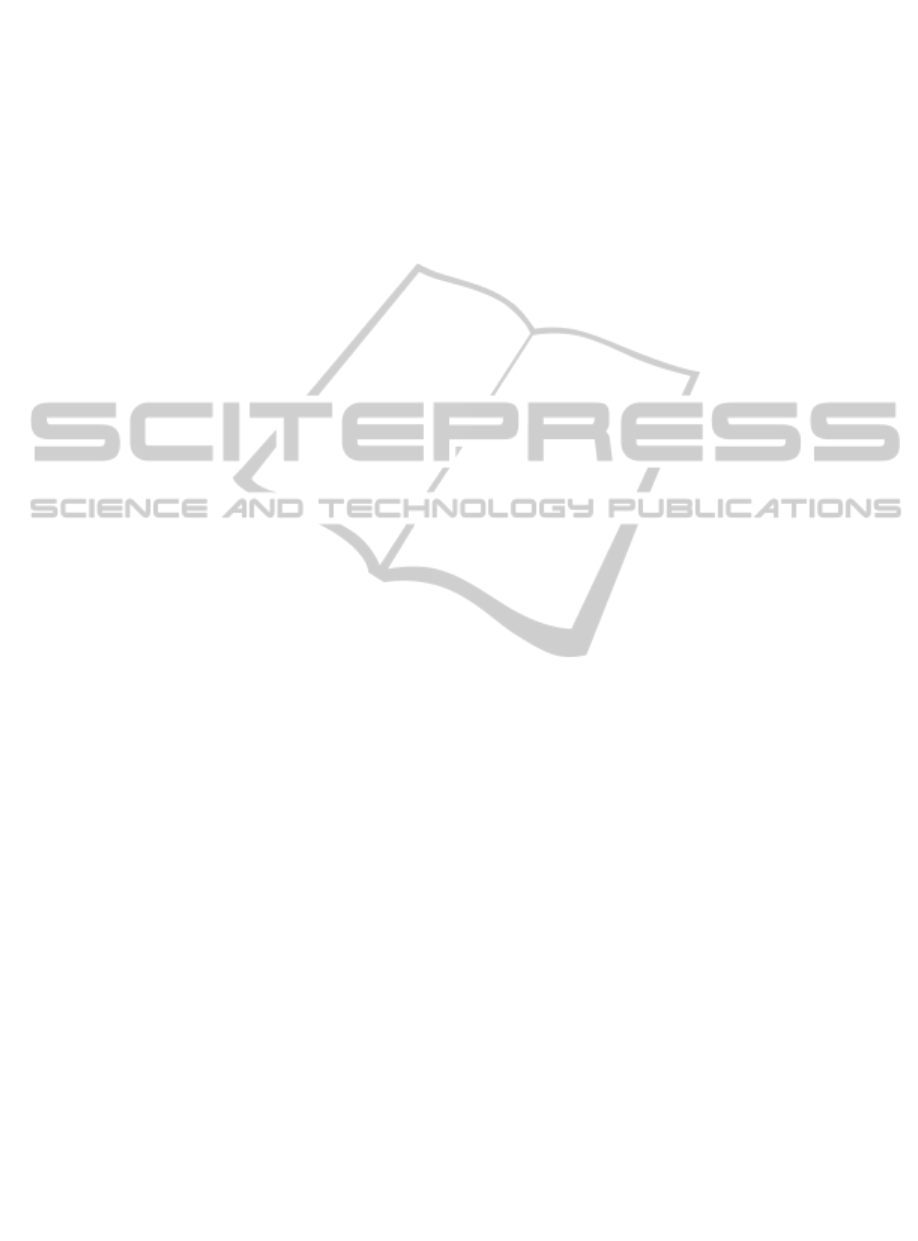
patient healthcare especially in cases where doctor’s
personal intervention is not required and the advice
to patients can be provided over this portal. This will
have a very high impact on the present overall
healthcare system where doctors’ availability is very
scarce in remote rural areas.
ACKNOWLEDGEMENTS
The authors are thankful to SAP Research and SAP
Labs, India for funding this project as a part of the
“1
st
SAP Research & Innovation Award” granted to
PES Institute of Technology.
REFERENCES
Black, H. R., 2002, ’Cardio-Vascular Risk Factors’, Yale
University of Medicine Heart Book, Chapter 3, pp. 32-
36.
