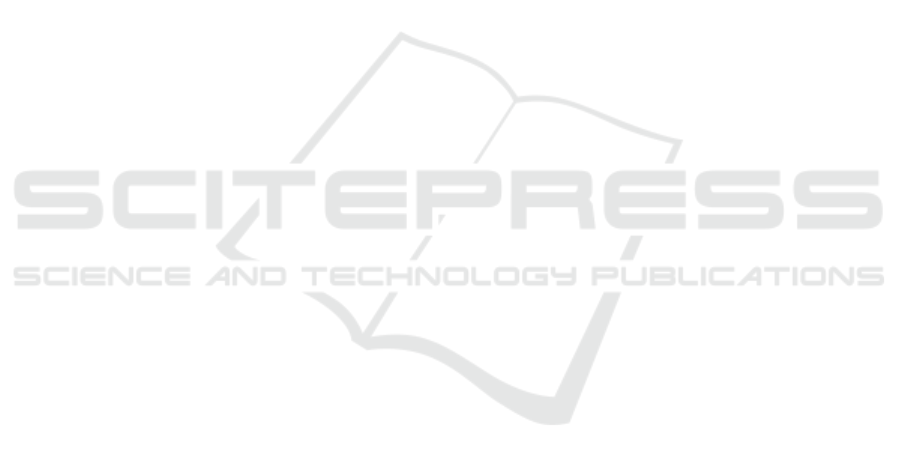
Such a model will likely exist of, amongst others, the
different products. Consider a lacking product feature
as a negative impact on the sales of the organization.
In the DuPont formula, such aspects will impact both
the operation efficiency and asset use efficiency. Con-
sequently, it will be very hard to arrive at a correct
and precise analysis of the cause of the declining ROE
by using functional decomposition. Moreover, other
terms can easily be added to the DuPont formula, or
a completely different decomposition can be made.
As a result, different analysts will arrive at different
conclusions. Based on constructional models, a more
objective analysis can be made (Dietz, 2006). There-
fore, we expect the integration of constructional mod-
els in organizational diagnosis approaches. However,
different shortcomings with regard to this expectation
can be observed.
First, many causal diagrams only focus on creat-
ing finer grained black-box models. Put differently,
they decompose a big black box into smaller black
boxes. However, they do not consider the relevance
of including constructional mechanisms. As a result,
these organizational diagnosis approaches limit them-
selves to functional decomposition, and exhibit simi-
lar shortcomings as described in the example above.
For example, Russo describes how Causal Loops Di-
agramming (CLD) can be used to specify correlation
relations between variables (Russo, 2008). However,
such approaches are only considered to be able to
predict the behavior of organizations, not to explain
the observed phenomenon (Craver, 2006). Moreover,
Woodward argues that such approaches may even fall
short when used for predicting behavior (Woodward,
2005): without constructional knowledge, it is not
possible to foresee the “conditions under which those
relations might change or fail to hold altogether”.
Second, certain approaches seem to propose to in-
clude constructional elements in the functional de-
composition in order to claim causality. By including
constructional elements, a direct relationship between
observed functional elements and constructional ele-
ments can be made. Craver calls such models “mech-
anism sketches” (Craver, 2006). Mechanism sketches
are incomplete models of a mechanism, which “char-
acterize some parts, activities, and features of the
mechanism’s organization, but [which have] gaps”
(Craver, 2006). With regard to this approach, several
reservations can be made.
• It has been argued that functional and construc-
tional models are different in nature (Dietz, 2006).
Consequently, different modeling constructs need
to be used, which makes a model harder to inter-
pret.
• Modeling the functional variables of an orga-
nization would already result in an enormous
amount of variables (Ettema, 2011). Adding addi-
tional variables will result in increasing complex-
ity, which makes the models harder to manage and
interpret.
• Adding constructional elements in an ad-hoc
manner fails to identify dependencies between
various constructional elements.
• Craver argues that the missing gaps in such mech-
anism sketches can function as “veil for a failure
of understanding” (Craver, 2006).
Third, approaches which explicitly incorporate a
constructive perspective, and separate it from beha-
vorial observations, do not offer any support on how
to model or select such a constructive perspective.
For example, we discussed the sawtooth thinking ap-
proach in LSS (see Figure 1). In this approach, the
behavioral measurements belong to the factual world,
while a constructional model would belong to the in-
terpretative world. However, no guidance to identify
relevant constructional elements is available: cause
and effect thinking in LSS is supposed to be per-
formed through “brainstorming” (Ettema, 2011).
This analysis shows that, in order to deal with the
presented complexity, current diagnosis approaches
(1) only consider functional decomposition, or (2) in-
clude constructional elements partially, or (3) include
explicitly constructional models, but do not provide
guidelines on how to construct them. Based on this
classification, it can be concluded that it is useful to
develop a method, based on a current organizational
diagnosis approach, which explicitly includes a con-
crete approach for constructing constructional mod-
els (such as, for example, Enterprise Ontology (Dietz,
2006)).
5 CONCLUSIONS
In the introduction, we started by positioning two is-
sues in the field of organizational diagnosis. We can
summarize the contributions of this paper with regard
to these issues. First, the inherent complexity of or-
ganizations makes diagnosing challenging. In regard
to this issue, this paper makes a contribution by us-
ing the concept of entropy to interpret the origin of
this complexity. Moreover, the paper shows how en-
tropy can be controlled based on insights from related
fields. We identified the presence of structure in the
constructional perspective to be primordial in control-
ling entropy. Consequently, a diagnosing approach
which attempts to address this complexity should ex-
plicitly incorporate a constructional perspective. Sec-
Second International Symposium on Business Modeling and Software Design
142