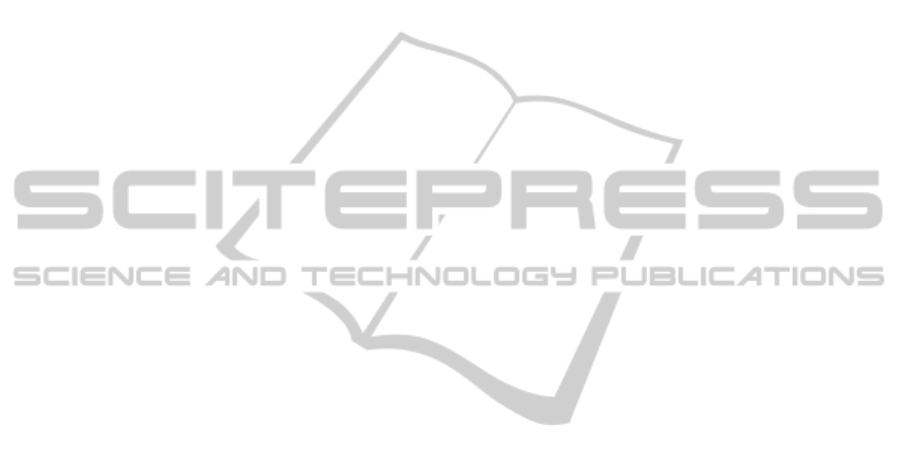
time, using knowledge from both ontologies. Con-
cepts from chosen core sets are used as a bridge be-
tween those ontologies, opening new opportunities in
knowledge integration and creating new possibilities
when interoperating with other parties.
As a next step, research on ontology merging and
integration will be performed examining new possi-
bilities emerging from usage of a common set of core
concepts.
REFERENCES
Boinski, T., Budnik, L., Jakowski, A., Mrozinski, J., and
Mazurkiewicz, K. (2009). OCS – Domain Oriented
Ontology Creation System. In SMI’09, 4th Interna-
tional Conference ’Congress of Young IT Scientists’.
HARD Olsztyn.
Enisa (2010). Enisa: a European Union
Agency - Glossary of Risk Management.
http://www.enisa.europa.eu/act/rm/cr/risk-
management-inventory/glossary.
Euzenat, J. and Shvaiko, P. (2007). Ontology matching.
Springer-Verlag New York Inc.
Fellbaum, C. et al. (1998). WordNet: An electronic lexical
database. MIT press Cambridge, MA.
Fernandez, M., Gomez-Perez, A., and Juristo, N. (1997).
Methontology: from ontological art towards ontologi-
cal engineering. In Proceedings of the AAAI97 Spring
Symposium Series on Ontological Engineering, pages
33–40.
Gennari, J. H., Musen, M. A., Fergerson, R. W., Grosso,
W. E., Crubzy, M., Eriksson, H., Noy, N. F., and Tu,
S. W. (2002). The evolution of Protege: An environ-
ment for knowledge-based systems development. Stan-
ford Medical Institute, Stanford.
Hall, D. and Hulett, D. (2002). Universal risk project, final
report. Risk Special Interest Group, PMI.
Hu, W., Jian, N., Qu, Y., and Wang, Y. (2005). Gmo: A
graph matching for ontologies. In Integrating Ontolo-
gies Workshop Proceedings, page 41. Citeseer.
Jian, N., Hu, W., Cheng, G., and Qu, Y. (2005). Falcon-
AO: Aligning ontologies with Falcon. In Integrating
Ontologies Workshop Proceedings. Citeseer.
Kissel, R. (2006). Glossary of key information security
terms. Glossary, National Institute of Standards and
Technology, US Department of Commerce.
Knight, F. (2002). Risk, uncertainty and profit. Beard Books
Inc.
Masolo, C., Borgo, S., Gangemi, A., Guarino, N.,
and Oltramari, A. (2003).
WonderWeb Deliverable
D18. Laboratory For Applied Ontology - ISTC-CNR,
Trento, Italy.
McGuiness, D. and Noy, N. (2005). Ontology development
101: a guide to creating your first ontology. Universi-
dad de Stanford.
Niles, I. and Pease, A. (2001). Towards a Standard Up-
per Ontology. In Formal Ontology in Information Sys-
tems, Proceedings of the international conference on
Formal Ontology in Information Systems, Ogunquit,
Maine, USA.
Noy, N. F., Fergerson, R. W., and Musen, M. A. (2000). The
knowledge model of Protege-2000: Combining inter-
operability and flexibility. In Lecture Notes in Com-
puter Science. Springer-Verlag.
Sommerville, I. (2006). Software Engineering. 8th. Harlow,
UK: Addison-Wesley.
Staab, S. and Studer, R. (2009). Handbook on ontologies,
Ontology Validation. Springer Verlag.
Tudorache, T., Noy, N. F., Tu, S. W., and Musen, M. A.
(2008). Supporting collaborative ontology develop-
ment in Protege. In Seventh International Semantic
Web Conference, Karlsruhe, Germany.
Waste, R. (2006). IAEA Safety Glossary.
INFLUENCE AND SELECTION OF BASIC CONCEPTS ON ONTOLOGY DESIGN
369