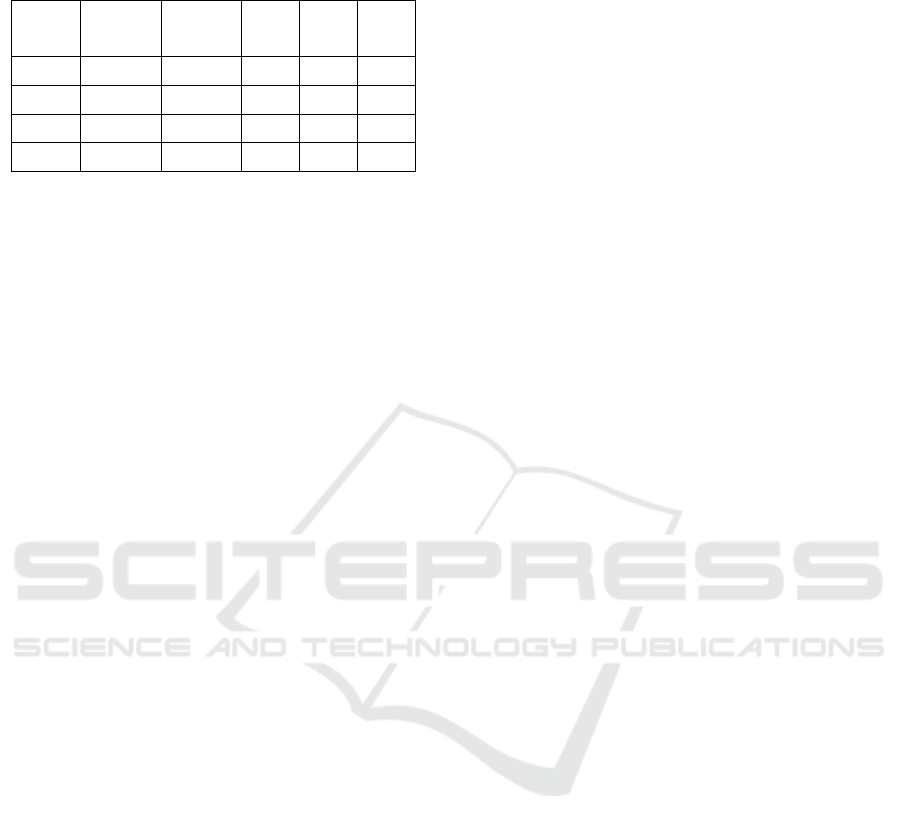
Table 3: Generalized likelihood ratio test of hypothesis for
the stochastic frontier production model.
Null L-
likelihood
L-
likelihood
LR
test
Critical Decision
Hypothesis Function
(H
0
)
Function
(H
1
)
Statistic Value
H
0
: γ =
0
87.1588 120.3780 66.4383 2.706* Reject
H
0
:
β
kl
= 0
70.7475 125.4358 109.3765 18.307 Reject
H
0
: µ =
0
125.3437 125.4358 0.1842 3.841 Accept
H
0
: η =
0
120.3780 125.3437 9.9314 3.841 Accept
tic exceeds the critical value. This result confirms that
technical inefficiencies exist and are significant in ex-
plaining the performance in the production of crude
palm oil by the states. The second null hypothesis
H0 : βkl = 0 which specifies that the Cobb-Douglas
production function is statistically more preferable
than the translog production function is rejected. This
indicates that the usage of translog production func-
tion is more appropriate for the data set. The third null
hypothesis H
0
: µ = 0 is accepted since the test statistic
value did not exceed the critical value. We can con-
clude that the most suitable distribution for the ineffi-
ciency is the half-normal distribution. Finally, the null
hypothesis H
0
: η = 0 implies that the technical inef-
ficiencies are time invariant. This is rejected showing
that time does significantly influence the technical in-
efficiencies in the production model. From the results
of these hypothesis tests, we can conclude that the
most preferable form of the production function for
the data set is the translog stochastic frontier produc-
tion function with the inefficiency assumed to follow
a half-normal distribution and are time-variant.
4 CONCLUSION
This study set out to estimate the technical efficiency
(TE) of producing crude palm oil (CPO) in Malaysia
by applying the parametric stochastic frontier analy-
sis (SFA) technique. The overall mean TE is 0.79.
We found that fruit mill capacity, labour and time as
input variables significantly affect the level of CPO
output. Labour and time variables have positive rela-
tionship with the output level. On the other hand, fruit
mill capacity was shown to have a negative relation-
ship with the CPO production which could possibly
indicate that the mills are not utilized to their full ca-
pacity. Plantation area was proven to be statistically
insignificant in affecting output level. 71.6% of the
variation in deviations were due to technical ineffi-
ciencies whereas 28.4% were cause by the stochastic
random error. SFA estimated the state of Selangor to
be the most efficient CPO producing state among our
population and the state of Malacca to be the least
efficient. Even though the average efficiency of the
Malaysian CPO industry seems to be increasing grad-
ually each year, there is still room for improvement.
Inefficiencies could be reduced by managing existing
resources better, utilization of idle capacity, operat-
ing at optimal scale and applying the ways of efficient
states. The status of fruit mills in Malaysia needs to
be looked at as it was discovered to have a negative re-
lationship with output level. The existing mills possi-
bly are not fully utilized. Future study should be done
on the productivity of CPO production based on the
size of fruit mills and whether smaller fruit mills are
easier to manage and monitor. The productivity of the
whole industry decreases each year due to technologi-
cal change. Thus, investing in new technology is what
needs to be done to encourage productivity growth in
the industry. It is recommended that further study be
done on identifying the factors influencing the TE of
producing CPO in Malaysia preferably using the SFA
(Coelli, 1995) model specification. The inclusion of
environmental variables is highly suggested such as
rainfall and temperature.
REFERENCES
Basiron, Y. (2007). Palm oil production through sustainable
plantations. European Journal of Lipid Science and
Technology, 109(4):289–295.
Baten, M., Kamil, A., and Mohammad, A. (2009). Model-
ing technical inefficiencies effects in a stochastic fron-
tier production function for panel data. African Jour-
nal of Agricultural Research, 4(12):1374–1382.
Battese, G. and Coelli, T. (1992). Frontier production func-
tions, technical efficiency and panel data: With appli-
cation to paddy farmers in india. Journal of Produc-
tivity Analysis, 3(1-2):153–169.
Battese, G. and Corra, G. (1977). Estimation of a produc-
tion frontier model: with application to the pastoral
zone of eastern australia. Australian Journal of Agri-
cultural Economics, 21(3):169–179.
Bogetoft, P., Otto, L., and Boles, J. (2011). Benchmark-
ing with dea, sfa and r. In Proceedings of the Annual
Meeting (Western Farm Economics Association, New
York. Springer.
Coelli, T. (1995). Estimators and hypothesis tests for a
stochastic frontier function: a monte carlo analysis.
Journal of Productivity Analysis, 6(3):247–268.
Coelli, T. and Battese, G. (1996a). Identification of fac-
tors which influence the technical inefficiency of in-
dian farmers. Australian Journal of Agricultural and
Resource Economics, 40(2):103–128.
Coelli, T. and Battese, G. (1996b). Identification of fac-
tors which influence the technical inefficiency of in-
dian farmers. Australian Journal of Agricultural Eco-
nomics, 40(2):103–128.
ICAISD 2023 - International Conference on Advanced Information Scientific Development
202