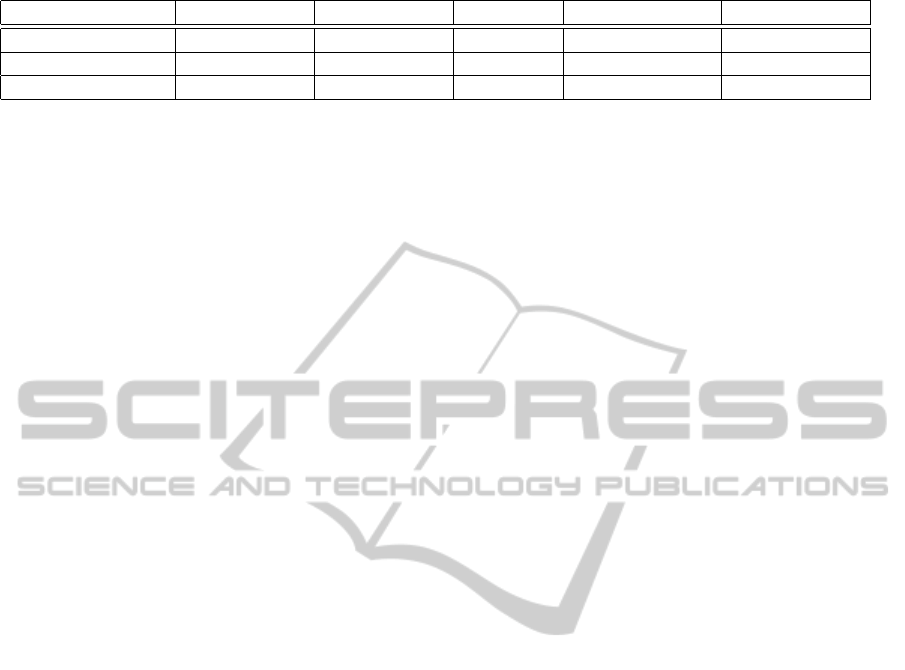
Table 3: Results of the 5-minute interval analysis.
EPISODE LABEL LF HF LF/HF VLF HRV
APN 48234±25319 72448±34804 0.69±0.29 558386±342620 303962±71490
BDL 34327±22208 53066±26431 0.65±0.41 409091±319387 256597±97733
NOR 25319±14824 38920±15508 0.63±0.19 352645±177317 239728±82953
We compared HRV indexes in segments with
and without apneic episodes using a 5-minutes
interval analysis. Results show an increase in all
indexes in apneic segments. Borderline segments,
corresponding to intervals with few apneic events,
give intermediate HRV indexes (bigger than normal
intervals and lower than apneic ones). We have
not found a similar analysis in the literature, but,
if we identify borderline intervals as “mild” apnea
intervals, our results could be compared with the
ones from (Gula et al., 2003; Park et al., 2008) that
show increments in HRV indexes in “severe” apneic
patients, compared to “mild” ones.
5 CONCLUSIONS
In this paper we present a preliminary study of apneic
patients by means of HRV using polysomnograms
acquired during siesta time. Results indicate
variations in some spectral indexes when apneic
events are present, as observed in other overnight
studies. This is an interesting result because it could
allow to significantly increase the number of patients
under observation in a sleep unit.
Although results related to the ECG siesta
recordings are promising, we must be cautious since
a more exhaustive analysis should be performed.
However, results presented in this paper suggest the
possibility of identifying apneic events in daytime
sleep, thus allowing the clinicians to use automated
systems to detect apnea in short naps.
ACKNOWLEDGEMENTS
This work has been supported by Xunta de Galicia
(PGIDIT06SIN30501PR) and the Spanish MEC and
European FEDER (TIN2009-14372-C03-03).
REFERENCES
Brindle, R. and Conklin, S. (2011). Daytime
sleep accelerates cardiovascular recovery after
psychological stress. Int J Behav Med (published
online).
Drinnan, M., Allen, J., Langley, P., and Murray, A. (2000).
Detection of sleep apnoea from frequency analysis of
heart rate variability. In Computers in Cardiology
2000, pages 259–262.
Gula, L. J., Krahn, A. D., Skanes, A., Ferguson, K. A.,
George, C., Yee, R., and Klein, G. J. (2003). Heart rate
variability in obstructive sleep apnea: a prospective
study and frequency domain analysis. Ann Noninvas
Electro, 8(2):144–149.
G
¨
unes, S., Polat, K., and Yosunkaya, S. (2010). Multi-class
f-score feature selection approach to classification of
obstructive sleep apnea syndrome. Expert Syst Appl,
37(2):998 – 1004.
Korman, M., Doyon, J., Doljansky, J., Carrier, J., Dagan,
Y., and Karni, A. (2007). Daytime sleep condenses
the time course of motor memory consolidation. Nat
Neurosci, 10(9):1206–1213.
Moody, G., Mark, R., Goldberger, A., and Penzel, T.
(2000). Stimulating rapid research advances via
focused competition: the computers in cardiology
challenge 2000. In Computers in Cardiology 2000,
volume 27, pages 207–210. Ieee.
Otero, A., Dapena, S. F., F
´
elix, P., Presedo, J., and Tarasco,
M. (2009). A low cost screening test for obstructive
sleep apnea that can be performed at the patient’s
home. In Proceedings IEEE ISP, pages 199–204.
Otero, A., F
´
elix, P., and
´
Alvarez, M. (2011). Algorithms
for the analysis of polysomnographic recordings with
customizable criteria. Expert Syst Appl, 38(8):10133
– 10146.
Park, D.-H., Shin, C.-J., Hong, S.-C., Yu, J., Ryu, S.-H.,
Kim, E.-J., Shin, H.-B., and Shin, B.-H. (2008).
Correlation between the severity of obstructive sleep
apnea and heart rate variability indices. J Korean Med
Sci, 23(2):226–231.
Penzel, T., Kantelhardt, J. W., Grote, L., Peter, J.-H.,
and Bunde, A. (2003). Comparison of detrended
fluctuation analysis and spectral analysis for heart rate
variability in sleep and sleep apnea. In IEEE Trans
Biomed Eng, volume 50, pages 1143–1151.
Roche, F., Pichot, V., Sforza, E., Court-Fortune, I.,
Duverney, D., Costes, F., Garet, M., and Barth
´
el
´
emy,
J. C. (2003). Predicting sleep apnoea syndrome from
heart period: a time-frequency wavelet analysis. Eur
Respir J, 22(6):937–942.
Rodr
´
ıguez-Li
˜
nares, L., M
´
endez, A., Lado, M., Olivieri,
D., Vila, X., and G
´
omez-Conde, I. (2011). An open
source tool for heart rate variability spectral analysis.
Comput Meth Prog Bio, 103(1):39 – 50.
HEART RATE VARIABILITY IN SIESTA POLYSOMNOGRAMS - A Preliminary Study
337