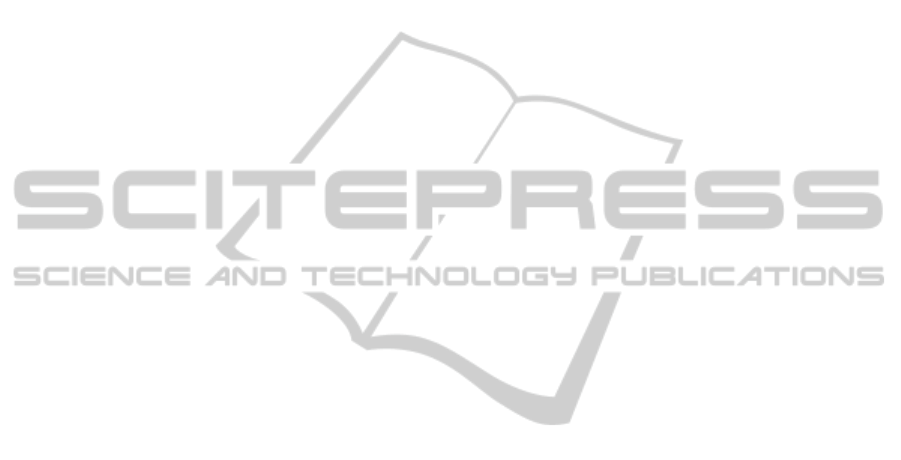
accelerometer: the first aligned with the direction of
gait progression and coincident with the
biomechanical anteroposterior (AP) axis of the
body; the second in the left/right direction and
coincident with the biomechanical mediolateral
(ML) axis of the body.
For each measure, both in ST and in DT, we
computed the mean value across the three repeated
trials for the following analyses.
To select, from all the available features (56
measures extracted from the signals, 28 for ST and
28 for DT), the subset which has the best
discriminative ability, a “wrapper” feature selection
(Kohavi & John, 1997) was implemented; the
objective function was the predictive accuracy of a
given classifier on the training set. We used the
following classifiers: linear and quadratic
discriminant analysis (LDA and QDA, respectively),
Mahalanobis classifier (MC), logistic regression
(LR), K-nearest neighbours (KNN, K=1) and linear
support vector machines (SVM). An exhaustive
search among subsets of cardinality from one to
three was implemented; the limit of three was
chosen to permit a clinical interpretation of the result
(it would be difficult to associate too many features
with different aspects of the disease). Subsets of
different cardinalities were considered separately.
The adopted procedure is similar to the one
proposed by Brewer et al. (2009) where an
exhaustive search of subsets of three features was
performed. Still, in the present study, feature
selection bias was also considered because the
available features (56) are more than the available
data (40 subjects).
Since feature selection is part of the tuning
design of the classifier, it needs to be performed on
the training set, in order to avoid the aforementioned
feature selection bias in the final evaluation of the
accuracy of the classifier (Simon, Radmacher,
Dobbin, & McShane, 2003). The most common
solution to this problem is to use a nested cross
validation procedure (Kohavi and John, 1997): the
internal feature selection step is repeated for each
training set resulting from the external cross
validation. In this study, because of the small sample
size (40), a leave-one-out cross validation (LOOCV)
was implemented both for the feature selection steps
and for the final evaluation of the classifier.
As it can be seen in figure 2, the external cross
validation used for estimation of the accuracy of the
classifier (LOOCV
ext
) splits the dataset in 40
different training and testing sets (TR
i
,TS
i
1≤i≤40);
for each TR
i
, a different feature selection step was
performed (FS
i
, 1≤i≤40). The objective function
(predictive accuracy) of each feature selection was
evaluated by an internal LOOCV (LOOCV
int
). After
each FS
i
, a list of optimal subsets of features was
generated: there was generally more than one subset
with the same highest LOOCV
int
accuracy (more
than one optimal subset). In the nested procedure TS
i
should be classified from the classifier built with a
single subset chosen by FS
i
; in this study, since more
than one optimal subset was found, it was not
possible to make a unique choice. Moreover
different FS
i
led to different lists of optimal subsets.
So we decided to extract the subset which was
selected as optimal more frequently over all the FS
i
(overall optimal subset, see figure 2). The number of
times a certain subset was selected as optimal
(selection times) can be seen as an index of how that
subset is robust to changes in the training set, and
therefore to selection bias. Eventually, the accuracy
of the classifier (misclassification rate, MR) was
computed by LOOCV
ext
for the overall optimal
subset (see figure 2).
3 RESULTS AND DISCUSSION
In table 1 the results of the feature selection
procedure for subsets of 3 measures are reported; the
estimated accuracy is presented together with the
selection times (the number of times a subset was
selected as optimal among the 40 different feature
selection procedures). Subsets of 3 measures were
preferred since subsets of lower cardinality led to
higher misclassification rates. It can be seen that a
good misclassification rate could be achieved (7.5%-
10%) by all the classifiers. As discussed in section 2,
estimates of misclassification rates of subsets with
higher selection times should be considered as more
reliable, regarding selection bias, with respect to
estimates with lower selection times. Therefore
subsets with higher selection times should be
preferred.
Considering the overall optimal subsets from all
the classifiers, the procedure always selected a
measure related with the sit-to-stand and one or two
measures related with the gait phase. In four subsets
there is also a measure extracted during stand-to-sit.
It should also be remarked that every subset
presented in table 1 is made of both single and dual
task related measures.
These measures improve the discrimination
power between CTRL and PD with respect to the
traditional TUG duration (the best misclassification
rate that can be obtained by using this single
measure with the reported classifiers, in ST or in
FEATURE SELECTION FOR THE INSTRUMENTED TIMED UP AND GO IN PARKINSON'S DISEASE
97