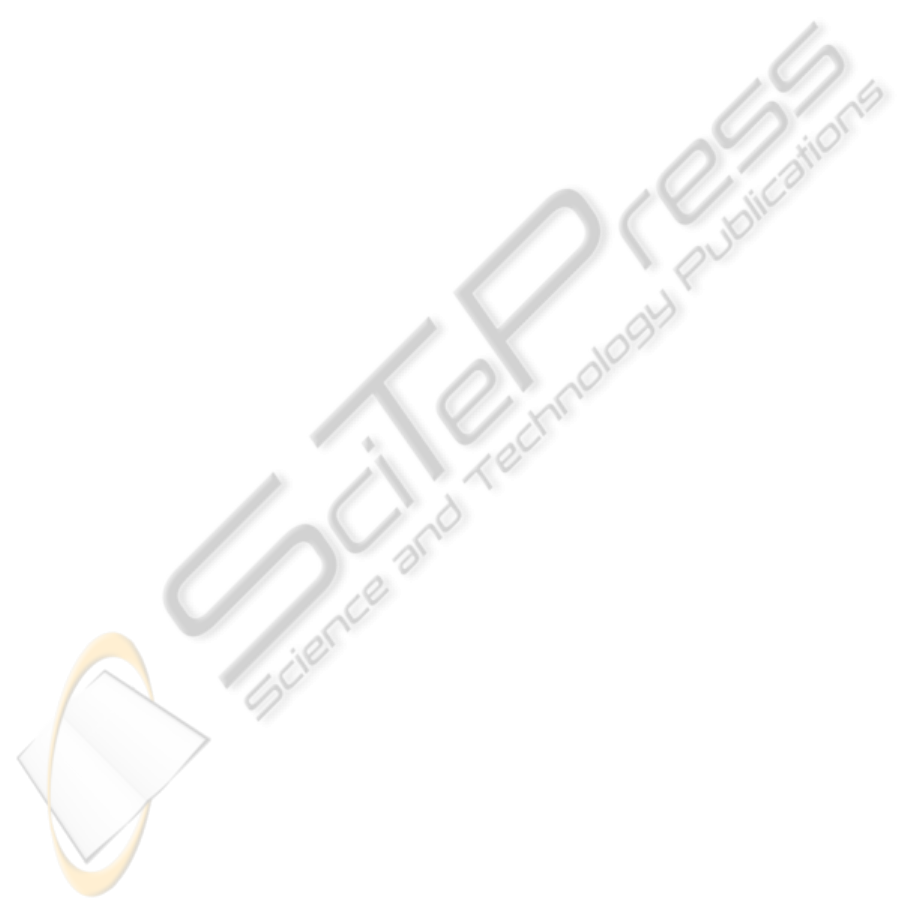
A spatial calibration method was reported in
(Reumers et al., 2005b) is used for the construction
of a transformation table for fast conversion of image
coordinates to real world coordinates. The displace-
ment between successive images is used to accelerate
the image processing algorithms and to provide more
robustness to illumination variations and to weeds.
2.2 Crop Detection Program
The crop detection system we propose is robust to
a wide range of natural conditions, highly variable
in intensity and spectral content, both over time and
space. A large range of soil and crop types (both
green and non-green) can be dealt with. Soil cover-
age by the seedlings is very small upon transplanting,
sometimes less than 1%. In horicultural applications,
the space between plants can be large, which forms
an extra objective (Southall, 1999). The image anal-
ysis algorithms are developed specifically to exploit
the contextual information of this situation.
The main features of the image-processing loop
are segmentation, line or grid fitting and determina-
tion of search region positions. The segmentation is
based on the normalised difference vegetation index
(De Smedt et al., 2011). This technique combines the
red and near-infrared information. Over monochrome
NIR images, the use of the NDVI offers the advantage
of much higher robustness to changes in illumination
intensity. The use of automatic thresholding is suffer-
ing from the low percentage of soil coverage by the
crop. Therefor at program initiation, a fixed empirical
threshold level is used. Once sufficient plants are de-
tected, search regions are constructed. The increased
soil coverage inside the search regions allows the use
of automatic thresholding. This unique approach in-
troduces exceptional robustness to changes in illumi-
nation intensity and even spectral content.
The plants within the field of view should not
touch or overlap each other and the size of the weeds
close to the crop must be smaller than the size of the
crop for a correct segmentation. Upon transplanting
of seedlings and during early crop development stages
these conditions are met.
Plant positions in a new image are predicted based
on their previous position and vehicle displacement.
The predicted positions determine the search regions
locations. New search regions are defined at the lo-
cation where new plants will enter the side of view.
This approach prevents the need to process the entire
image and analyse only the relevant information.
In the binary image resulting from the segmenta-
tion step, individual plants are identified based on size
and position. The plant centres are chosen to repre-
sent plant positions. The detected plant positions are
used to fit lines marking crop row positions. Both a
line fit method and a grid fit method are implemented,
based on least squares to minimise the distance be-
tween the fitted line/grid and the plant positions.
The line method fits a line for each row separately
and then integrates this information by the calculation
of parameter averages, weighed according to the num-
ber of plants in each row. The equations of the fitted
lines allow the calculation of the desired output values
O, θ and N. The grid fit technique increases robust-
ness and accuracy by integrating all two-dimensional
information of plant positions in a single optimisa-
tion. Still it is possible to perform a trade-off between
the accuracy importance of the longitudinal rows and
the accuracy importance of the lateral rows. The grid
fit method needs to be initialised by a previous grid
position estimate, which can be provided by the line
fit method. Note that both fit methods are performed
in the real world coordinate system. Some of the out-
put signals show high frequency noise, probably due
to camera vibrations and the nature of the application.
This can be solved by the use of a low-pass filter.
2.3 Indoor Evaluation Tests
For our experiments, we use a setup of two rails (18m)
on which an experimental vehicle is able to ride. In
between these rails soil was spread and two rows of
lettuce were planted (32 plants), according to a rect-
angular grid. This setup allows a very accurate valida-
tion of the measurement data. Two different camera
mountings where applied on the vehicle (figure 1).
During a test the vehicle was pushed manually
in order to move the vision system along the crop
rows. All experiments were conducted at four dif-
ferent driving speeds named slow (0.4 to 0.5m/s),
medium (0.7 to 1.0m/s), fast (1.3 to 1.6m/s) and very
fast (2.1 to 2.3m/s). To prevent motion blur, six halo-
gen lights (500W) where used to decrease integration
time. These are mounted on the vehicle.
To obtain validation data, the following proce-
dures were followed. Since the orientation of the cam-
era relative to the rails, and hence relative to the crop
rows, is constant during a test, the validation value of
the heading angle θ is constant for a certain camera
mounting as well. Moreover, both the crop rows and
the calibration pattern are placed parallel to the rails.
Consequently the validation value of the heading an-
gle θ is equal to 0, as during spatial calibration the
coordinate system is defined parallel to the pattern.
Note that the camera need not be aligned with the
rails. This is a big advantage, since perfect alignment
is hard to achieve. The point of interest for which
ICPRAM 2012 - International Conference on Pattern Recognition Applications and Methods
560