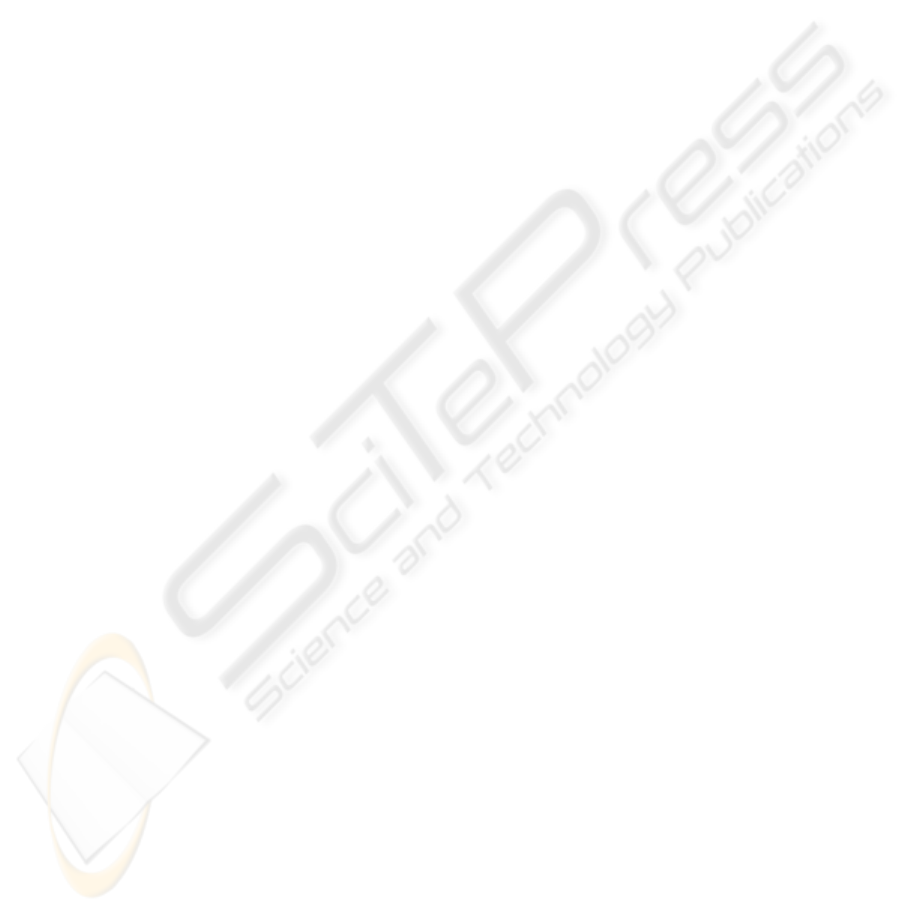
One interesting observation has to do with the
moduli features. The moduli presented many strong
correlations, five against one, two and four
occurrences for Z, Y, and X axes, respectively. They
had strong correlation between 0.2AOC and
1.0AOC for both AWLs. The moduli values can be
calculated with bi- and triaxial accelerometers and
their use can be helpful because of results
repeatability, specially the number of zero-crossings.
The RZ feature brought good perspectives to
wrist movement analyses. Table 1 shows nine strong
correlations whereas the RMS value and zero-
crossing, from where it is derived, presented six and
five correlations respectively. The use of peak
counting to determine muscle contraction was not
effective when performed concomitantly with
movement artifact, since only one correlation was
observed between 0.2AOC and 1.0AOC. Further
studies with new indicators can improve MMG
movement analysis, making unnecessary to
eliminate unwanted artifact interferences in the onset
of contraction, therefore, enhancing myoelectrical
prosthesis control.
5 CONCLUSIONS
This paper investigated mechanomyographic
analyses with 0.2 s and 1.0 s time delay after onset
of contraction during four wrist movements. The
main outcome was the great amount of correlation
between antagonist sides. In such case, the strong
correlations between 0.2AOC and 1.0AOC in
antagonist sides mean that the onset of contraction
do not interfere with the time delay. Radial deviation
has a smaller range of movement and for this reason
varying the time delays before the analyses did not
affect their correlation. Modulus was the most
frequent feature with strong correlation with varying
time delay what showed its repeatability. The
correlations were strong for antagonist movements
mainly in the inner side of the forearm.
Of all analysed features for 0.2AOC and
1.0AOC, it was demonstrated that in antagonist
movements RZ feature, zero-crossing and RMS are
very similar and can be used, if necessary, to reduce
the time delay for myoelectrical prosthesis
activation.
ACKNOWLEDGEMENTS
We would like to thank CNPq, CAPES and FINEP
for important funding and financial support and
Sidnei Schuindt for material support.
REFERENCES
Alves, N. & Chau, T. (2008) Stationarity distributions of
mechanomyogram signals from isometric contractions
of extrinsic hand muscles during functional grasping.
Journal of Electromyography and Kinesiology, 18,
509-515.
Beck, T. W., Housh, T. J., Johnson, G. O., Weir, J. P.,
Cramer, J. T., Coburn, J. W. & Malek, M. H. (2004)
Mechanomyographic and electromyographic time and
frequency domain responses during submaximal to
maximal isokinetic muscle actions of the biceps
brachii. European Journal of Applied Physiology, 92,
352-9.
Cipriano, J. J. (2003) Photographic manual of regional
orthopaedic and neurological tests, Atlanta, Georgia,
Lippincott Williams & Wilkins.
Englehart, K. & Hudgins, B. (2003) A robust, real-time
control scheme for multifunction myoelectric control.
IEEE Transactions on Biomedical Engineering 50,
848-854.
Nogueira-Neto, G. N., Müller, R. W., Salles, F. A.,
Nohama, P. & Button, V. L. S. (2008)
Mechanomyographic sensor: a triaxial accelerometry
approach. BIOSTEC. Funchal, Madeira - Portugal.
Nolan, Y. & Depaor, A. (2004) The mechanomyogram as
a channel of communication and control for the
disabled. International Conference of the IEEE EMBS.
26 ed. San Francisco.
Petitjean, M., Maton, B. & Fourment, A. (1998)
Summation of elementary phonomyograms during
isometric twitches in humans. European Journal of
Applied Physiology, 77, 527-35.
Prociow, P., Wolczowski, A., Amaral, T., Dias, O. &
Filipe, J. (2008) Identification of hand movements
based on MMG and EMG signals. BIOSTEC. Funchal,
Madeira - Portugal.
Silva, J. & Chau, T. (2003) Coupled microphone-
accelerometer sensor pair for dynamic noise reduction
in MMG signal recording. Electronics Letters, 39,
1496-8.
Smith, D. B., Housh, T. J., Johnson, G. O., Evetovich, T.
K., Ebersole, K. T. & Perry, S. R. (1998)
Mechanomyographic and electromyographic
responses to eccentric and concentric isokinetic
muscle actions of the biceps brachii. Muscle & nerve,
21.
Wojtczak, P., Amaral, T. G., Dias, O. P., Wolczowski, A.
& Kurzynski, M. (2009) Hand movement recognition
based on biosignal analysis. Engineering Applications
of Artificial Intelligence, 22, 608-615.
MECHANOMYOGRAPHIC ANALYSIS WITH 0.2 S AND 1.0 S TIME DELAY AFTER ONSET OF CONTRACTION
299