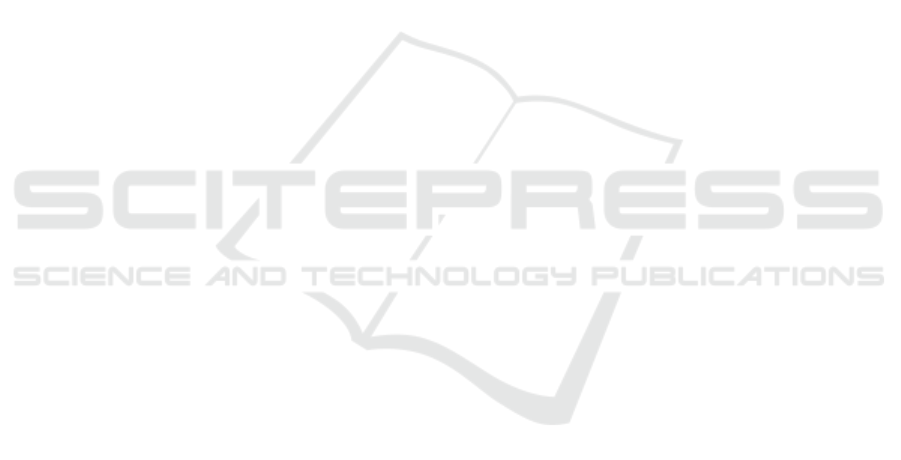
sults section, the maximum flow of 100% CAVs pene-
tration rate increased by approximately 13.55% com-
pared with the all HDVs scenario. Moreover, the im-
provements in critical density for different scenarios
have a similar trend with the maximum flow improve-
ments. In terms of congestion level, even a low CAV
penetration rate may greatly improve the traffic con-
dition. Table 4 shows that 20% of CAVs penetration
rate eliminates the congestion in the original demand
and 110% demand scenarios. In the 120% demand
scenario, 20% CAVs greatly reduce traffic conges-
tion level. Last but not least, when the CAV pene-
tration rate rises from 0% to 100%, the increase in
the proportional demand the network can carry under
the same congestion degree is much more significant
than the increase in the maximum flow. In this work,
the increase in the proportional demand is more than
two times the increase in maximum flow. This is due
to the introduction of CAVs reducing the congestion
level and making the congestion dissipate faster. At
the same time, a daily demand rather than a constant
demand enlarges the advantage.
However, there is a limitation in this study. The
traffic flow is heterogeneously distributed, which
means the transportation system still has untapped po-
tential. The capacity of the traffic system will be more
fully utilized if a control algorithm is used to control
the distribution of traffic as homogeneously as possi-
ble, which we leave as future work.
ACKNOWLEDGEMENTS
The research reported in this paper is part of the
project Prin 2020 DigiT-CCAM-Digital Twins per
la Mobilit
`
a Cooperativa, Connessa e Automatizzata
(project no. 2020Z9HEMJ) funded by the Italian
Ministry of the University and the Research.
REFERENCES
Amb
¨
uhl, L., Loder, A., Bliemer, M. C., Menendez, M., and
Axhausen, K. W. (2020). A functional form with a
physical meaning for the macroscopic fundamental di-
agram. Transportation Research Part B: Methodolog-
ical, 137:119–132.
Chen, D., Ahn, S., Chitturi, M., and Noyce, D. A. (2017).
Towards vehicle automation: Roadway capacity for-
mulation for traffic mixed with regular and automated
vehicles. Transportation research part B: method-
ological, 100:196–221.
Daganzo, C. F. (2007). Urban gridlock: Macroscopic mod-
eling and mitigation approaches. Transportation Re-
search Part B: Methodological, 41(1):49–62.
Geroliminis, N. and Daganzo, C. F. (2008). Existence
of urban-scale macroscopic fundamental diagrams:
Some experimental findings. Transportation Research
Part B: Methodological, 42(9):759–770.
Geroliminis, N. and Sun, J. (2011). Properties of a well-
defined macroscopic fundamental diagram for urban
traffic. Transportation Research Part B: Methodolog-
ical, 45(3):605–617.
Ghiasi, A., Hussain, O., Qian, Z. S., and Li, X. (2017).
A mixed traffic capacity analysis and lane manage-
ment model for connected automated vehicles: A
markov chain method. Transportation Research Part
B: Methodological, 106:266–292.
Godfrey, J. (1969). The mechanism of a road network. Traf-
fic Engineering & Control, 8(8).
Goodall, N. J., Smith, B. L., and Park, B. (2013). Traffic
signal control with connected vehicles. Transporta-
tion Research Record, 2381(1):65–72.
Gu
´
eriau, M. and Dusparic, I. (2020). Quantifying the im-
pact of connected and autonomous vehicles on traffic
efficiency and safety in mixed traffic. In 2020 IEEE
23rd International Conference on Intelligent Trans-
portation Systems (ITSC), pages 1–8. IEEE.
Herman, R. and Prigogine, I. (1979). A two-fluid approach
to town traffic. Science, 204(4389):148–151.
Hu, G., Lu, W., Whalin, R. W., Wang, F., and Kwembe,
T. A. (2021). Analytical approximation for macro-
scopic fundamental diagram of urban corridor with
mixed human and connected and autonomous traffic.
IET Intelligent Transport Systems, 15(2):261–272.
Liu, H., Kan, X., Shladover, S. E., Lu, X.-Y., and Ferlis,
R. E. (2018). Impact of cooperative adaptive cruise
control on multilane freeway merge capacity. Journal
of Intelligent Transportation Systems, 22(3):263–275.
Loder, A., Amb
¨
uhl, L., Menendez, M., and Axhausen,
K. W. (2019). Understanding traffic capacity of urban
networks. Scientific reports, 9(1):1–10.
Lopez, P. A., Behrisch, M., Bieker-Walz, L., Erdmann, J.,
Fl
¨
otter
¨
od, Y.-P., Hilbrich, R., L
¨
ucken, L., Rummel,
J., Wagner, P., and Wießner, E. (2018). Microscopic
traffic simulation using SUMO. In 2018 21st inter-
national conference on intelligent transportation sys-
tems (ITSC), pages 2575–2582. IEEE.
Lu, Q., Tettamanti, T., H
¨
orcher, D., and Varga, I. (2020).
The impact of autonomous vehicles on urban traffic
network capacity: an experimental analysis by mi-
croscopic traffic simulation. Transportation Letters,
12(8):540–549.
L
¨
ucken, L., Mintsis, E., Kallirroi, N. P., Alms, R., Fl
¨
otter
¨
od,
Y.-P., and Koutras, D. (2019). From automated to
manual - modeling control transitions with SUMO.
In Weber, M., Bieker-Walz, L., Hilbrich, R., and
Behrisch, M., editors, SUMO User Conference 2019,
volume 62 of EPiC Series in Computing, pages 124–
144. EasyChair.
Mahmud, S. S., Ferreira, L., Hoque, M. S., and Tavassoli,
A. (2017). Application of proximal surrogate indica-
tors for safety evaluation: A review of recent develop-
ments and research needs. IATSS research, 41(4):153–
163.
VEHITS 2023 - 9th International Conference on Vehicle Technology and Intelligent Transport Systems
384