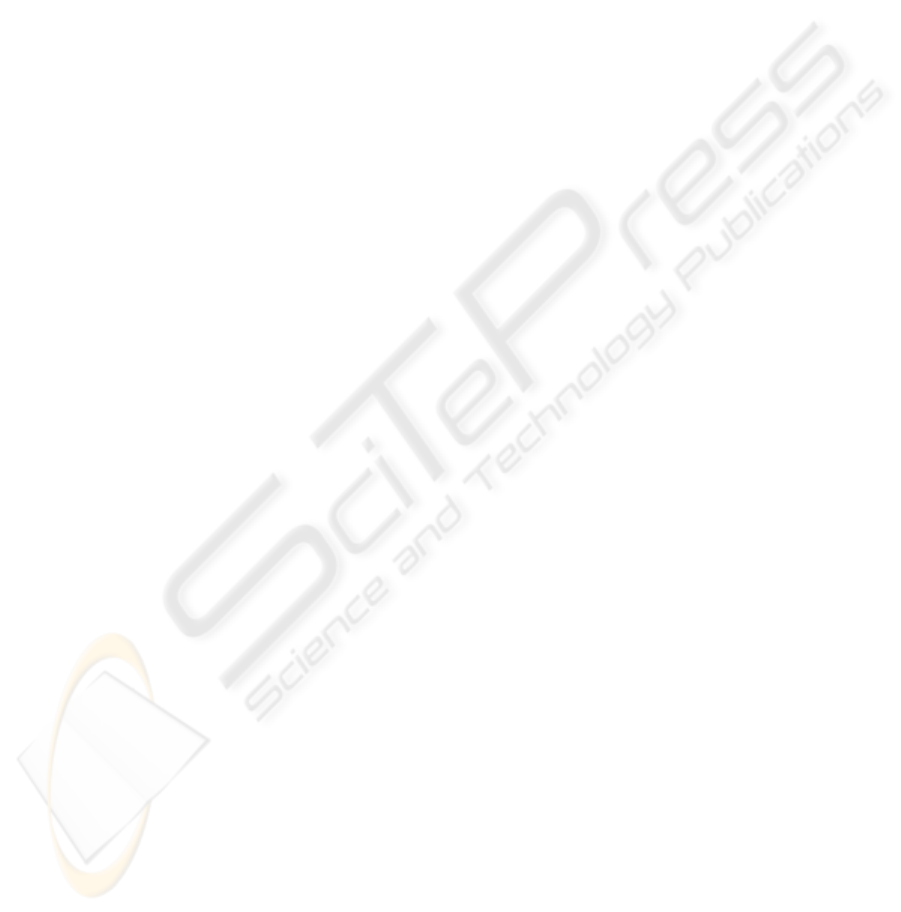
5 CONCLUSIONS
In this work we developed a longitudinal analy-
sis of bidding behavior considering reactivity pat-
terns through bidder-auction interactions. In order
to do this, we first apply a reactivity characterization
methodology for online auctions, presented in recent
research, to identify auction negotiation patterns as
well as bidding behavior in a real case study of an
online auction’s service (eBay).
We then analyze the bidding behavior evolution
over time by considering the sequence of exhibited
bidding behavior by each bidder. We represent these
sequences as a directed graph (Bidding Behavior
Model Graph) in which each bidding behavior pro-
file is a vertex and each transition (that represents a
temporal change in the bidder’s profile) is an edge.
Analyzing this graph we identify some changes
in bidding behavior over time. We observe that ini-
tially bidders tend to act during earlier stages of the
auction negotiation without competition. Later, when
they acquire more experience they start acting close
to the end of the auction with high competition. We
proceeded to divide the longitudinal dataset in differ-
ent periods and apply this same approach on each pe-
riod. We observe the same trend in each sub-period of
the dataset, and conclude that the patterns of changes
are not random. We also apply this approach us-
ing different previously established auction negotia-
tion patterns to demonstrate that the negotiation influ-
ences the evolution of bidder behavior. We are able
to demonstrate that the reactivity patterns that bidders
are subject to during negotiation affect the bidding be-
havior evolution.
