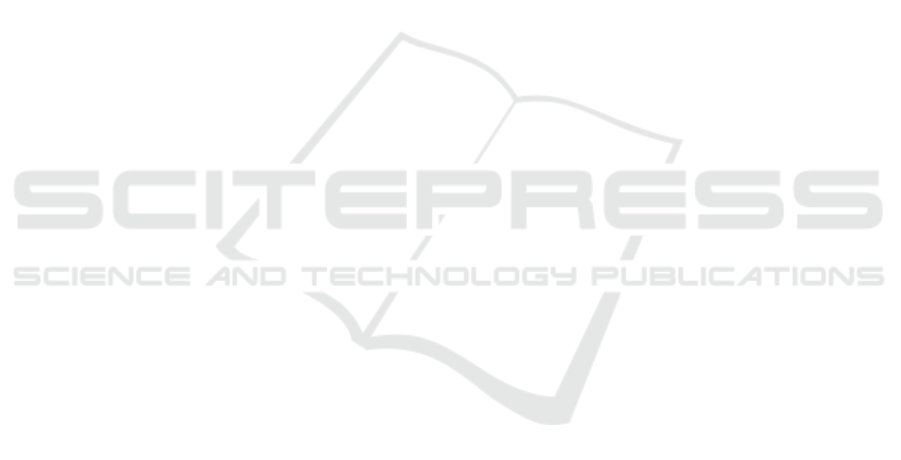
IGL. (2022). Material Safety Data Sheet Natural Gas. IGL.
https://www.iglonline.net/uploads/files/Material_Safet
y_Data_Sheet-_Natural_Gas.pdf
Jones, D., Snider, C., Nassehi, A., Yon, J., & Hicks, B.
(2020). Characterising the Digital Twin: A systematic
literature review. CIRP Journal of Manufacturing
Science and Technology, 29, 36–52.
https://doi.org/10.1016/j.cirpj.2020.02.002
Li, X., Zhang, Y., Li, C., Wang, T., & Xi, C. (2022).
Application of Digital Twin in Handling and
Transportation of Hazardous Chemicals. Applied
Sciences (Switzerland), 12(24).
https://doi.org/10.3390/app122412746
Mckinsey and Company. (2023). What is digital-twin
technology ? (Issue July).
Melesse, T. Y., Di Pasquale, V., & Riemma, S. (2020).
Digital twin models in industrial operations: A
systematic literature review. Procedia Manufacturing,
42(2019), 267–272.
https://doi.org/10.1016/j.promfg.2020.02.084
MIT Technology Review Insights;, & Shell Inc; (2022).
Digital technology : The backbone of a net-zero
emissions future. In MIT Technology Review Insights.
https://www.shell.com/energy-and-
innovation/digitalisation/digitalisation-accelerating-
the-energy-transition/digitalisation-and-
decarbonisation-mit-
report/_jcr_content/root/main/section/call_to_action/li
nks/item0.stream/1678207879539/34a35ef0e6176ff3e
b255
Mohammadpoor, M., & Torabi, F. (2020). Big Data
analytics in oil and gas industry: An emerging trend.
Petroleum, 6(4), 321–328.
https://doi.org/10.1016/j.petlm.2018.11.001
Negri, E., Fumagalli, L., & Macchi, M. (2017). A Review
of the Roles of Digital Twin in CPS-based Production
Systems. Procedia Manufacturing, 11(June), 939–948.
https://doi.org/10.1016/j.promfg.2017.07.198
Opoku, D. G. J., Perera, S., Osei-Kyei, R., & Rashidi, M.
(2021). Digital twin application in the construction
industry: A literature review. Journal of Building
Engineering, 40(April), 102726.
https://doi.org/10.1016/j.jobe.2021.102726
PNGRB. (2008). PNGRB (Technical Standards and
Specifications including Safety Standards for City or
Local Natural Gas Distribution Networks) Regulations,
2008. PNGRB.
PNGRB. (2018). PNGRB CGD in India. In PNGRB.
