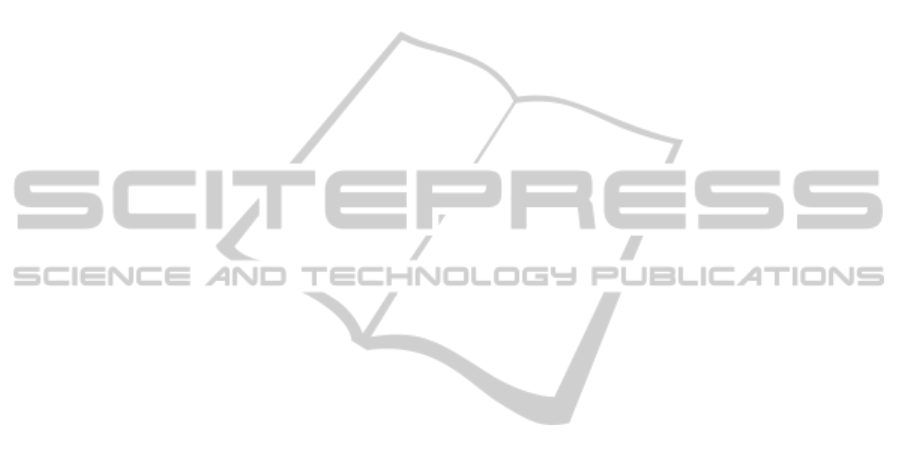
ing Addabbo et alt.(2010a) will be in a local direction,
the analysis will be replicated to a more homogeneous
area (the province of Modena). At national level we
aim to match the survey data on the population with
regional administrative data on the health infrastruc-
tures to allow for a deeper analysis of the impact of
health services on individual health outcomes.The re-
sults of our research on the construction of a new in-
dex of the capability of living a healthy life can then
be transferred - by a researcher from our research unit
- to the Italian Commission on the Definition of the
Quality of Life created in April 2011 by the Italian
National Institute of Statistics (ISTAT) and the Na-
tional Centre of Economics and Labour (Cnel) both
because health is a relevant dimension in the construc-
tion of the quality of life, and because of the innova-
tive methodology used for its measurement.
REFERENCES
Addabbo, T., Chiarolanza, A., Fuscaldo, M., and Pirotti, T.
(2010a). Una valutazione estesa del benessere sec-
ondo lapproccio delle capacit
`
a: vivere una vita sana,
chapter 7. In Baldini, M., Bosi, P., and Silvestri, P., ed-
itors, Le citt
`
a incartate, Mutamenti nel modello emil-
iano alle soglie della crisi, Bologna. Il Mulino.
Addabbo, T. and Di Tommaso, M. L. (2008). Children’s ca-
pabilities and family characteristics in Italy. Materiali
di discussione del Dipartimento di Economia Politica,
(590).
Addabbo, T., Di Tommaso, M. L., and Facchinetti, G.
(2004). To what extent fuzzy set theory and structural
equation modelling can measure functionings? An ap-
plication to child well-being. Materiali di Discussione
del Dipartimento di Economia Politica, (468).
Addabbo, T., Facchinetti, G., Maccagnan, A., Mastroleo,
G., and Pirotti, T. (2010b). A fuzzy system to evaluate
how parents interact with their children. In Metody
Informatyki Stosowanej, volume 3/2010.
Addabbo, T., Facchinetti, G., and Mastroleo, G. (2006). Ca-
pability and functionings. a fuzzy way to measure in-
teraction between father and child. In Saeed Khalid,
Pejas, Jerzy, and Mosdorf Romuald, editors, Biomet-
rics, Computer Security Systems and Artificial Intelli-
gence Applications, pages 185–195. Springer Science.
Addabbo, T. and Favaro, D. (2011). Part-time and tempo-
rary employment in a gender perspective, chapter 3.
In Addabbo, T. and Solinas, G., editors, Non standard
employment and quality of work. The case of Italy.
Springer Verlag, forthcoming.
Apolone, G., Mosconi, P., and Ware, J. E. (2005). Ques-
tionario sullo stato di salute SF12. versione italiana.
Milano. Istituto di Ricerche Farmacologiche Mario
Negri.
Cheli, B. and Lemmi, A. (1995). A “totally” fuzzy and
relative approach to the multidimensional analysis of
poverty. Economic Notes, pages 115–134.
Chiappero Martinetti, E. (2000). A multidimensional as-
sessment of well-being based on Sens functioning ap-
proach. Rivista Internazionale di Scienze Sociali,
2:207–239.
Cole, T. J., Bellizzi, M. C., Flegal, K. M., and Dietz, W. H.
(2000). Establishing a standard definition for child
overweight and obesity worldwide: international sur-
vey. British Medicine Journal, 320(7244):1240–1243.
Di Martino, M. (2008). Le condizioni di salute della popo-
lazione: Un’analisi multilivello delle disuguaglianze
sociali. In XXIX Conferenza Italiana di Scienze Re-
gionali, Bari.
Fleurbaey, M. (2009). Beyond gdp: the quest for a mea-
sure of social welfare. Journal of Economic Litera-
ture, 47(4):1029–1075.
Gandek, B., Ware Jr, J. E., Aaronson, N. K., Apolone, G.,
Bjorner, J. B., Brazier, J. E., Bullinger, M., Kaasa, S.,
Leplege, A., Prieto, L., et al. (1998). Cross-validation
of item selection and scoring for the sf-12 health sur-
vey in nine countries:: Results from the iqola project.
Journal of Clinical Epidemiology, 51(11):1171–1178.
Kuklys, W. (2003). Measurement and determinants of wel-
fare achievement-evidence. In 3rd Conference on the
Capability Approach, Pavia.
Mackenbach, J. P., Bakker, M. J., et al. (2003). Tackling so-
cioeconomic inequalities in health: analysis of Euro-
pean experiences. The Lancet, 362(9393):1409–1414.
Pirotti, T. (2006). Le dimensioni del benessere: la salute.
misure sfocate nellapproccio delle capacit
`
a. Master’s
thesis, Universit
`
a di Modena e Reggio Emilia.
Sen, A. K. (1993). Capability and well-being. In Nuss-
baum, M. and Sen, A. K., editors, The Quality of Life,
Oxford. Clarendon Press.
Sen, A. K. (1997). Inequality, unemployment and contem-
porary europe. Int’l Lab. Rev., 136:155.
Stiglitz, J. E., Sen, A. K., Fitoussi, J. P., et al. (2009). Re-
port by the commission on the measurement of eco-
nomic performance and social progress. Commission
on the Measurement of Economic Performance and
Social Progress, Paris.
United Nations Development Programmes (1990). Human
development report 1990: Concept and measurement
of human development. In UNDP, New York Oxford.
Oxford University Press.
Wagstaff, A., Paci, P., and Van Doorslaer, E. (1991). On the
measurement of inequalities in health. Social Science
& Medicine, 33(5):545–557.
Ware Jr, J. E., Kosinski, M., and Keller, S. D. (1996). A 12-
item short-form health survey: construction of scales
and preliminary tests of reliability and validity. Medi-
cal care, 34(3):220.
Ware Jr, J. E., Kosinski, M., Keller, S. D., and Institute, N.
E. M. C. H. (1998). Sf-12: How to score the sf-12
physical and mental health summary scales.
Ware Jr, J. E., Snow, K. K., Kosinski, M., and Gandek, B.
(1993). SF-36 health survey: manual and interpreta-
tion guide. The Health Institute, New England Medi-
cal Center.
FCTA 2011 - International Conference on Fuzzy Computation Theory and Applications
398