The results can be applied to define seller’s strate-
gies, forecasting of economic models, or to design de-
cision support tools for e-commerce, for example.
As future work, we want to conduct a detailed
analysis of how reactivity influences bidding behav-
ior evolution, identifying the main factors that affect
it. Since auctions involve both bidders and sellers,
we also plan to generate insights on how sellers learn
over time.
REFERENCES
Agrawal, R. and Srikant, R. (1995). Mining sequential pat-
terns. In Yu, P. S. and Chen, A. S. P., editors, Eleventh
International Conference on Data Engineering, pages
3–14, Taipei, Taiwan. IEEE Computer Society Press.
Akula, V. and Menasc´e, D. (2007). Two-level workload
characterization of online auctions. Electronic Com-
merce Research and Applications Journal, 6(2):192–
208.
Ariely, D. and Simonson, I. (2003). Buying, bidding, play-
ing, or competing? value assessment and decision dy-
namics in online auctions. Journal of Consumer Psy-
chology, 13(1):113–123.
Auctions, Y. (2003). Yahoo!, Inc. http:// auc-
tions.yahoo.com/.
Bajari, P. and Hortacsu, A. (2003). The winner’s curse,
reserve prices, and endogenous entry: Empirical in-
sights from ebay auctions. RAND Journal of Eco-
nomics, 34(2):329–55.
Bapna, R., Goes, P. B., Gupta, A., and Jin, Y. (2004).
User heterogeneity and its impact on electronic auc-
tion market design: An empirical exploration research
essays. MIS Quarterly, 28(1).
Bock, H.-H. (2002). Data mining tasks and methods - clas-
sification: the goal of classification. pages 254–258.
Brown, K. N., Burke, D. A., Hnich, B., and Tarim, A.
(2005). The trading agent competition as a test prob-
lem for constraint solving under change and uncer-
tainty. In Proc. Workshop on Constraint Solving under
Change and Uncertainty, CP, pages 9–12.
EBay (2007). eBay, Inc. http://www.ebay.com/.
Falkowski, T., Bartelheimer, J., and Spiliopoulou, M.
(2006). Mining and visualizing the evolution of sub-
groups in social networks. In WI ’06: Proceedings
of the 2006 IEEE/WIC/ACMInternational Conference
on Web Intelligence, pages 52–58, Washington, DC,
USA. IEEE Computer Society.
Hartigan, J. (1975). Clustering Algorithms. John Wiley and
Sons, Inc.
Menasc´e, D. and Akula, V. (2003). Towards workload char-
acterization. In Proceedings of the IEEE 6th Annual
Workshop on Workload Characterization (WWC-6),
Austin, Texas, USA. IEEE Computer Society.
Menasc´e, D. A. and Almeida, V. A. F. (2000). Scaling for
E Business: Technologies, Models, Performance, and
Capacity Planning. Prentice Hall PTR, Upper Saddle
River, NJ, USA.
Pereira, A., Rocha, L., ao, F. M., Jr., W. M., and G´oes, P.
(2007a). Characterizing bidding behavior in internet
auctions. In NAEC’07: Proceedings of Networking
and Electronic Commerce Research Conference, Lake
Garda, Italy.
Pereira, A., Rocha, L., ao, F. M., Torres, T., G´oes, P., and
Jr., W. M. (2007b). A hierarchical characterization
model for online auctions. In ICISTM’07: Proceed-
ings of the First International Conference on Infor-
mation Systems, Technology and Management, New
Delhi, INDIA.
Pereira, A., Rocha, L., ao, F. M., Torres, T., Jr., W. M.,
and G´oes, P. (2007c). Analyzing ebay negotiation pat-
terns. In WEBIST’07: Proceedings of the 3rd Inter-
national Conference on Web Information Systems and
Technologies, Barcelona, SPAIN.
Tseng, V. S., Chang, J.-C., and Lin, K. W. (2006). Min-
ing and prediction of temporal navigation patterns for
personalized services in e-commerce. In SAC ’06:
Proceedings of the 2006 ACM symposium on Applied
computing, pages 867–871, New York, NY, USA.
ACM Press.
WEBIST 2008 - International Conference on Web Information Systems and Technologies
430