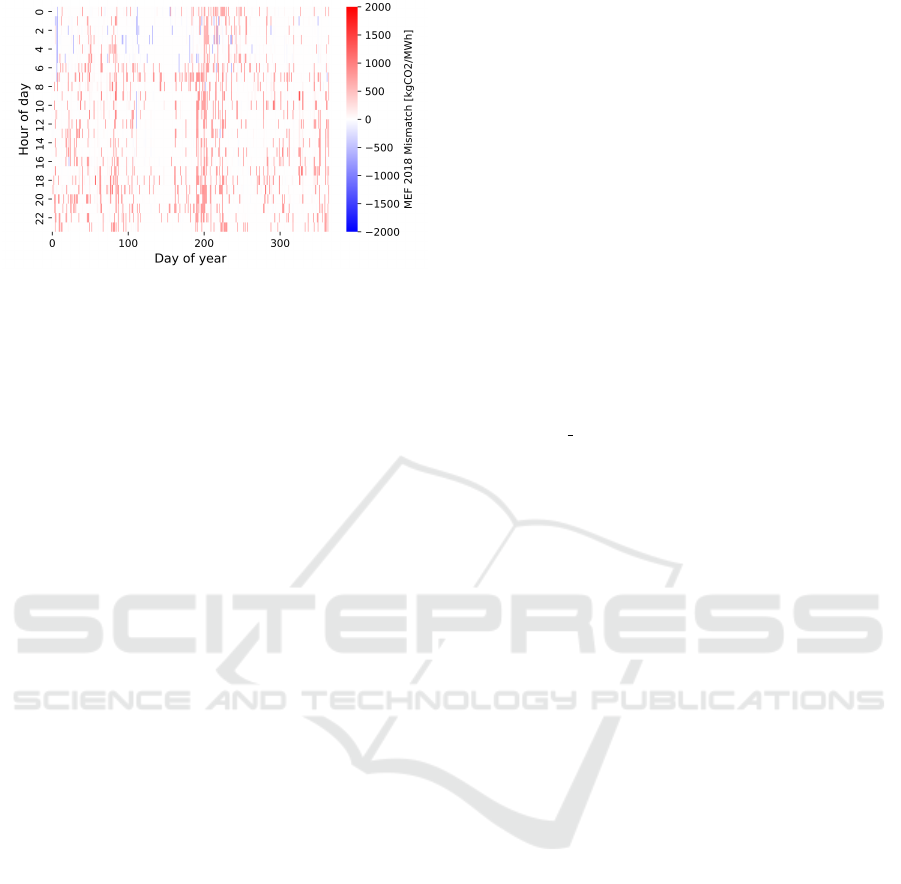
Figure 7: Marginal emission factors differences between
scenarios considering versus ignoring electricity imports.
5 CONCLUSIONS
In this paper, we provide an approach for the deter-
mination of MEF profiles from the electricity sector
and applied the method to the case of the Nether-
lands from 2018 to 2022 via generation facilities merit
order construction. The impact of electricity trade
with other bidding zones is taken into account, lead-
ing to higher accuracy compared to ignoring elec-
tricity trade, which results in underestimating emis-
sion factors. Although the share of electricity gen-
erated from renewable sources has increased substan-
tially over the past few years, electricity prices are still
largely determined by carbon-intensive power plants
at the margin. Variations in MEF profiles and shifts
in marginal power plants are primarily caused by fuel
and CO
2
prices. It is expected that future develop-
ments will increase the share of renewables and in-
crease the variability of marginal generation as a re-
sult. Although the current share of renewable energy
in the electricity mix account for only a small share of
the marginal mix, this is expected to change in the fu-
ture. The consequences of these changes for a future
scenario could be investigated in future work.
In conclusion, the proposed MEF construction ap-
proach is well suited to be used in the emissions opti-
mization of distributed power systems to promote de-
carbonization e.g., scheduling of electricity demand
and evaluating load shifting potentials.
ACKNOWLEDGEMENTS
This study is supported by the Dutch Ministry of
Economic Affairs and Climate Policy and the Dutch
Ministry of the Interior and Kingdom Relations
through the ROBUST project under grant agreement
MOOI32014. This study is also supported by the
Horizon 2020 program and the ARV project under
grant agreement 101036723.
REFERENCES
Biggar, D. R. and Hesamzadeh, M. R. (2014). The eco-
nomics of electricity markets. Wiley Blackwell.
Brinkel, N., Schram, W., AlSkaif, T., Lampropoulos, I.,
and Van Sark, W. (2020). Should we reinforce the
grid? cost and emission optimization of electric ve-
hicle charging under different transformer limits. Ap-
plied Energy, 276:115285.
Brown, M. A. and Chapman, O. (2021). The size, causes,
and equity implications of the demand-response gap.
Energy Policy, 158:112533.
CBS (2022). More electricity from renewable sources,
less from fossil sources. https://www.cbs.nl/en-
gb/news/2022/10/more-electricity-from-renewable-
sources less-from-fossil-sources, Accessed: Decem-
ber 9, 2022.
De Vita, A., Kielichowska, I., Mandatowa, P., Capros,
P., Dimopoulou, E., Evangelopoulou, S., Fotiou, T.,
Kannavou, M., Siskos, P., Zazias, G., et al. (2018).
Technology pathways in decarbonisation scenarios.
Tractebel, Ecofys, E3-Modelling: Brussels, Belgium.
ENTSO-E (2021). Transparency platform; day-ahead
prices. https://transparency.entsoe.eu/transmission-
domain/r2/dayAheadPrices/show, Accessed: January
13, 2023.
German Federal Statistical Office (2022). Preise -
erdgas- und stromdurchschnittspreise. https://www.
destatis.de/, Accessed: January 9, 2023.
Gissey, G. C., Guo, B., Newbery, D., Lipman, G., Mon-
toya, L., Dodds, P., Grubb, M., and Ekins, P. (2019).
The value of international electricity trading. A project
commissioned by Ofgem.
Hawkes, A. (2010). Estimating marginal co2 emissions
rates for national electricity systems. Energy Policy,
38(10):5977–5987.
Hoehne, C. G. and Chester, M. V. (2016). Optimizing plug-
in electric vehicle and vehicle-to-grid charge schedul-
ing to minimize carbon emissions. Energy, 115:646–
657.
Holland, S. P., Kotchen, M. J., Mansur, E. T., and Yates,
A. J. (2022). Why marginal co2 emissions are not
decreasing for us electricity: Estimates and implica-
tions for climate policy. Proceedings of the National
Academy of Sciences, 119(8):e2116632119.
Holland, S. P., Muller, N. Z., Mansur, E. T., and Yates, A. J.
(2015). Environmental benefits from driving electric
vehicles? National Bureau of Economic Research:
Cambridge MA.
Investing (2022). Stock market quotes and financial news.
https://www.investing.com/, Accessed: October 5,
2022.
JRC (2019). European commission open power plants
database(jrc-ppdb-open). http://data.europa.eu/89h/
SMARTGREENS 2023 - 12th International Conference on Smart Cities and Green ICT Systems
56