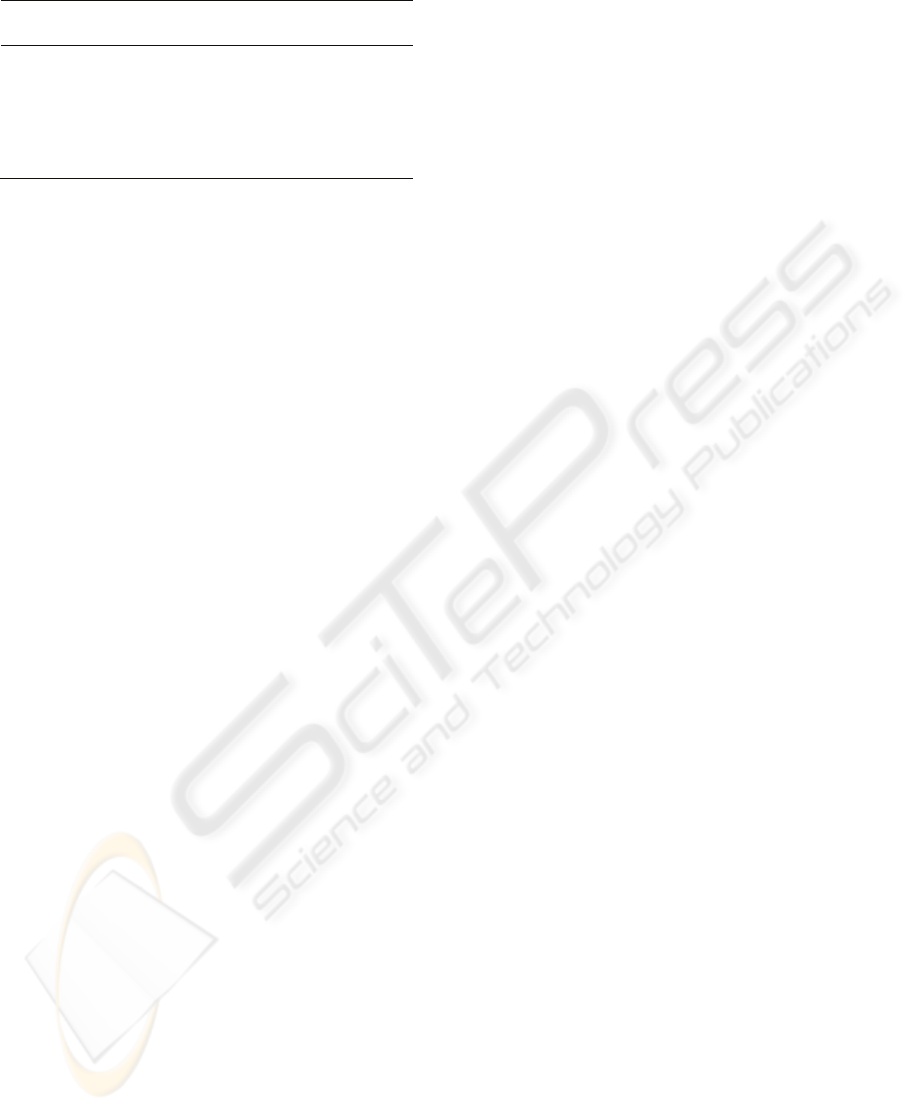
Table 4: Summary of Results.
Independent
Variables
Effect on Dependent
Variable
Volume √
Valence √
% of Negative Reviews √
% of Positive Reviews √
Product Type √
Product Lifecycle (PLC) Partial support
new product sales may lead to the poor results.
The findings reveal the significant effect of
WOM including the multiple indicators of WOM
including volume, valence and the ratio of negative
vs. positive reviews on new product sales in the
online setting. The findings of this study indicate
several interesting practical directions for
practitioners. Marketers need to observe and respond
to online WOM communication actively. They
should develop strategies to promote consumer
advocacy, to encourage consumer reviews and other
forms of WOM, and hopefully generate positive
reviews when they launch new products. Given the
speed and wide reach of online WOM, the benefit of
satisfied customers as the best advertisement can be
amplified many times. This applies to both
experience and search products, but more so for
experience products, which are subject to greater
influence from online WOM because experience
products have less tangible attributes than search
products.
