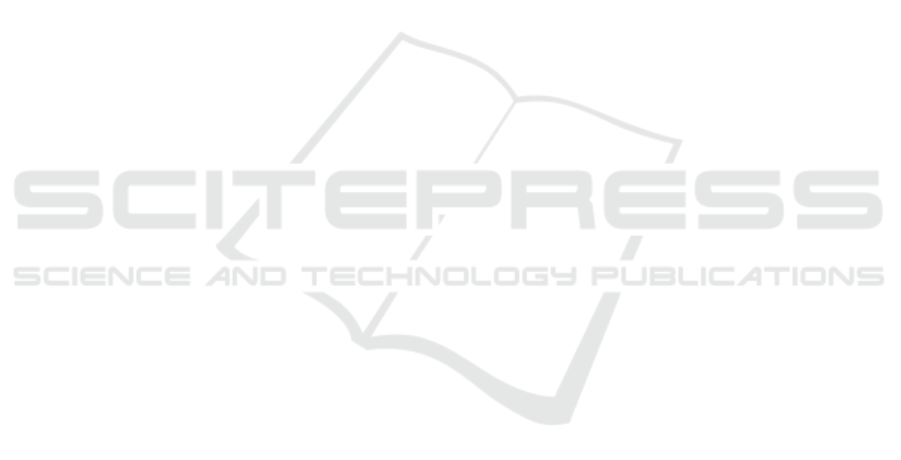
Brown, J. D. and Wahlers, R. G. (1998). The environ-
mentally concerned consumer: An exploratory study.
Journal of Marketing Theory and Practice, 6(2):39–
47.
Campbell-Johnston, K., Vermeulen, W. J., Reike, D., and
Brullot, S. (2020). The circular economy and cascad-
ing: towards a framework. Resources, Conservation
& Recycling: X, 7:100038.
Cuerva, M. C., Triguero-Cano,
´
A., and C
´
orcoles, D. (2014).
Drivers of green and non-green innovation: empirical
evidence in low-tech smes. Journal of Cleaner Pro-
duction, 68:104–113.
de Lima, F. A., Seuring, S., and Sauer, P. C. (2022). A sys-
tematic literature review exploring uncertainty man-
agement and sustainability outcomes in circular sup-
ply chains. International Journal of Production Re-
search, 60(19):6013–6046.
Elghaish, F., Matarneh, S. T., Edwards, D. J., Rahimian,
F. P., El-Gohary, H., and Ejohwomu, O. (2022).
Applications of industry 4.0 digital technologies to-
wards a construction circular economy: gap analysis
and conceptual framework. Construction Innovation,
2022(ahead-of-print).
Elghaish, F., Matarneh, S. T., Talebi, S., Abu-Samra, S.,
Salimi, G., and Rausch, C. (2021). Deep learning for
detecting distresses in buildings and pavements: a crit-
ical gap analysis. Construction Innovation.
EMF (2017). The new plastics economy: Rethinking the
future of plastics & catalysing action. https://tinyurl.
com/3rduzf6x. Accessed August 21, 2019.
Forti, V., Balde, C. P., Kuehr, R., and Bel, G. (2020). The
global e-waste monitor 2020: Quantities, flows, and
the circular economy potential.
Gharfalkar, M., Ali, Z., and Hillier, G. (2016). Clarifying
the disagreements on various reuse options: Repair,
recondition, refurbish and remanufacture. Waste Man-
agement & Research, 34(10):995–1005.
Hoornweg, D. and Bhada-Tata, P. (2012). What a waste: a
global review of solid waste management.
Korley, L. T., Epps III, T. H., Helms, B. A., and Ryan, A. J.
(2021). Toward polymer upcycling—adding value and
tackling circularity. Science, 373(6550):66–69.
Kumar, V., Sezersan, I., Garza-Reyes, J. A., Gonzalez,
E. D., and Moh’d Anwer, A.-S. (2019). Circular econ-
omy in the manufacturing sector: benefits, opportuni-
ties and barriers. Management Decision.
Lopes de Sousa Jabbour, A. B., Jabbour, C. J. C., God-
inho Filho, M., and Roubaud, D. (2018). Industry 4.0
and the circular economy: a proposed research agenda
and original roadmap for sustainable operations. An-
nals of Operations Research, 270(1):273–286.
Machnik, A. and Kr
´
olikowska-Tomczak, A. (2022). Aware-
ness rising of consumers, employees, suppliers, and
governments. In Responsible Consumption and Pro-
duction, pages 22–36. Springer.
Mboli, J. S., Thakker, D., and Mishra, J. L. (2022). An
internet of things-enabled decision support system for
circular economy business model. Software: Practice
and Experience, 52(3):772–787.
Mboli, J. S., Thakker, D., Mishra, J. L., and Sivarajah, S.
(2021). Domain experts and natural language pro-
cessing in the evaluation of circular economy busi-
ness model ontology. In 2021 IEEE 15th Interna-
tional Conference on Semantic Computing (ICSC),
pages 374–379. IEEE.
Menezes, B. C., Kelly, J. D., Leal, A. G., and Le Roux,
G. C. (2019). Predictive, prescriptive and detective
analytics for smart manufacturing in the information
age. IFAC-PapersOnLine, 52(1):568–573.
Mishra, J. L., Hopkinson, P. G., and Tidridge, G. (2018).
Value creation from circular economy-led closed loop
supply chains: a case study of fast-moving consumer
goods. Production Planning & Control, 29(6):509–
521.
Motovilov, A. and Lutchenko, S. (2022). Calculation of
mean time to failure of infocommunication system
wich failure rate is cycle changed. In 2022 Dynamics
of Systems, Mechanisms and Machines (Dynamics),
pages 1–4. IEEE.
Nobre, G. C. and Tavares, E. (2017). Scientific literature
analysis on big data and internet of things applications
on circular economy: a bibliometric study. Sciento-
metrics, 111(1):463–492.
Ramadoss, T. S., Alam, H., and Seeram, R. (2018). Artifi-
cial intelligence and internet of things enabled circular
economy. The International Journal of Engineering
and Science, 7(9):55–63.
ResCoM (2017). Rescom shared terminology. https://
tinyurl.com/2hzmcvx3. Accessed April 21, 2019.
The E-waste Coalition, W. (2019). A new circular vision
for electronics: Time for a global reboot. World Eco-
nomic Forum.
Uc¸ar, E., Le Dain, M.-A., and Joly, I. (2020). Digital
technologies in circular economy transition: evidence
from case studies. Procedia cirp, 90:133–136.
Wang, L.-X. and Mendel, J. M. (1992). Generating fuzzy
rules by learning from examples. IEEE Transactions
on systems, man, and cybernetics, 22(6):1414–1427.
WEF (2014). Towards the circular economy: Accelerat-
ing the scale-up across global supply chains. https:
//tinyurl.com/nhjsfxva. Accessed May 10, 2019.
ICEIS 2023 - 25th International Conference on Enterprise Information Systems
666