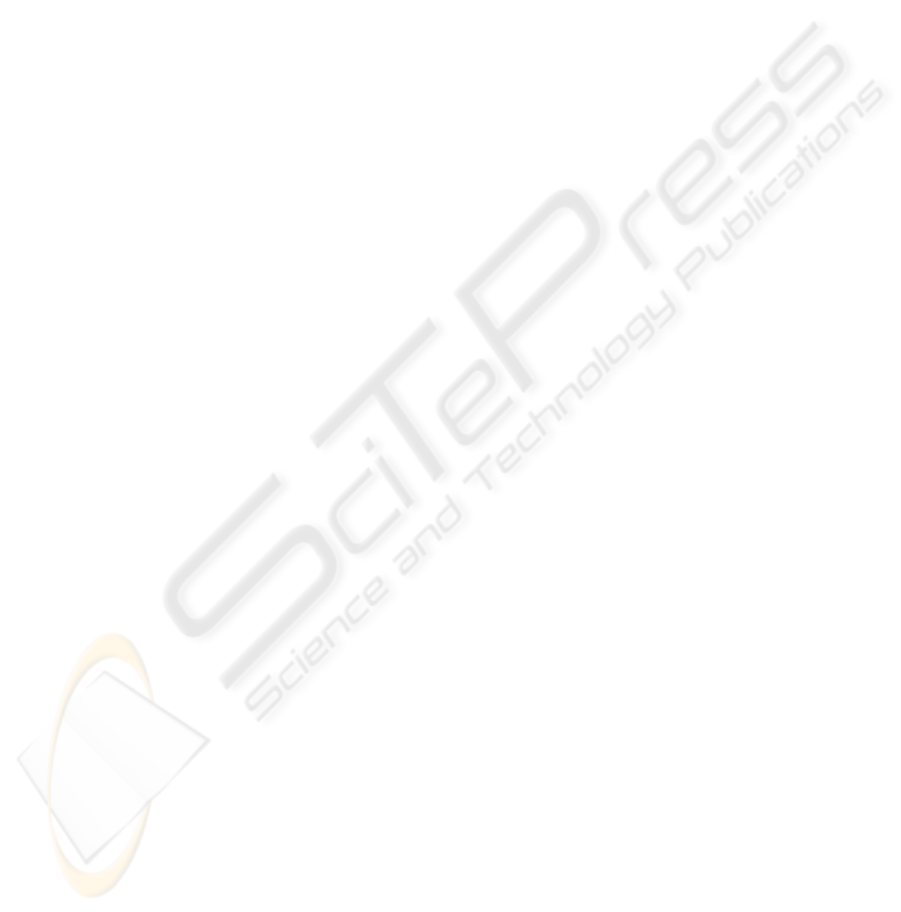
ticketing machines (van Dijk, Minoch and Laing,
2007). The literature on this topic explains that
people are willing to use a SST if it matches their
attitudes toward technology in general and towards
technology-based self-service options in particular
(Bobbitt and Dabholkar, 2001).
2.2 The Theory of Planned Behaviour
(TPB)
According to the TPB, an individual’s behaviour can
be explained by his or her behavioural intention,
which is influenced by attitude, the subjective norm
and perceived behavioural control (Ajzen, 1991). In
our context, the TPB states that a consumer’s
intention to use a SST is simultaneously determined
by such factors as a positive or negative evaluative
effect about using the technology.
2.3 The Technology Acceptance Model
(TAM)
Based on Fishbein and Ajzen’s (1975) theory of
reasoned action (TRA), the TAM focuses on the role
of ease of use and usefulness in predicting the
attitude toward using a new technology (Davis,
1986). Following prior research findings and using
the TPB as a basic structure, attitude is decomposed
by incorporating perceived usefulness and perceived
ease of use. The integrated model proposed here
combines the TPB and the TAM and explains the
adoption of SSTs for online services.
We then infer that the casual factors of the TPB,
the TAM, and an integrated TPB/TAM model will
have positive relationships with the behavioural
intention. H1, H2 and H3 are initiated underlying
TPB, while H4, H5, H6, and H7 are proposed based
on the TAM. These hypotheses were further verified
for their validity by empirical data.
H1: Attitude toward using a SST will have a
positive impact on behavioural intention.
H2: The subjective norm will have a positive
impact on behavioural intention.
H3: Perceived behavioural control will have a
positive impact on behavioural intention.
H4: The perceived usefulness of SSTs will have
a positive impact on attitude toward using a SST.
H5: The perceived usefulness of SSTs will have
a positive impact on behavioural intention.
H6: The perceived ease of use of SSTs will have
a positive impact on attitude toward using a SST.
H7: The perceived ease of use of SST will have
a positive impact on perceived usefulness of a
SST.
3 METHODS
3.1 Sample and Procedure
Questionnaires were distributed to a sample of 280
adult consumers in Taiwan. Participation in the
survey was completely voluntary and anonymous.
The definition and classification of SSTs used in this
survey were based on the typology of Meuter, et al.
(2000), which is one of the few comprehensive and
empirically based SST classification schemes.
Respondents were asked to evaluate their
experiences with SSTs that they had used most
frequently, as well as their resulting behavioural
intentions. Respondents’ evaluations covered a wide
range of SST providers, including post offices,
banks, stock exchanges, cinemas, railways, airlines,
bookstores, and internet services.
The sample was comprised of 48.2% males and
51.8% females, and the age range of the participants
was 18-45. Most respondents were university-
educated with a monthly personal income level of
less than NT $ 20,000.
3.2 Measures
Twenty-one measure variables were used to reflect
the components of an integrated TPB/TAM model.
Subjects responded using a five-point scale (1 =
completely disagree; 5 = completely agree) to
questions about the intention to use SSTs. The scales
of behavioural intention (BI) to use a SST are
borrowed from Venkatesh and Davis (1996, 2000).
Attitude (ATT) was adapted from the measurement
defined by Bhattacherjee (2000). For measuring
subjective norm (SN) and perceived behavioural
control (PBC), the items are adopted from Taylor
and Todd (1995) and Bhattacherjee (2000). The
perceived usefulness (PU) and the perceived ease of
use (PEOU) scales are adopted from Venkatesh and
Davis (1996, 2000). In addition, some demographic
variables, such as sex, age and college year, were
collected.
4 RESULTS
First, we performed empirical exploratory factor
analysis (EFA) and conducted reliability analysis to
confirm the reliability of the variables adopted in the
study. The reliability of all instruments assessed by
the Cronbach’s reliability coefficients ranged from
0.71 to 0.87 and exceeded the .60 lower limit of
acceptability (Hair, et al., 1998). Next, confirmatory
ICEIS 2009 - International Conference on Enterprise Information Systems
162