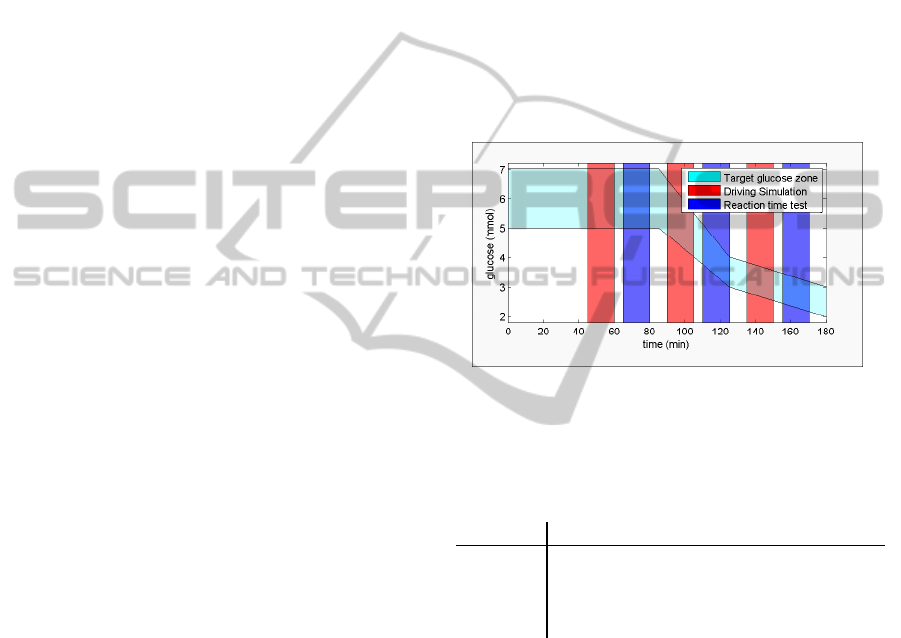
events using ECG changes.
Many of the earlier studies have used diabetic
or/and healthy test subjects (Laitinen et al., 2008;
Koivikko et al., 2008). However, it is known chronic
diabetics who have suffered from diabetes for a long
time have lower autonomic response to hypoglycemia
than subjects with sorter history of diabetes. In this
reason our dataset contains three different groups of
subjects: 1). healthy normal subjects, 2). diabet-
ics who have suffered diabetes less than 5 years and
3). chronic diabetics who have suffered from diabetes
over 5 years.
2 METHODS
Advanced PCR based method was used for analyzing
repolarization characteristics beat-by-beat (Lipponen
et al., 2010). In the PCR method each T-wave was
modeled using three optimal orthogonal basis vec-
tors. These basis vectors were obtained as the most
significant eigenvectors of correlation matrix com-
puted from 1000 previous T-wave segments. Use of
such a large number of T-wave segments was possi-
ble because no remarkable heart rate or morphology
changes where present in used measurements, and on
the other hand such a large amount of prior infor-
mation maximize the denoising effect of the model.
Similar PCR approach was applied to model the QRS
complexes.
From each estimated waveforms, Q-wave onset,
R-wave peak, T-wave peak and T-wave offset were
then extracted. From these extracted time points QT
interval, RR interval adn R/T-wave amplitude ratio
time series weere then formed. In addtition, heart
rate corrected QTc time series was formed by using
Friedricia’s method.
For time series trend estimation, smoothness pri-
ors method was used (Tarvainen et al., 2002). Before
the trend estimation each time series was transformed
evenlly sampled time series by using 4Hz cubic spline
interpolation. Used trend estimation method reflects
time-varying lowpass filter with adjustable cutoff fre-
quency which can be changed by using a smooth-
ing parameter α. Because in these time series the
effects due to off the glucose concentration changes
are shown in very low frequency range, relatively low
cutoff frequency was used.
Glucose values were measured at 5 minute inter-
vals, but values were then interpolated such that sam-
ple rate was 4 Hz, same as for all time series. Al-
though second order and seven point Savitzky-Golay
smoothing was done to beforehand to reduce mea-
surement errors, because it is highly presumable that
blood glucose value doesn’t change rapidly during 5
minutes such a smoothing is recommended.
3 MATERIALS
ECG measurements were recorded in Turku Univer-
sity Hospital and altogether 27 subjects participated
test sessions. Continuous measurements of biosig-
nals such as ECG and EEG were acquired during the
test, along with the blood glucose measurements at 5
minute intervals. In this paper, we consentrate only
on analyzis of ECG signal. ECG was recorded using
a modified chest lead V5 with sample rate 128 Hz.
Figure 1: Measurement protocol. Target glucose zone is
presented by light blue, driving simulation by red and reac-
tion time tests by dark blue.
Table 1: Ages, sexes and mean duration of diabetes (years)
of test subjects in different groups.
Healthy T1DM T1DMc
number 9 6 7
age 43.0 ± 8.9 40.5 ± 9.8 49.4 ± 11.1
sex f/m 2/7 0/6 0/7
dignosis - 3.2 ± 2.3 22.7 ± 12.7
Subjects were divided into three groups: 1) 9
nondiabetic healthy subjects (Healthy), 2) 6 diabet-
ics whose diabetes were diagnosed less than 5 years
ago (T1DM), 3) 7 chronic diabetics, diagnosied over
5 years ago and who have suffered hypoglycemic
events repeatedly (T1DMc). Characteristics of dif-
ferent groups are presented in table 1. Unfortunately
T-wave was almost invisible in five subjects ECG and
results of repolarization characteristics were not reli-
able so those measurements were removed from final
analysis.
Protocol of the measurement is presented in Fig-
ure 1. Firstly, blood glucose value was adjusted
range of 5-7 mmol to normoglycemic section. Nor-
molglycemic section lasted approximately85 minutes
and during this period first driving and reaction time
BIOSIGNALS 2011 - International Conference on Bio-inspired Systems and Signal Processing
108