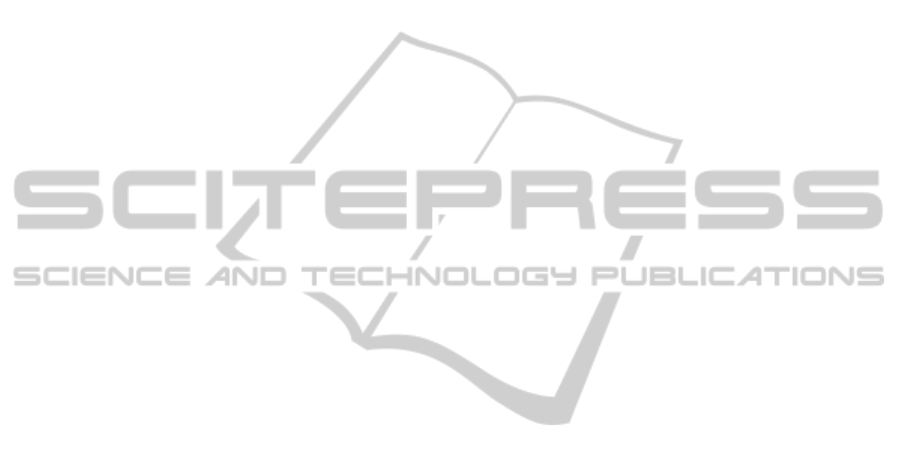
during CNS Development and Injury,". In: Proc.
Pacific Symp. Biocomputing, 1999. 41-52.
Gardner, T. 2003. Inferring genetic networks and
identifying compound mode of action via expression
profiling. Science, vol. 301, 102-105.
Ghahramani, Z. 1998. Learning Dynamic Bayesian
Networks. Adaptive Processing of Sequences and
Data Structures, Springer-Verlag, 168-197.
Holter, N. S., Maritanm, A., Cieplak, M., Fedoroff, N. V.
and Banavar, J. R. 2001. "Dynamic Modeling of Gene
Expression Data,". Proc. Nat'l Academy of Science.
USA.
Liu, T., Sung, W. and Mittal, A. 2006. Model Gene
Network by Semi-Fixed Bayesian Network. Expert
Systems with Applications, vol. 30, no.1, 42-49.
Margolin, A. A., Nemenman, I., Basso, K., Wiggins, C.,
Stolovitzky, G., Dalla Favera, R. and Califano, A.
2006. ARACNE: an algorithm for the reconstruction
of gene regulatory networks in a mammalian cellular
context. BMC Bioinformatics, 7 Suppl 1, S7.
Murphy, K. and Mian, S. 1999. Modeling Gene
Expression Data Using Dynamic Bayesian Networks.
technical report, Univ. of California.
Rangel, C., Angus, J., Ghahramani, Z., Lioumi, M.,
Sotheran, E. A., Gaiba, A., Wild, D. L. and Falciani,
F. 2004. Modeling T-Cell Activation Using Gene
Expression Profiling and State Space Models.
Bioinformatics, vol. 20, no. 9, 1361-1372.
Ronen, M., Rosenberg, R., Shraiman, B. and Alon, U.
Year. Assigning Numbers to the Arrows:
Parameterizing a Gene Regulation Network by Using
Accurate Expression Kinetics. In: Proc Nat'l Academy
Science, USA, 2002. 10555-10560.
Spellman, P., Sherlock, G., Zhang, M., Iyer, V., Anders,
K., Eisen, M., Brown, P., Botsein, D. and B, F. 1998.
Comprehensive Identification of Cell Cycleregulated
Genes of the Yeast Saccharomyces cerevisiae by
Microarray Hybridization. Molecular Biology of the
Cell, vol. 9, no. 12, 3273-3297.
Tian, T. and Burrage, K. Year. Stochastic Neural Network
Models for Gene Regulatory Networks. In: Proc. 2003
IEEE Congress Evolutionary Computation, 2003. 162-
169.
Wang, Z., Gao, H., Cao, J. and Liu, X., 2008a. “On
Delayed Genetic Regulatory Networks with Polytopic
Uncertainties: Robust Stability Analysis,”. IEEE
Trans. NanoBioscience, vol. 7, no. 2, 154-163.
Wang, Z., Liu, X., Liang, J. and Vinciotti, V., 2009. An
Extended Kalman Filtering Approach to Modeling
Nonlinear Dynamic Gene Regulatory Networks via
Short Gene Expression Time Series. IEEE/ACM
Transactions on Computational Biology and
BioinformaticS, vol. 6, no. 3, 410-419.
Wang, Z., Yang, F., Ho, D. W. C., Swift, S., Tucker, A.
and Liu, X. 2008b. Stochastic Dynamic Modeling of
Short Gene Expression Time Series Data. IEEE Trans.
NanoBioscience, vol. 7, no. 1, 44-55.
Wu, F., Zhang, W. and Kusalik, A. J. Year. Modeling
Gene Expression from Microarray Expression Data
with State-Space Equations. In: Proc. Pacific Symp.
Biocomputing, 2004. 581-592.
Yu, J., Smith, V., Wang, P. and Hartemink, A. 2004.
Advances to Bayesian Network Inference for
Generating Causal Networks from Observational
Biological Data. Bioinformatics, vol. 20, no. 18, 3594-
3603.
Zoppoli, P., Morganella, S. and Ceccarelli, M. 2010.
TimeDelay-ARACNE: Reverse engineering of gene
networks from time-course data by an information
theoretic approach. BMC Bioinformatics, 11, 154.
INFERENCE OF GENE REGULATORY NETWORKS BY EXTENDED KALMAN FILTERING USING GENE
EXPRESSION TIME SERIES DATA
155