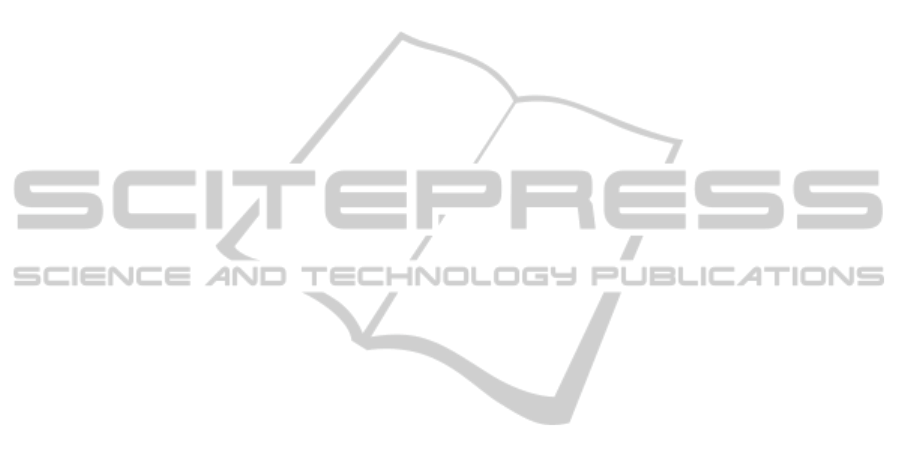
4 CONCLUSIONS
With the results presented in this paper it is clear
that there are some issues needing improvement so
the integration process can be as reliable and
consistent as possible. At the end we think that these
two systems work in an individual way and in fact
there is no real integration between them. All
registries are duplicated, i.e., each registry is
introduced manually in both applications by
different health professionals. That is a big concern
in terms of data quality as this process can lead to
different registries and even duplication of errors.
This would be avoided if the communication
between the systems was more effective reducing
the source of errors.
By analysing the results of the individual quality
of data produced by both systems, it is possible to
understand that ObsCare need additional validation
tools. In fact, there are tools implemented in this
system but, as we observed in the presented results,
they are not being as effective as desired. However it
is patent that ObsCare, because of his purpose, has
more detailed data, but not in a consistent and
complete way. There is considerable amount of
missing data, some variables have invalid values
registered and, as we verified, there are different
representations for the same variables.
The central system SONHO evidences less
interest in collecting some specific variables as they
are not as important for the system purpose.
Nevertheless some detected data problems can be
very useful to call the attention of the NHS so they
can change the way data are collected, improving his
completeness, consistence and detail.
Through the comparison, differences are clear
between both systems. The differences were
detected in every variable and table analysed. This
proves that the integration failed as there is no really
interaction. A better communication between both
systems could conduct to more reliable information
and save time in the introduction of data so that
health professionals can have more time to be
focused on patients and on research.
This is a preliminary study, and so all results
collected and presented will be further explored
during our future work. In the next steps of our
research we will be working with developers to test
and improve their validation tools and to implement
an application to scan all data and check for these
and other data quality problems. We would also like
to extend this study to other departmental systems
working at the HSJ central hospital.
ACKNOWLEDGEMENTS
The authors would like to thank the support given by
the research project HR-QoD – Quality of data
(outliers, inconsistencies and errors) in hospital
inpatient databases: methods and implications for
data modelling, cleansing and analysis (project
PTDC/SAU – ESA /75660/ 2006).
REFERENCES
Arts, D. G., De Keizer, N. F. and Scheffer, G. J., 2002.
Defining and improving data quality in medical
registries: a literature review, case study, and generic
framework. J Am Med Inform Assoc, 9, 600-11.
Cruz-Correia, R., Vieira-Marques, P., Ferreira, A.,
Oliveira-Palhares, E., Costa, P. and Costa-Pereira, A.,
2006. Monitoring the integration of hospital
information systems: How it may ensure and improve
the quality of data. Stud Health Technol Inform, 121,
176-82.
Cruz-Correia, R. J., 2010. Implementation, monitoring and
utilization of an integrated Hospital Information
System--lessons from a case study. Stud Health
Technol Inform, 160, 238-41.
Dismuke, C. E. and Sena, V., 1999. Has DRG payment
influenced the technical efficiency and productivity of
diagnostic technologies in Portuguese public
hospitals? An empirical analysis using parametric and
non-parametric methods. Health Care Manag Sci, 2,
107-16.
Freitas, A., Marques, B., Silva-Costa, T., Lopes, F.,
Garcia-Lema, I. and Costa-Pereira, A. Year. Data
Quality issues in DRG databases. In: 26th PCS
International Conference, 2010a Munich.
Freitas, A., Silva-Costa, T., Marques, B. and Costa-
Pereira, A. Year. Implications of data quality problems
within hospital administrative databases. In: 12th
mediterranean conference on medical and biological
engineering and computing – medicon 2010, 27-30
May 2010b Porto Carras, Chalkidiki, Greece.
Silva-Costa, T., Freitas, A., Jácome, J., Lopes, F. and
Costa-Pereira, A. Year. A eficácia de uma ferramenta
de validação na melhoria da qualidade de dados
hospitalares. In: CISTI - 2ª Conferência Ibérica de
Sistemas e Tecnologias de Informação, 21 a 23 de
Junho 2007 Porto.
Silva-Costa, T., Marques, B. and Freitas, A. Year.
Problemas de Qualidade de Dados em Bases de Dados
de Internamentos Hospitalares. In: 5ª Conferência
Ibérica de Sistemas e Tecnologias de Informação, 16 a
19 de Junho 2010 Santiago de Compostela.
VirtualCare. VCOBS.GYN - ObsCare [Online]. Available:
http://virtualcare.med.up.pt/index.php/en/Produtos/vco
bsgyn-eng.html [Accessed].
HEALTHINF 2012 - International Conference on Health Informatics
200