Wilson, P. W. F., D’Agostino, R. B., Levy, D., Belanger,
A. M., Silbershatz, H. & Kannel W. B., 1998, ‘MD
Prediction of Coronary Heart Disease Using Risk
Factor Categories’, American Heart Association.
‘Australian Type2 Diabetes Risk Assessment Tool’,
Australian Government, Department of Health and
Ageing, July 2008.
Bhopal, R., Fischbacher, C., Vartiainen, E., Unwin, N.,
White, M & Alberti G, 2005, ’Predicted and observed
cardiovascular disease in South Asians: Application of
FINRISK, Framingham and SCORE models to
Newcastle Heart Project data’, Journal of Public
Health, Vol. 27, No.1, pp. 93-100.
Paynter, N. P., Chasman, D. I., Buring, J. E., Shiffman, D.,
Cook, N. R. & Ridker, P. M., 2009, ‘Cardiovascular
Disease Risk Prediction With and Without Knowledge
of Genetic Variation at Chromosome’ , Annals of
Internal medicine, Vol. 150, No. 2, pp.65-72.
Quirke, T. P., Gill, P. S., Mant, J. W. & Allan, T. F., 2003,
‘The applicability of the Framingham coronary heart
disease prediction functions to black and minority
ethnic groups in the UK’, Department of Primary
Care and General Practice, University of
Birmingham, pp. 785– 786.
D’Agostino, R. B., Vasan, R. S., Pencina, R. J., Wolf, P.
A., Cobain, M., Massaro, J. M. & William B. Kannel,
2008, ‘General Cardiovascular Risk Profile for Use in
Primary Care The Framingham Heart Study’,
American Heart Association, Inc., pp. 743-753.
Mendis, S., Lindholm, L. H., Mancia, G., Whitworth, J.,
Alderman, M., Lim, S. & Heagerty, T., 2007, ‘World
Health Organization (WHO) and International Society
of Hypertension (ISH) risk prediction charts:
assessment of cardiovascular risk for prevention and
control of cardiovascular disease in low and middle-
income countries’, Cardiovascular Diseases, World
Health Organization, Geneva, Switzerland, pp. 1578-
82.
Ridker, P. M., Buring, J. E., Rifai, N & Cook, N. R., 2007,
‘Development and validation of improved algorithms
for the assessment of global cardiovascular risk in
women: the Reynolds Risk Score’, Journal of
American Medical Association, Vol. 297(6).
Pérez-López, F. R., Cuadros-López, J. L., Fernández-
Alonso, A. M., Cuadros-Celorrio, A. M., Sabatel-
López, R. M. & Chedraui, P., 2010, ‘Assessing fatal
cardiovascular disease risk with the SCORE
(Systematic Coronary Risk Evaluation) scale in post-
menopausal women 10 years after different hormone
treatment regimens’, Gynecological Endocrinology,
26(7):533-8.
Cox, J. H., Coupland, C., Vinogradova, Y., Robson, J.,
May, M. & Brindle, P, 2007, ‘Derivation and
validation of QRISK, a new cardiovascular disease
risk score for the United Kingdom: prospective open
cohort study’, British Medical Journal.
Woodward, M., Brindle, P. & Pedoe, H. T., 2007, ‘Adding
social deprivation and family history to cardiovascular
risk assessment: the ASSIGN score from the Scottish
Heart Health Extended Cohort (SHHEC)’, British
Medical Journal, Heart 2007.
Parikh, N. I., Michael, J., Pencina et al, 2008, ‘A Risk
Score for Predicting Near-Term Incidence of
Hypertension: The Framingham Heart Study’, Annals
of Internal Medicine.
Wang, W., Lee, ET; Fabsitz RR et al, 2006, ‘A
Longitudinal Study of Hypertension Risk Factors and
Their Relation to Cardiovascular Disease the Strong
Heart Study’, American Heart Association,
Hypertension.
Yadav, R., Tiwari, P. & Dhanaraj, E., 2008, ‘Risk factors
and complications of type 2 diabetes in Asians’,
CRIPS Vol. 9 No. 2.
‘Lowering Your Blood Pressure With DASH’, U.S.
Department of Health and Human Services, National
Institutes of Health National Heart, Lung, and Blood
Institute, No. 06-4082, April 2006.
HEALTHINF 2011 - International Conference on Health Informatics
542