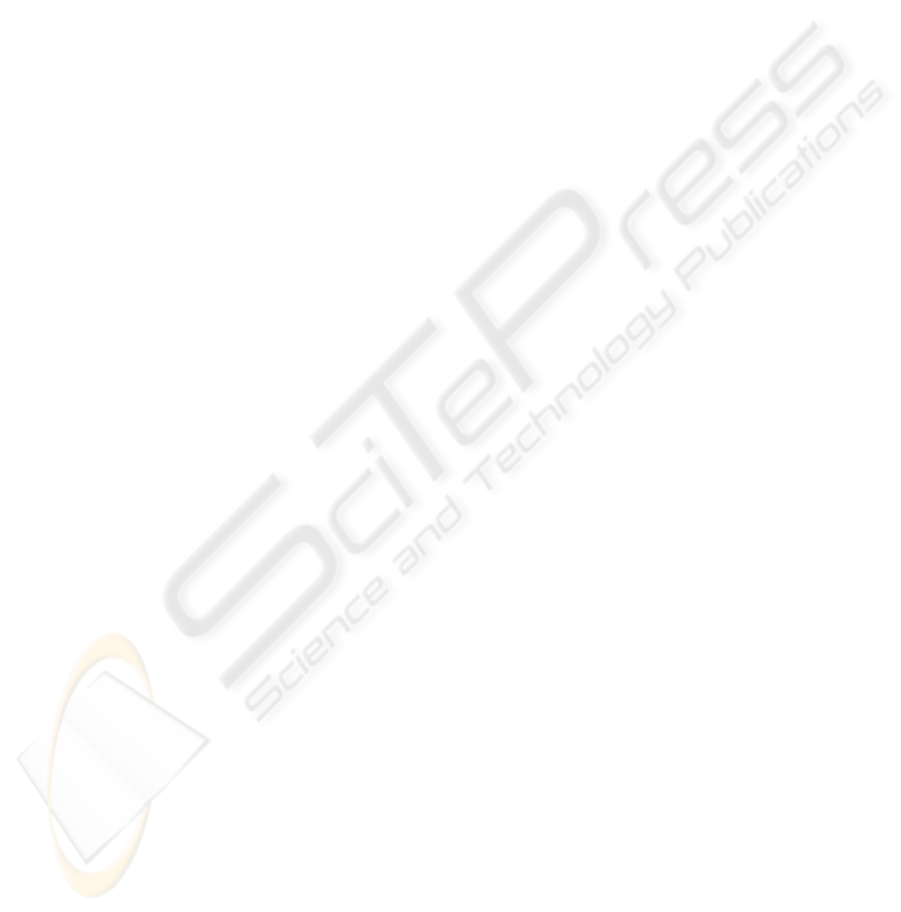
zation may explain evoked neural dynamics. Neuro-
science and biobehavioral reviews, 31(7):1003–1016.
Kronland-Martinet, R., Morlet, J., and Grossmann, A.
(1988). Analysis of sound patterns through wavelet
transforms. International Journal on Pattern Recog-
nition and Artificial Intelligence, 1(2):273–301.
Li, X., Yao, X., Fox, J., and Jefferys, J. (2007). Interac-
tion dynamics of neuronal oscillations analysed using
wavelet transforms. Journal of Neuroscience Meth-
ods, 160(1):178–185.
Mallat, S. (1999). A wavelet tour of signal processing, 2nd
edition. Academic Press, New York.
Moratti, S., Clementz, B., Gao, Y., Ortiz, T., and Keil, A.
(2007). Neural mechanisms of evoked oscillations:
Stability and interaction with transient events. Human
Brain Mapping, 28(12):1318–1333.
Nikulin, V., Linkenkaer-Hansen, K., Nolte, G., Lemm,
S., M¨uller, K., Ilmoniemi, R., and Curio, G. (2007).
A novel mechanism for evoked responses in the
human brain. European Journal of Neuroscience,
25(10):3146–3154.
Percival, D. and Walden, A. (2000). Wavelet Methods for
Time Series Analysis. Cambridge University Press,
New York.
Quiroga, R., Sakowitz, O., Bas¸ar, E., and Sch¨urmann, M.
(2001). Wavelet transform in the analysis of the fre-
quency composition of evoked potentials. Brain Re-
search Protocols, 8:16–24.
Slobounov, S., Hallett, M., Cao, C., and Newell, K. (2008).
Modulation of cortical activity as a result of voluntary
postural sway direction: An eeg study. Neuroscience
Letters, 442(3):309–313.
Stone, M. (1974). Cross-validatory choice and assessment
of statistical predictions (with discussion). Journal of
the Royal Statistical Society B, 36:111–147.
Tallon-Baudry, C., Bertrand, O., Delpuech, C., and Pernier,
J. (1996). Stimulus specificity of phase-locked and
non-phase-locked 40 hz visual responses in human.
Journal of Neuroscience, 16:4240–4249.
Uhlhaas, P. and Singer, W. (2006). Neural synchrony in
brain disorders: relevance for cognitive dysfunctions
and pathophysiology. Neuron, 52:155–168.
Vialatte, F., Bakardjian, H., Prasad, R., and Cichocki, A.
(in press 2009a). Eeg paroxysmal gamma waves dur-
ing bhramari pranayama: A yoga breathing technique.
Consciousness and Cognition.
Vialatte, F., Dauwels, J., Maurice, M., Yamaguchi, Y., and
Cichocki, A. (2009b). On the synchrony of steady
state visual evoked potentials and oscillatory burst
events. Cognitive Neurodynamics, 3(3):251–261.
Vialatte, F., Martin, C., Dubois, R., Haddad, J., Quenet, B.,
Gervais, R., and G, D. (2007). A machine learning
approach to the analysis of time-frequency maps, and
its application to neural dynamics. Neural Networks,
20:194–209.
Vialatte, F., Maurice, M., and Cichocki, A. (2008a). Why
sparse bump models? In Proceedings of OHBM meet-
ing: June 15-19 2008, Melbourne, Australia - Neu-
roimage, 41(S1):S159.
Vialatte, F., Sol´e-Casals, J., and Cichocki, A. (2008b). Eeg
windowed statistical wavelet scoring for evaluation
and discrimination of muscular artifacts. Physiolog-
ical Measurements, 29(12):1435–1452.
Vialatte, F., Sol´e-Casals, J., Dauwels, J., Maurice, M., and
Cichocki, A. (2009c). Bump time-frequency tool-
box: a toolbox for time-frequency oscillatory bursts
extraction in electrophysiological signals. BMC Neu-
roscience, 10(46).
Vialatte, F. B., Sol´e-Casals, J., Maurice, M., Latchoumane,
C., Hudson, N., Wimalaratna, S., Jeong, J., and An-
drzej, C. (2009d). Improving the quality of eeg
data in patients with alzheimers disease using ica.
In Proceedings of t15th International Conference on
Neural Information Processing, ICONIP, Auckland,
New Zealand, November 25-28 2008 - LNCS, Part II,
5507:979-986.
BIOSIGNALS 2010 - International Conference on Bio-inspired Systems and Signal Processing
484