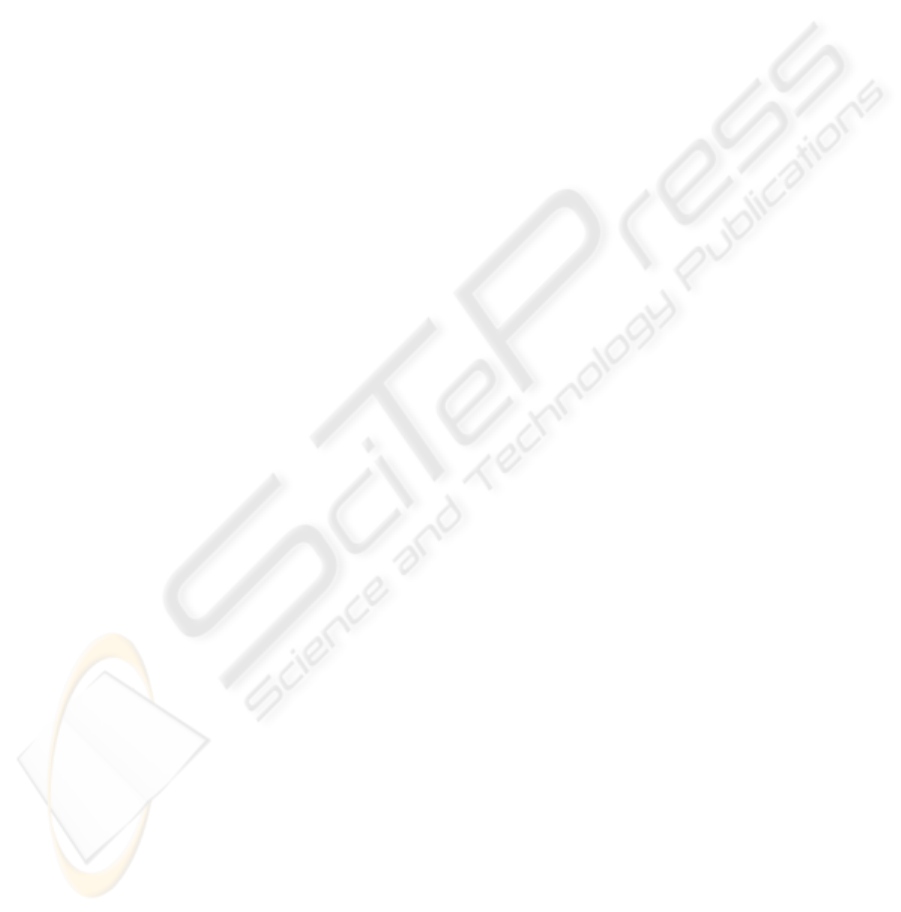
displaying organizational rules when necessary),
selecting and filtering learning content/context
according to the organization policy, and the like.
The IntelLEO platform is supposed to reduce the
communication bottlenecks featuring current
business processes at INI (compare the directions
and the intensity of communication in Figs. 1 and 2).
Note that both LKB services and harmonization
services in this IntelLEO target not only INI
employees, but also GOOD OLD AI members. The
idea is that collaboration between INI and GOOD
OLD AI in this IntelLEO is a two-way LKB process:
• INI obtains guidance from GOOD OLD AI in
finding more easily content, tools, technologies
and human resources relevant for the company's
business (metrics, evaluations, best practices,
and the like);
• GOOD OLD AI gets access to real-world
business cases and situations where they can
apply their research results and ICT tools and
validate them in an industrial setting.
3 LEARNING SCENARIOS
In this specific IntelLEO, several learning scenarios
are envisioned and the IntelLEO platfotm is
designed to support them. Two such scenarios are
described here.
Each learning scenario, in turn, is further
analyzed by one or two more specific usage
scenarios, and each usage scenario is illustrated by a
UML use-case diagram (Fowler & Scott, 1999). In
these diagrams, use cases roughly correspond to
simple learning services that the entire IntelLEO
concept is structured about. Hence usage scenarios
actually represent specific and more complex
application services, composed by orchestrating
more atomic learning services. Application services
are specific to this IntelLEO. However, many of
their parts – the constituting learning services – are
rather general and can be used as components of
application services and learning scenarios in other
SMEs that might want to collaborate with external
research teams.
3.1 IntelLEO Pedagogical Baseline
The pedagogical baseline of all learning scenarios
presented here and of the IntelLEO concept as a
whole is based on the knowledge conversion modes
(Nonaka & Takeuchi, 1995), a handy framework for
characterizing LKB processes in a learning
organization pedagogically. In this framework, one
can speak of:
• socialization of tacit knowledge (knowledge
accumulation by individuals in an
apprenticeship manner);
• externalization of tacit knowledge into explicit
(when individuals articulate tacit concepts or
create new concepts);
• combination of explicit knowledge (primarily
group-based learning activities);
• internalization of explicit knowledge (personal
learning from activities the individuals have
participated at).
3.2 Scenario 1 –
Supporting Guided Learning
At INI, in-house seminars on specific topics are
organized for employees time after time. The
IntelLEO platform can be used to support guided
learning in this context. Occasionally, selected
employees are sent to seminars organized outside the
company. This kind of learning is welcome by INI
employees, which is a good example of
harmonization of the learners' individual interests
with organization's needs.
In-house seminars at INI are given either by an
expert from INI (i.e., a metallurgist giving a talk to
selected other employees on a topic of interest for
the learners' job responsibilities), or by an external
presenter (socialization of tacit knowledge). The
external presenter may be from any area the INI
management decides is of interest for the employees
and for the company (from various areas of
technology to language learning).
The IntelLEO platform can be used to support
this kind of learning in several ways. For example,
when a seminar is organized, IntelLEO Wikis can be
used to upload the learning resources and structure
and workout assignments and practices (learning
path creation/documentation), Figure 3. The
assignments and practices can be conducted
individually (personal learning management) or in
groups (which requires support for team
composition and collaboration, and can also benefit
from enabling social networking activities through
the IntelLEO platform). To do assignments and
practices, the learners may want to use various tools,
which makes a case for ePortfolio management.
The role of the GOOD OLD AI Lab in this
learning scenario is to recommend and possibly
provide tools to support learning activities through
the IntelLEO platform, relevant ontologies and
annotations, and support for seminar planning. For
example, working collaboratively with INI
KMIS 2009 - International Conference on Knowledge Management and Information Sharing
220