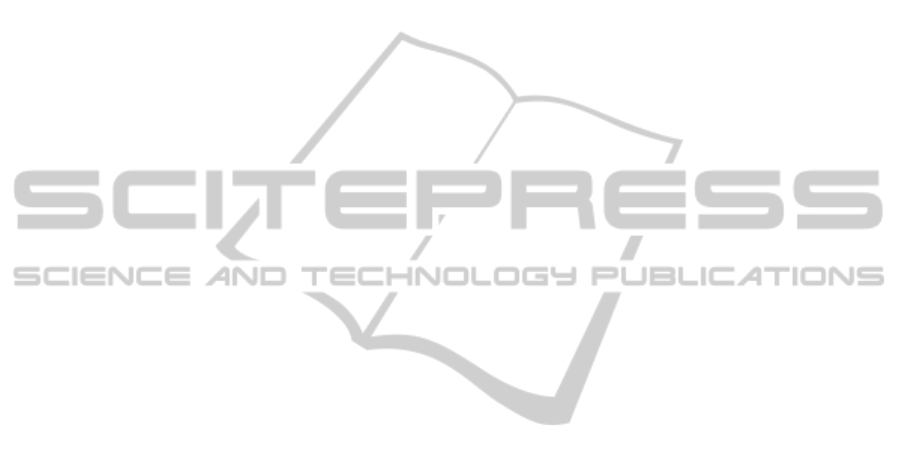
2. Biocom Technologies – Heart Rate Variability basics. http://www.biocomtech.com/hrv-
science/heart-rate-variability-basics [acessed: February 2011].
3. W. Wu, J. Lee, H. Chen, “Estimation of heart rate variability changes during different
visual stimulations using non-invasive real-time ECG monitoring system”, International
Joint Conference on Bioinformatics, System Biology and Intelligent Computing, 2009.
4. M. Malik, for the Task Force of the ESC and NASPE, “Heart rate variability: standards of
measurement, physiological interpretation and clinical use”, Circulation, vol. 93, no. 5, pp.
1043-1065, 1996.
5. M. Matsuanaga, T. Isowa, M. Miyakoshi, N. Kanayama, H. Mukarami, S. Fukuyama et. al,
“Associations among positive mood, brain, and cardiovascular activities in an affectively
positive situation”. Brain Research, vol. 1263(), pp 93-103, 2009.
6. F. C. M. Geisler, N. Vennewald, T. Kubiak and H. Weber, “The impact of heart rate
variability on subjective well-being is mediated by emotion regulation”. Personality and
Individual Differences, vol. 49(7), pp 723-728, 2010.
7. J. Taelman, S. Vandeput, A. Spaepen, and S. Van Huffel, “Influence of Mental Stress on
Heart Rate and Heart Rate Variability”. ECIFMBE 2008, IFMBE Proceedings 22, pp.
1366-1369, 2008.
8. Shimmer – Wireless Sensing Solutions, http://www.shimmer-research.com [accessed:
September 2011].
9. E. H. Hon, S. T. Lee, “Elecetronic Evaluation of the fetal heart rate patterns preceding fetal
death, further observations”. Am J Obstet Gynec col. 87, pp 814-826, 1965.
10. G. N. Healy, D. W. Dunstan, J. Salmon, and E. Cerin, “Breaks in sedentary time: Beneficial
associations with metabolic risk”. Diabetes care, Vol. 31, number 4, 2008.
11. J. Kwapisz, G. M. Weiss, S. A. Moore, “Activity recognition using cell phone
accelerometers”,Human Factors, pp 10-18, 2010. (Retrieved
from http://storm.cis.fordham.edu/~gweiss/papers/sensorKDD-2010.pdf).
12. M. J. Mathie, A. C. F. Coster, B. H. Lovell, and B. G. Celler, “Accelerometry: providing an
integrated, practical method for long-term, ambulatory monitoring of human
movement”,Journal of Physiological Measurement, vol 25(2), 2004.
13. M.T. Hamilton, D. G. Hamilton, T.W. Zderic, “Role of low energy expenditure and sitting
in obesity, metabolic syndrome, type 2 diabetes, and cardiovascular disease. Diabetes”
,56(11): 2655–2667. doi:10.2337/db07-0882. PMID:17827399, 2007.
14. Mobile Dev&Design, http://mobiledecdesign.com [accessed: September 2011].
15. V. Magagnin, M. Mauri, P. Cipresso, L. Mainardi, E.N. Brown, S. Cerutti, M.Villamira, R.
Barbieri, “Heart rate variability and respiratory sinus arrhythmia assessment of affective
states by bivariate autoregressive spectral analysis”. Computing in Cardiology (2010) 145-
148.
16. Mauri, M., Magagnin, V., Cipresso, P., Mainardi, L., Brown, E.N., Cerutti, S., Villamira,
M., Barbieri, R.: Psychophysiological signals associated with affective states. Conf Proc
IEEE Eng Med Biol Soc 2010 (2010) 3563-3566.
17. Gaggioli, G. Pioggia, G. Tartarisco, G. Baldus, D. Corda, P. Cipresso, G. Riva, “A Mobile
Data Collection Platform for Mental Health Research.” Personal and Ubiquitous
Computing, (in press).
18. A. Matic, V. Osmani, A. Popleteev, O. Mayora, “Smart Phone Sensing to Examine Effects
of Social Interactions and Non-Sedentary Work Style on Mood Changes”. Proceedings of
the 7
th
International and Interdisciplinary Conference on Modeling and Using Context
(CONTEXT ’11), Karlsruhe, Germany, 2011.
101