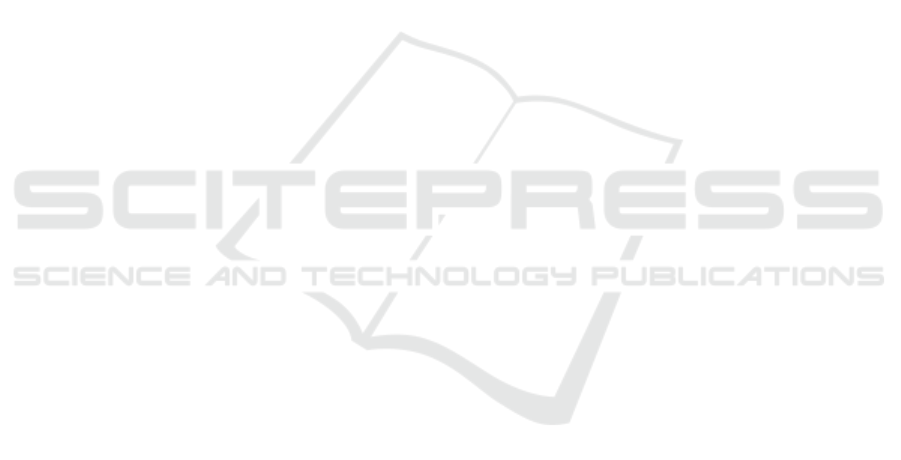
– Stage 1A: the tumor is smaller than 0.2 mm and
has not spread to the lymph nodes.
– Stage 1B: Cancer between 0.2 mm and 2 mm is
found in the lymph nodes.
• Stage 2: compared to stage 1, the tumor is larger
and might have migrated to a few nearby lymph
nodes. In particular:
– Stage 2A: Though it can’t be detected, the tu-
mor has spread from one to three lymph nodes
(but has not spread to other parts of the body).
The tumor can be 20 mm or smaller (less than
20 mm it cannot be identified) and can spread
to one to three lymph nodes, or the tumor is be-
tween 20 mm and 50 mm and has not spread to
lymph nodes.
– Stage 2B: The tumor is between 20 mm and 50
mm and has spread to one to three lymph nodes,
or the tumor is larger than 50 mm but has not
spread to any lymph nodes.
• Stage 3: the tumor is larger than at stage 2 and/or
has spread to several lymph nodes and/or to tissue
around the breast or breast bone. In particular:
– Stage 3A: The tumor has spread from four to
nine lymph nodes or to mammary lymph nodes,
but not to other parts of the body; or the tumor
is larger than 50 mm and spread to one to three
lymph nodes.
– Stage 3B: The tumor has spread to the chest
area or caused the breast to swell, or it is in-
flammatory breast cancer. It may have spread
to up to nine lymph nodes but has not spread to
other parts of the body.
– Stage 3C: This refers to any tumor that has
spread to 10 or more lymph nodes, including
those under the collarbone, but has not spread
to other parts of the body.
• Stage 4: the cancer has metastasized, or mobi-
lized, and spread to distant parts of the body, typ-
ically bones, lungs or liver. This is an advanced
stage of cancer, called metastatic breast cancer.
It is possible to have recurrence breast cancer within
the first two or three years after treatment, but, in
some cases, it may recur also many years later, (City
of Hope, 2023).
Screening is essential since early diagnosis can
change the course of the disease by avoiding reach-
ing the metastatic form; without any form of preven-
tion, there is a risk of tumor growth and of its spread
through metastases, affecting the success of the ther-
apy and decreasing the probability of survival. Di-
agnostic tests (e.g. mammography, ultrasonography,
magnetic resonance imaging, breast self-examination,
as well as modern and more precise imaging methods)
help the early detection of tumors or lesions predis-
posing to tumors, (Kolak et al., 2017).
The problem of the containment of breast cancer
has been faced also studying the patient’s molecular
profile, to predict the drug response, as in (Huang
et al., 2021). A compartmental modeling approach
is proposed in (Tang et al., 2022), where adverse re-
action on the patient heart (cardiotoxicity) is studied
in the framework of fractional calculus.
The aim of this paper is to carry out an epidemio-
logical study of the spread of breast cancer, proposing
a compartmental model able to represent, in a simpli-
fied way, the population partitioned with respect to
the individuals’ conditions regarding the breast can-
cer. Male breast cancer is rare and accounts for about
1% of cancers occurring in men and about 1% of all
breast cancers worldwide, so the target population is
the female population, (Fox et al., 2022). In particu-
lar, five compartments are introduced:
• Healthy population.
• Population at stage 0, 1 and 2.
• Population at stage 3 and 4.
• Population of dead subjects from causes different
from breast cancer.
• Population of dead individuals because of breast
cancer.
The population division is inspired by the arti-
cle (Van der Broek et al., 2018), whose model sim-
ulates individual life histories from birth to death,
with and without breast cancer, in the presence and
in the absence of screening and treatment, facing the
problem of risk based screening and treatment by us-
ing MISCAN-Faia microsimulation model. The MIS-
CAN is a computer program introduced in (Habbema
et al., 2018) based on Monte Carlo simulation, yield-
ing the effect of screening on morbidity and mortality
on the population.
In this paper, after the introduction of a dynamical
model describing the evolution of the population in
the five stages, the identification of model parameters
is obtained by using real data of the female popula-
tion of Lazio (region of Italy). Then, three contain-
ment measures are considered, corresponding to non
invasive prevention, like echografy and mammogra-
phy screening, first level actions, corresponding to
chemotherapy, and second level actions, correspond-
ing to surgery. In the framework of optimal control
theory it is possible to propose the best resource al-
location strategy aiming at reducing the number of
population at stage 3 and 4 as well as the number of
individuals dead for breast cancer.
ICINCO 2023 - 20th International Conference on Informatics in Control, Automation and Robotics
196