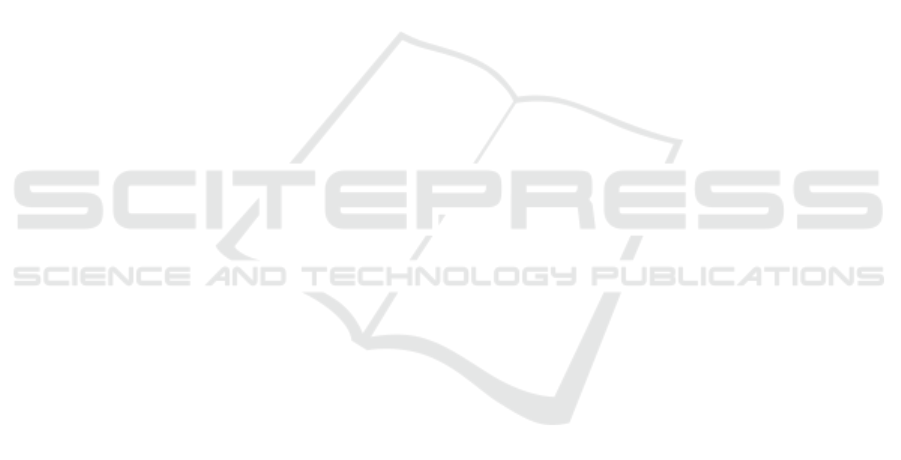
iContracts. Our following research will focus on val-
idating the integration experimentally.
The research novelty is that we have demonstrated
how it is possible to make explicit a traditionally im-
plicit process, in such a way that data processing be-
comes possible. So far, legal experts have not reached
consensus on how to manage contract risk. Our pa-
per shows how it is possible. Moreover, in relation to
the bow-tie method, we presented a new theoretical
version for it which builds upon the old one, disam-
biguate some of the bow-tie constructs, and enrich its
conceptualisation.
ACKNOWLEDGEMENTS
Georgios is the main author. Giulia provided a sci-
entific framework for the research. Athanasios con-
tributed in the literature. Jaap and Bart are the main
supervisors.
REFERENCES
Agrawal, V. (2016). Towards the ontology of iso/iec 27005:
2011 risk management standard. In HAISA, pages
101–111.
Becker, B. J. and Alow, A. M. (2019). Model-based Meta-
Analysis and Related Approaches, pages 339–364.
Russell Sage Foundation.
Dauer, E. A. (2006). The role of culture in legal risk man-
agement. Scandinavian Studies in Law, 49:49–6.
de Ruijter, A. and Guldenmund, F. (2016). The bowtie
method: A review. Safety Science, 88:211–218.
de Ruijter, A. and Guldenmund, F. (2016). The bowtie
method: A review. Safety science, 88:211–218.
Dud
´
a
ˇ
s, M., Lohmann, S., Sv
´
atek, V., and Pavlov, D. (2018).
Ontology visualization methods and tools: a survey
of the state of the art. The Knowledge Engineering
Review, 33.
Espenschied, L. E. (2010). Contract drafting: Powerful
prose in transactional practice. American Bar Asso-
ciation.
Fahad, M. and Qadir, M. A. (2008). A framework for on-
tology evaluation. pages 149–158.
Fahad, M., Qadir, M. A., and Shah, S. A. H. (2008). Eval-
uation of ontologies and dl reasoners. In Shi, Z.,
Mercier-Laurent, E., and Leake, D., editors, Intelli-
gent Information Processing IV, pages 17–27, Boston,
MA. Springer US.
for Chemical Process Safety, C. (2018). Bow Ties in Risk
Management: A Concept Book for Process Safety.
Wiley-AIChE.
Fox, C. M. (2008). Working with contracts. What Law
doesnt teach you. Second Edition. New York: Practis-
ing Law Institute.
Gomez-Perez, A. (1995). Some ideas and examples to eval-
uate ontologies. pages 299–305.
G
´
omez-P
´
erez, A., Fern
´
andez-L
´
opez, M., and Corcho,
´
O.
(2004). Ontological engineering: With examples from
the areas of knowledge management, e-commerce and
the semantic web. In Advanced Information and
Knowledge Processing.
G
´
omez-P
´
erez, A. (2001). Evaluation of ontologies. Interna-
tional Journal of Intelligent Systems, 16(3):391–409.
Haapio, H. and Siedel, G. J. (2013). A short guide to con-
tract risk.
Hogan, A. (2020). Linked Data, pages 515–625. Springer
International Publishing, Cham.
Khakzad, N., Khan, F., and Amyotte, P. (2012). Dynamic
risk analysis using bow-tie approach. Reliability En-
gineering & System Safety, 104:36–44.
Kishchuk, B., Creed, I., Laurent, K., Nebel, S.,
Kreutzweiser, D., Venier, L., and Webster, K. (2018).
Assessing the ecological sustainability of a forest
management system using the iso bowtie risk man-
agement assessment tool. The Forestry Chronicle,
94(1):25–34.
Kluwer, W. (2017). The history of bowtie. http://www.
cgerisk.com.
Kluwer, W. (2019). The bowtie method. http://www.
cgerisk.com.
Kuwahara, S., Yoshino, N., Sagara, M., and
Taghizadeh Hesary, F. (2015). Role of the credit risk
database in developing smes in japan: Lessons for the
rest of asia.
Leva, M. C., Balfe, N., McAleer, B., and Rocke, M. (2017).
Risk registers: Structuring data collection to develop
risk intelligence. Safety science, 100:143–156.
Lu, L., Liang, W., Zhang, L., Zhang, H., Lu, Z., and Shan, J.
(2015). A comprehensive risk evaluation method for
natural gas pipelines by combining a risk matrix with
a bow-tie model. Journal of Natural Gas Science and
Engineering, 25:124–133.
Mason, J. (2017). Intelligent contracts and the construction
industry. Journal of Legal Affairs and Dispute Resolu-
tion in Engineering and Construction, 9(3):04517012.
Nirenburg, S. and Raskin, V. (2001). Ontological seman-
tics, formal ontology, and ambiguity. In Proceedings
of the International Conference on Formal Ontology
in Information Systems - Volume 2001, FOIS ’01, page
151–161, New York, NY, USA. Association for Com-
puting Machinery.
Patterson, F. D. and Neailey, K. (2002). A risk regis-
ter database system to aid the management of project
risk. International Journal of Project Management,
20(5):365–374.
Stathis, G., Trantas, A., Biagioni, G., van den Herik,
J., Custers, B., Daniele, L., and Katsigiannis, T.
(2023). Towards a foundation for intelligent con-
tracts. In Proceedings of the 15th International Con-
ference on Agents and Artificial Intelligence - Volume
2: ICAART,, pages 87–98. INSTICC, SciTePress.
DATA 2023 - 12th International Conference on Data Science, Technology and Applications
234