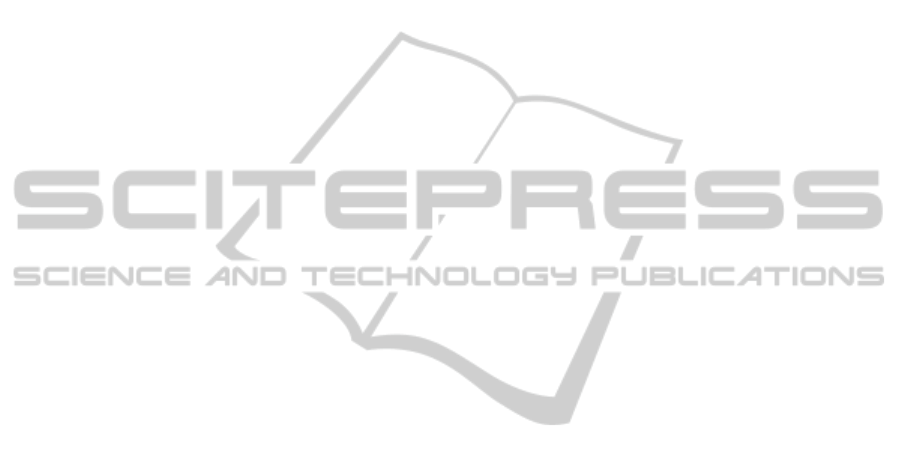
L., Lubar, J. F., & Kounios, J. (2007). EEG
neurofeedback: a brief overview and an example of
peak alpha frequency training for cognitive
enhancement in the elderly. The Clinical
Neuropsychologist, 21(1), 110-129.
Berger, H. (1929). Über das Elektrenkephalogram des
Menschen. Archiv für Psychiatrie, 87, 527-570.
Bazanova, O., & Aftanas, L. (2008). Individual EEG
alpha-indices and nonverbal creativity in female
during the spontaneous menstrual cycle in comparison
with male subjects. Annals of General Psychiatry, 7,
S101.
Cabral, R.J., & Scott, D.F. (1976). Effects of two
desensitization techniques, biofeedback and relaxation,
on intractable epilepsy: follow-up study. Journal of
Neurology, Neurosurgery, and Psychiatry, 39, 504-
507.
Chorbajian, T. (1971). Nomographic approach for the
estimation of heart rate recovery time from exercise in
humans. Journal of Applied Physiology, 31, 962-964.
Dempster, T., & Vernon, D. (2009). Identifying indices of
learning for alpha neurofeedback training. Applied
Psychophysiology and Neurofeedback, 34, 309-318.
Egner, T., Strawson, E., & Gruzelier, J.H. (2002). EEG
signature and phenomenology and alpha/theta
neurofeedback following training versus mock
feedback. Applied Psychophysiology and
Biofeedback, 27, 261-270.
Egner, T., Zech, T.F., & Gruzelier, J.H. (2004). The
effects of neurofeedback training on the spectral
topography of the electroencephalogram. Clinical
Neurophysiology, 115, 2452-2460.
Goldman, R.I., Stern, J.M., Engel Jr., J., & Cohen, M.S.
(2002). Simultaneous EEG and fMRI of the alpha
rhythm. Neuroreport, 13, 2487-2492.
Gruzelier, J.H. (2002). A review of the impact of
hypnosis, relaxation, guided imagery and individual
differences on aspects of immunity and health. Stress,
5, 147-163.
Hardt, J. V., & Kamiya, J. (1978). Anxiety change through
electroencephalographic alpha feedback seen only in
high anxiety subjects. Science, 201(4350), 79-81.
Herbert, R., & Tan, G. (2004). Quantitative EEG phase
evaluation of Transcendental Meditation. Journal of
Neurotherapy, 8(2), 120-121.
