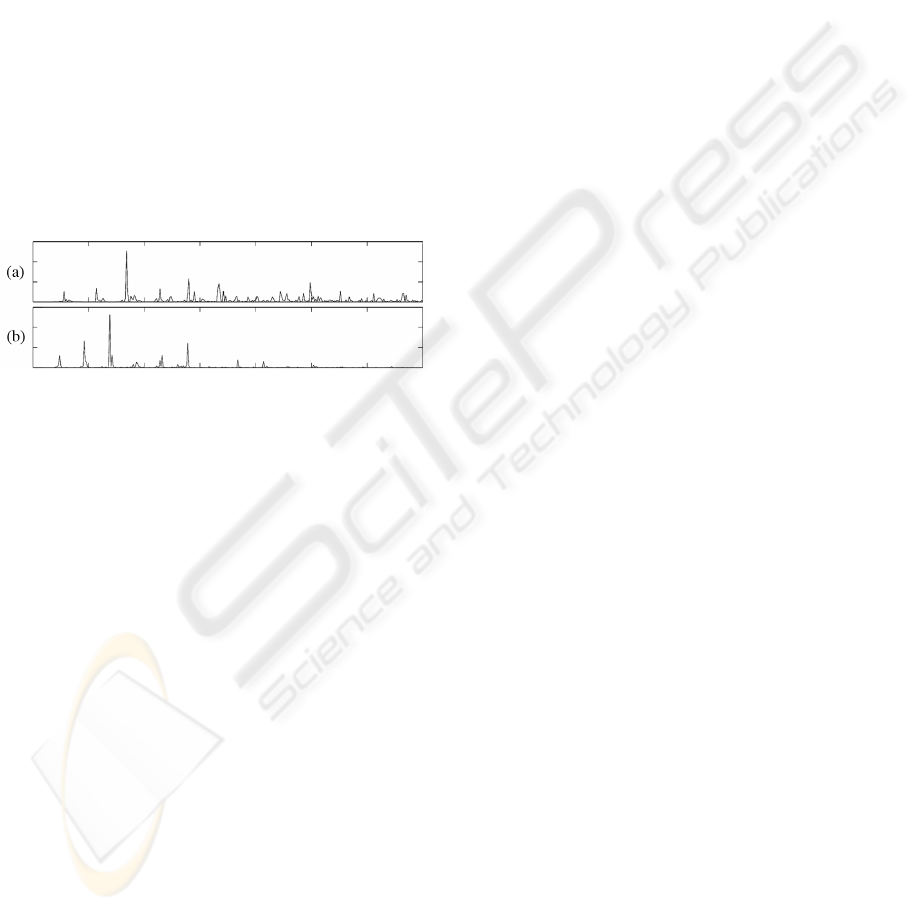
by both markers is displayed in Figs. 3 and 4.
5 DISCUSSION AND
CONCLUSIONS
In the present work spectral processing has been used
to obtain two indexes that reveal statistically signifi-
cant differences (p < 0.0001) between patients who
suffered SCD and a sample of completely healthy
subjects.
Both parameters showed values substantially
higher for patients who suffered SCD than for healthy
subjects. This fact suggests that the ECG of healthy
subjects is characterized by a higher harmonic con-
tent. Consequently, it could be considered that fre-
quency peaks of relative high amplitude in the ECG
of healthy patients are disappeared in subjects who
suffered SCD, such as Fig. 5 shows.
Figure 5: Typical PSD of an ECG for the (a) NSRDB and
(b) SCDHDB.
On the other hand, the time course of the markers
showed very constant values during the last 5 previous
hours preceding the death of the patient. Therefore,
SCD could be predicted with an anticipation above 5
hours, which suggests the problem that causes SCD,
it can be congenital or acquired during subject’s life.
Finally, given that the analyzed database are lim-
ited, the results should be considered with caution.
Nevertheless, the work suggests that SC and MFD can
initiate new lines of research as non-invasive predic-
tors of SCD. In this sense, a wider data set allowing
a more rigorous statistical analysis should be required
in order to provide confidence in the robustness of the
proposed parameters.
ACKNOWLEDGEMENTS
This work was supported by the projects PII1C09-
0036-3237 and PII2C09-0224-5983 from Junta de
Comunidades de Castilla-La Mancha.
The authors would like to acknowledge the invalu-
able helpful support received from Dr. J.L. Bardaj
´
ı,
Dr. M.L. L
´
opez, Dr. F. Madero, and Eng. M.E.
Garc
´
ıa.
REFERENCES
Al-Khatib, S. M., Sanders, G. D., Bigger, J. T., Buxton,
A. E., and et al (2007). Preventing tomorrow’s sud-
den cardiac death today: part i: Current data on risk
stratification for sudden cardiac death. Am Heart J,
153(6):941–950.
Arya, A., Haghjoo, M., and Sadr-Ameli, M. A. (2006). Risk
stratification for arrhythmic death after myocardial in-
farction: current perspective and future direction. Int
J Cardiol, 108(2):155–164.
Castells, F., Rieta, J. J., Millet, J., Zarzoso, V., and Asso-
ciate (2005). Spatiotemporal blind source separation
approach to atrial activity estimation in atrial tach-
yarrhythmias. IEEE Trans Biomed Eng, 52(2):258–
267.
Chugh, S. S., Kelly, K. L., and Titus, J. L. (2000). Sudden
cardiac death with apparently normal heart. Circula-
tion, 102(6):649–654.
Durin, O., Pedrinazzi, C., Donato, G., Pizzi, R., and Inama,
G. (2008). Usefulness of nonlinear analysis of ecg
signals for prediction of inducibility of sustained ven-
tricular tachycardia by programmed ventricular stim-
ulation in patients with complex spontaneous ventric-
ular arrhythmias. Ann Noninvasive Electrocardiol,
13(3):219–227.
Engel, G., Beckerman, J. G., Froelicher, V. F., Yamazaki,
T., and et al (2004). Electrocardiographic arrhythmia
risk testing. Curr Probl Cardiol, 29(7):365–432.
Goldberger, A. L., Amaral, L. A., Glass, L., Hausdorff,
J. M., Ivanov, P. C., and et al (2000). Physiobank,
physiotoolkit, and physionet: Components of a new
research resource for complex physiologic signals.
Circulation, 101(23):E215–E220.
Lasko, T. A., Bhagwat, J. G., Zou, K. H., and Ohno-
Machado, L. (2005). The use of receiver operat-
ing characteristic curves in biomedical informatics. J
Biomed Inform, 38(5):404–415.
Proakis, J. G. and Manolakis, D. K. (2007). Digital Signal
Processing. Principles, Algorithms and Applications.
Pretence Hall.
S
´
anchez, C., Rieta, J. J., Castells, F., Alcaraz, R., and Mil-
let, J. (2004). Wavelet domain blind signal separation
to analyze supraventricular arrhythmias from Holter
registers. Conf Proc Independent Component Analy-
sis and Blind Signal Separation, 5:1111–1117.
S
¨
ornmo, L. and Laguna, P. (2005). Bioelectrical Signal Pro-
cessing in Cardiac and Neurological Applications. El-
sevier Academic Press.
FREQUENCY DOMAIN ANALYSIS AS RISK PREDICTOR OF SUDDEN CARDIAC DEATH FROM LONG-TIME
ECG RECORDINGS
427