PNGRB. (2023). PNGRB 12th CGD Bidding Round.
PNGRB, 93.
https://www.pngrb.gov.in/OurRegulation/pdf/Gazette-
Regulation/English/GSR808(E)-E.pdf
PPAC. (2023). PPAC Archive. PPAC, MoPNG.
https://ppac.gov.in/archives
Prasad, A. (2011). Risks and challenges in the speedy
execution of pipeline projects. Premium Pipeline
International Digest, October, 5–9.
Prasad, A., & Kumar, A. (2022). Supply Chain
Performance Measurement Methodology for COVID-
19 Disruption Recovery- Natural Gas Retailing in
India. Pacific Business Review International, 15(1),
41–55.
Prasad, A., Kumar, A., & Chatnani, N. N. (2023). Carbon
Neutrality with Sustainable Supply Chain Project
Management Framework for Affordable Access to
Natural Gas in India. Pacific Business Review
International, 15(12), 1–17.
Pregnolato, M., Gunner, S., Voyagaki, E., De Risi, R.,
Carhart, N., Gavriel, G., Tully, P., Tryfonas, T.,
Macdonald, J., & Taylor, C. (2022). Towards Civil
Engineering 4.0: Concept, workflow and application of
Digital Twins for existing infrastructure. Automation in
Construction, 141(July), 104421.
https://doi.org/10.1016/j.autcon.2022.104421
PTI. (2019). $60 billion investment coming in gas
infrastructure: Dharmendra Pradhan. The Economic
Times.
https://economictimes.indiatimes.com/industry/energy
/oil-gas/60-billion-investment-coming-in-gas-
infrastructure-dharmendra-
pradhan/articleshow/71566401.cms?from=mdr
Rajput, S., Sabharwal, N., Singh, A., Shingan, B., & Yadav,
B. P. (2022). City Gas Distribution Incident Analysis in
India using Pareto Principle: A Comprehensive
Analysis. Journal of Failure Analysis and Prevention,
22(3), 899–911. https://doi.org/10.1007/s11668-022-
01422-9
Rosen, R., Von Wichert, G., Lo, G., & Bettenhausen, K. D.
(2015). About the importance of autonomy and digital
twins for the future of manufacturing. IFAC-
PapersOnLine, 28(3), 567–572.
https://doi.org/10.1016/j.ifacol.2015.06.141
Schleich, B., Anwer, N., Mathieu, L., & Wartzack, S.
(2017). Shaping the digital twin for design and
production engineering. CIRP Annals - Manufacturing
Technology, 66(1), 141–144.
https://doi.org/10.1016/j.cirp.2017.04.040
Semeraro, C., Lezoche, M., Panetto, H., & Dassisti, M.
(2021). Digital twin paradigm: A systematic literature
review. In Computers in Industry (Vol. 130).
https://doi.org/10.1016/j.compind.2021.103469
Shahat, E., Hyun, C. T., & Yeom, C. (2021). City digital
twin potentials: A review and research agenda.
Sustainability (Switzerland), 13(6), 1–20.
https://doi.org/10.3390/su13063386
Sircar, A., Yadav, K., Rayavarapu, K., Bist, N., & Oza, H.
(2021). Application of machine learning and artificial
intelligence in oil and gas industry. Petroleum
Research, 6(4), 379–391.
https://doi.org/10.1016/j.ptlrs.2021.05.009
Status, C., & Trends, F. (2020). Industry 4.0 - Current
Status and Future Trends. In Industry 4.0 - Current
Status and Future Trends.
https://doi.org/10.5772/intechopen.86000
Tao, F., Sui, F., Liu, A., Qi, Q., Zhang, M., Song, B., Guo,
Z., Lu, S. C. Y., & Nee, A. Y. C. (2019). Digital twin-
driven product design framework. International
Journal of Production Research, 57(12), 3935–3953.
https://doi.org/10.1080/00207543.2018.1443229
Conceptualizing a Digital Twin Model for Natural Gas Retailing in a Geographic Area in India
955