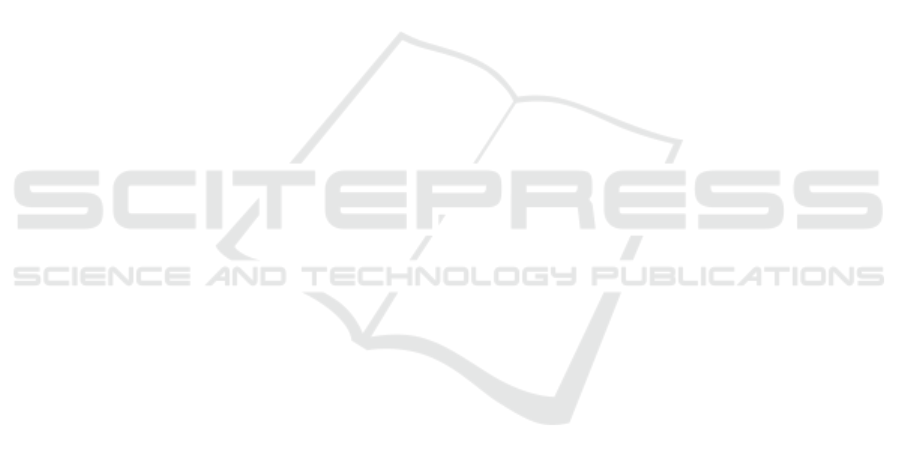
REFERENCES
Adams, R. V. and Blair, E. (2019). Impact of
time management behaviors on undergraduate en-
gineering students’ performance. Sage Open,
9(1):2158244018824506.
Aguilar, S. J., Karabenick, S. A., Teasley, S. D., and Baek,
C. (2021). Associations between learning analytics
dashboard exposure and motivation and self-regulated
learning. Computers & Education, 162:104085.
Alario-Hoyos, C., Est
´
evez Ayres, I. M., San Agustin, M. P.,
Leony Arreaga, D. A., and Delgado Kloos, C. (2015).
Mylearningmentor: A mobile app to support learners
participating in moocs.
Broos, T., Peeters, L., Verbert, K., Soom, C. V., Langie, G.,
and Laet, T. D. (2017). Dashboard for actionable feed-
back on learning skills: Scalability and usefulness. In
International Conference on Learning and Collabora-
tion Technologies, pages 229–241. Springer.
Clarke, V., Braun, V., and Hayfield, N. (2015). Thematic
analysis. Qualitative psychology: A practical guide to
research methods, 222(2015):248.
Clow, D. (2012). The learning analytics cycle: closing the
loop effectively. In Proceedings of the 2nd interna-
tional conference on learning analytics and knowl-
edge, pages 134–138.
Colladon, A. F. and Grippa, F. (2018). The importance
of being honest: Correlating self-report accuracy and
network centrality with academic performance. The
Journal of Psychology, 152(5):304–324.
Garc
´
ıa-Ros, R. and P
´
erez-Gonz
´
alez, F. (2012). Spanish ver-
sion of the time management behavior questionnaire
for university students. The Spanish Journal of Psy-
chology, 15(3):1485–1494.
Garc
´
ıa-Sol
´
orzano, D., Santamar
´
ıa, E., Moran, J. A., Cobo,
G., and Monzo, C. (2018). Personal informatics sys-
tems for supporting self-regulation in online learning
environments. Computer Applications in Engineering
Education, 26(5):1445–1456.
Ga
ˇ
sevi
´
c, D., Dawson, S., Rogers, T., and Gasevic, D.
(2016). Learning analytics should not promote one
size fits all: The effects of instructional conditions in
predicting academic success. The Internet and Higher
Education, 28:68–84.
Hamdan, K. M., Al-Bashaireh, A. M., Zahran, Z., Al-
Daghestani, A., Samira, A.-H., and Shaheen, A. M.