Positive consumer reviews can help reduce the
uncertainty and risks associated with purchasing
new products for potential buyers. Marketers may
incorporate valuable consumer feedback, especially
the negative WOM, in the development and
marketing of new products. Meanwhile, it is not
necessary for practitioners to discourage and
manipulate the negative reviews in the forums,
because according to our finding, online negative
reviews may not directly hurt new product sales as
long as the overall effect of WOM is positive.
Finally, the effect of online WOM on new product
sales is perhaps more influential beyond the
introductory stage of a product’s life cycle for
experience products, calling for greater efforts to
monitor and respond to online WOM.
REFERENCES
Bass, F.M., 1969. A new product growth model for
consumer durables. Management Science, 15(5), 215-
227.
Brown, J., Broderick, J.A., and Lee, N. 2007. Word of
mouth communication within online communities:
conceptualizing the online social network. Journal of
Interactive Marketing, 21(3), 2-10.
Brown, J.J and Reingen, P.H. 1987. Social ties and word-
of-mouth referral behavior. Journal of Consumer
Research, 14(3), 350-362.
Chevalier, J.A. and Mayzlin, D. 2006. The effect of word
of mouth on sales: online book reviews. Journal of
Marketing Research, XLIII, 345-354
Dellarocas, C. 2003. The digitization of word of mouth:
Promise and challenges of online feedback
mechanisms. Management Science, 49(10), 1407-
1424.
Dellarocas, C., Zhang, X. and Neveen, F.A. 2007.
Exploring the value of online product reviews in
forecasting sales: The case of motion pictures. Journal
of Interactive Marketing, 21(4), 23-45.
DoubleClick. 2004. DoubleClick’s Touchpoints II: The
Changing Purchase Process. March.
Duan, W., Gu, B., and Whinston, A.B. 2008. Do online
reviews matter? - An empirical investigation of panel
data. Decision Support Systems, 45(4), 1007-1016.
Godes, D. and Mayzlin, D. 2004. Using online
conversation to study word-of-mouth communication.
Marketing Science, 23(4), 545-561.
Herr, P.M., Kardes, F.R., and Kim, J. 1991. Effects of
word-of-mouth and product-attribute information on
persuasion: An accessibility-diagnosticity perspective.
Journal of Consumer Research, 17(4), 454-462.
Kanouse, D.E. and Hanson, L.R. 1972. Negativity in
evaluations. In E. E. Jones et al. (Eds.), Attribution:
Perceiving the Causes of behavior. Morristown, NJ:
General Learning Press.
King, M.F., and Balasubramanian, S.K. 1994. The effects
of expertise, end goal, and product type on adoption of
preference formation strategy. Journal of the Academy
of Marketing Science, 22(2), 146-159.
Liu, Y. 2006. Word of Mouth for movies: Its dynamics
and impact on box office revenue. Journal of
Marketing, 70, 74-89.
McGuire, W.J. 1961. The effectiveness of supportive and
refutation defenses in immunizing defenses.
Sociometry, 24, 184-197.
Reingen, P., Foster, B., Brown, J.J., and Seidman, S. 1984.
Brand congruence in interpersonal relations: A social
network analysis. Journal of Consumer Research, 11,
1-26.
Rogers, E.M. 2003. The Diffusions of Innovations, 5th ed.,
New York: The Free Press.
Van den Bulte, C. and Lilien, G. 2001. Medical Innovation
revisited: Social contagion versus marketing effort.
The American Journal of Sociology, 106(5), 1409-
1436.
Weathers, D., Sharma, S., and Wood, L.S. 2007. Effects of
online communication practices on consumer perceptions
of performance uncertainty for search and experience
goods. Journal of Retailing, 83(4), 393-401.
ICE-B 2009 - International Conference on E-business
150