Keizer, A.W., Verschoor, M., Verment, R.S., & Hommel,
B. (2010). The effect of gamma enhancing
neurofeedback on the control of feature bindings and
intelligence measures. International Journal of
Psychophysiology, 75, 25-32.
Klimesch, W. (1997). EEG alpha rhythms and memory
processes. International Journal of Psychophysiology,
26, 319-340.
Klimesch, W. (1999). EEG alpha and theta oscillations
reflect cognitive and memory performance: a review
and analysis. Brain Research Reviews, 29, 169-195.
Klimesch, W., Sauseng, P., & Hanslmayr, S. (2007). EEG
alpha oscillations: The inhibitiontiming hypothesis.
Brain Research Reviews, 53, 63-88.
Lindsley,
D.B. (1960). Attention, Consciousness, Sleep
and Wakefulnes. Handbook of Physiology:
Neurophysiology. American Physiological Society.
McNair, D. M., Lorr, M., & Droppleman, L. F. (1971).
Manual for the Profile of Mood States. San Diego,
CA: Educational and Industrial Testing Services.
Mental Health Policies and Programmes in the
Workplace (2005). Geneva, World Health
Organization (Mental Health Policy and Service
Guidance Package).
Nowlis, D.P., & Kamiya, J. (1970). The control of
electroencephalographic alpha rhythms through
auditory feedback and the associated mental activity.
Psychophysiology, 6, 476-484.
Nunez, P.L. (1995). Neocortical Dynamics and Human
EEG rhythms. London: Oxford University Press.
Perlini, A.H., & Spanos, N.P. (1991). EEG alpha
methodologies and hypnotisability: A critical review.
Psychophysiology, 28, 511-530.
Pfurtscheller, G., & Aranibar, A. (1979). Evaluation of
event-related desynchronization (ERD) preceding and
following voluntary self-paced movement.
Electroencephalography and Clinical
Neurophysiology, 46, 138-146.
Rice, K. M., Blanchard, E. B., & Purcell, M. (1993).
Biofeedback treatments of generalised anxiety
disorder: Preliminary results. Biofeedback and Self
Regulation, 18, 93-105.
Shaw, J.C. (2003). The Brain’s Alpha Rhythms and the
Mind. Amsterdam: Elsevier.
Vander Sloten, P. Verdonck, M. Nyssen & J. Haueisen
(Eds.). IFMBE Proceedings, 22, 1022-1025.
Thatcher, R.W., Krause, P.J., & Hrybyk, M. (1976).
Cortico-cortical associations and EEG coherence: a
two-compartment model. Electroencephalograhy and
Clincical Neurophysiology, 64, 123-143.
Verleger, Gasser, T., & Möcks, J. (1982). Correction of
EOG artifacts in event-related potentials of the EEG:
Aspects of reliability and validity. Psychophysiology,
19, 472- 480.
Van Boxtel, G.J.M., Denissen, A., Jager, M., Vernon, D.,
Dekker, M.K.J., Mihaljovic, V. & Sitskoorn, M.M.
(2011). A novel approach to alpha activity training.
International journal of psychophysiology, In Press.
Vernon, D. (2005). Can neurofeedback training enhance
performance? An evaluation of the evidence with
implications for future research. Applied
Psychophysiology and Biofeedback, 30, 347-364.
Vernon, D., Dempster, T., Bazanova, O., Rutterford, N.,
Pasqualini, M., & Andersen, S. (2009). Alpha
neurofeedback training for performance enhancement:
Reviewing the methodology. Journal of Neurotherapy,
13, 1-13.
Watson, C. G., Herder, J., & Passini, F. T. (1978). Alpha
biofeedback theory in alcoholics: An 18-month
follow-up. Journal of Clinical Psychology, 34(2), 765-
769.
Welch, P.D. (1967). The use of fast Fourier transforms for
the estimation of power spectra: A method based on
time averaging over short modified periodograms.
IEEE Transactions on Audio and Electroacoustics, 15,
70-73.
HEALTHINF 2012 - International Conference on Health Informatics
486