(2021). University students’ interaction, internet self-
efficacy, self-regulation and satisfaction with online
education during pandemic crises of covid-19 (sars-
cov-2). International Journal of Educational Manage-
ment.
Heikkil
¨
a, A. and Lonka, K. (2006). Studying in higher
education: students’ approaches to learning, self-
regulation, and cognitive strategies. Studies in higher
education, 31(1):99–117.
Inan-Karagul, B. and Seker, M. (2021). Improving lan-
guage learners’ use of self-regulated writing strate-
gies through screencast feedback. SAGE Open,
11(4):21582440211064895.
Karaoglan Yilmaz, F. G. and Yilmaz, R. (2020). Stu-
dent opinions about personalized recommendation
and feedback based on learning analytics. Technology,
knowledge and learning, 25(4):753–768.
Kizilcec, R. F., P
´
erez-Sanagust
´
ın, M., and Maldonado,
J. J. (2017). Self-regulated learning strategies predict
learner behavior and goal attainment in massive open
online courses. Computers & education, 104:18–33.
Konert, J., Bohr, C., Bellh
¨
auser, H., and Rensing, C. (2016).
PeerLA - Assistant for Individual Learning Goals and
Self-Regulation Competency Improvement in Online
Learning Scenarios. In 2016 IEEE 16th Int. Conf. Adv.
Learn. Technol., pages 52–56.
Kruger, J. and Dunning, D. (1999). Unskilled and unaware
of it: how difficulties in recognizing one’s own incom-
petence lead to inflated self-assessments. Journal of
personality and social psychology, 77(6):1121.
Matcha, W., Ga
ˇ
sevi
´
c, D., Pardo, A., et al. (2019). A sys-
tematic review of empirical studies on learning ana-
lytics dashboards: A self-regulated learning perspec-
tive. IEEE Transactions on Learning Technologies,
13(2):226–245.
N
´
u
˜
nez, A.-G., Silva, I., Solano-Quinde, L., M., Z.-P.,
Echeverria, V., and De Laet, T. (2022). Learn-
ing analytics to support the provision of feedback in
higher education: a systematic literature review. Latin
American Conference on Learning Technologies (LA-
CLO2022).
Pardo, A., Jovanovic, J., Dawson, S., Ga
ˇ
sevi
´
c, D., and Mir-
riahi, N. (2019). Using learning analytics to scale the
provision of personalised feedback. British Journal of
Educational Technology, 50(1):128–138.
P
´
erez-
´
Alvarez, R., Maldonado-Mahauad, J., and P
´
erez-
Sanagust
´
ın, M. (2018). Tools to support self-regulated
learning in online environments: Literature review. In
European conference on technology enhanced learn-
ing, pages 16–30. Springer.
P
´
erez-
´
Alvarez, R., Maldonado-Mahauad, J. J., Sapunar-
Opazo, D., and P
´
erez-Sanagust
´
ın, M. (2017). Note-
myprogress: A tool to support learners’ self-regulated
learning strategies in mooc environments. In Euro-
pean conference on technology enhanced learning,
pages 460–466. Springer.
Viberg, O., Khalil, M., and Baars, M. (2020). Self-regulated
learning and learning analytics in online learning en-
vironments: A review of empirical research. In
Proceedings of the tenth international conference on
learning analytics & knowledge, pages 524–533.
Wise, A. F. (2014). Designing pedagogical interventions to
support student use of learning analytics. In Proceed-
ings of the fourth international conference on learning
analytics and knowledge, pages 203–211.
Zimmerman, B. J. (2000). Attaining self-regulation: A
social cognitive perspective. In Handbook of self-
regulation, pages 13–39. Elsevier.
Zimmerman, B. J. (2008). Investigating self-regulation and
motivation: Historical background, methodological
developments, and future prospects. American Edu-
cational Research Journal, 45(1):166–183.
CSEDU 2023 - 15th International Conference on Computer Supported Education
224