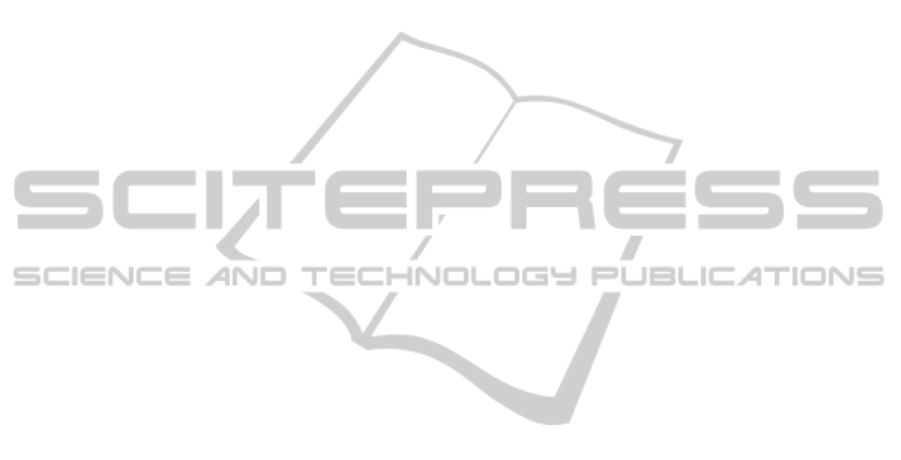
is used. The smaller difference during the measured
heating time of ironing reflects the smaller
difference in HR. Even if the detection is not perfect,
the data shows that the intensity (high/low) in which
a household activity has been performed can be
robustly detected by usage of an unobtrusive
ambient power sensor.
The mapping between the intensities of
household activities and the bicycle ergometer over
heart rate is to our knowledge the first attempt to
bring these different modalities together. The usage
of a simple fit with one parameter as a model for
energy expenditure is not sufficient for all practical
needs. For example, the model would predict
negative values when it extrapolates the energy
expenditure under certain circumstances. The most
important factor for a precise prediction of the
energy expenditure is the individual oxygen
consumption of a patient. To determine this value
the patient has to perform a load test with a cost
intensive breath by breath gas analysis. Hence, we
are currently working on a more complex model that
reflects the physiology of the human body in greater
detail. It takes the individual VO
2
consumption and
also environmental factors like temperature into
account and should, thereby, enable a more precise
prediction.
5 CONCLUSIONS
The use of the 3DLC model for the case study of an
enhanced energy expenditure determination for
COPD patients shows a way how data from the
domestic domain could be used to improve the
clinical decision making process. We substantiated
this abstract path with an experiment that was
conducted to create an intensity relation between the
telerehabilitation training on a bicycle ergometer and
the household activities ironing and vacuuming. We
showed that intensities of the activities can be
distinguished simply from the power consumption of
electrical devices that are used during the
performance of such an activity. We extracted
heating time for ironing and the frequency of
forward/backwards movements for vacuuming as
features from the power curves. These features
proved to be sufficient measures to distinguish
between two intensities in which the activities were
performed. Finally we used them for a correlation
with the ergometer training to estimate the energy
expenditure for household activities with an ambient
power sensor.
ACKNOWLEDGEMENTS
This work was funded in part by the German
Ministry of Education and Research within the
research project “OSAMI-D” (grant 01 IS 08003)
and in part by the Ministry for Science and Culture
of Lower Saxony within the Research Network
“Design of Environments for Ageing” (grant VWZN
2701).
REFERENCES
Abholz, H. H., Gillissen, A., Magnussen, H., Schott, G.,
Schultz, K., Ukena, D. and Worth, H., 2010. Nationale
Versorgungsleitlinie - Chronisch Obstruktive
Lungenerkrankung - COPD. In German.
http://www.copd.versorgungsleitlinien.de/.
Barger, T. S., Brown, D. E. and Alwan, M., 2005. Health-
status monitoring through analysis of behavioral
patterns, IEEE Transactions on Systems, Man and
Cybernetics, Part A: Systems and Humans 35(1), 22–
27.
Bauldoff, G. S., Ryan-Wenger, N. A. and Diaz, P. T.,
2007. Wrist actigraphy validation of exercise
movement in copd, Western Journal of Nursing
Research 29(7), 789–802. http://wjn.sagepub.com/
content/29/7/789.abstract.
Borg, G., 1970. Perceived exertion as an indicator of
somatic stress, Scandinavian journal of Rehabilitation
Medicine 2(2), 92–98.
Bousquet, J. and Khaltaev, N., 2007. Global surveillance,
prevention and control of Chronic Respiratory
Diseases - A comprehensive approach, Word Health
Organization.
Busch, C., Baumbach, C., Willemsen, D., Nee, O., Gorath,
T., Hein, A. and Scheffold, T., 2009. Supervised
training with wireless monitoring of ecg, blood
pressure and oxygen-saturation in cardiac patients.,
Journal of Telemedicine and Telecare 15(3), 112–114.
http://dx.doi.org/10.1258/jtt.2009.003002.
Carson, E., Cramp, D., Morgan, A. and Roudsari, A.,
1998. Clinical decision support, systems methodology,
and telemedicine: their role in the management of
chronic disease, Information Technology in
Biomedicine, IEEE Transactions on 2(2), 80 –88.
Chen, J., Zhang, J., Kam, A. H. and Shue, L., 2005. An
automatic acoustic bathroom monitoring system, in
‘Proc. IEEE Int. Symp. Circuits and Systems ISCAS
2005’, pp. 1750–1753.
Chen, Y.-P., Yang, J.-Y., Liou, S.-N., Lee, G.-Y. and
Wang, J.-S., 2008. Online classifier construction
algorithm for human activity detection using a tri-axial
accelerometer, Applied Mathematics and Computation
205(2), 849 – 860. Special Issue on Advanced
Intelligent Computing Theory and Methodology in
Applied Mathematics and Computation.
http://www.sciencedirect.com/science/article/pii/S009
InclusionofDatafromtheDomesticDomainintheProcessofClinicalDecisionMakingusingtheExampleofa
ComprehensiveAmbientEnergyExpenditureDeterminationforCOPDPatients
49