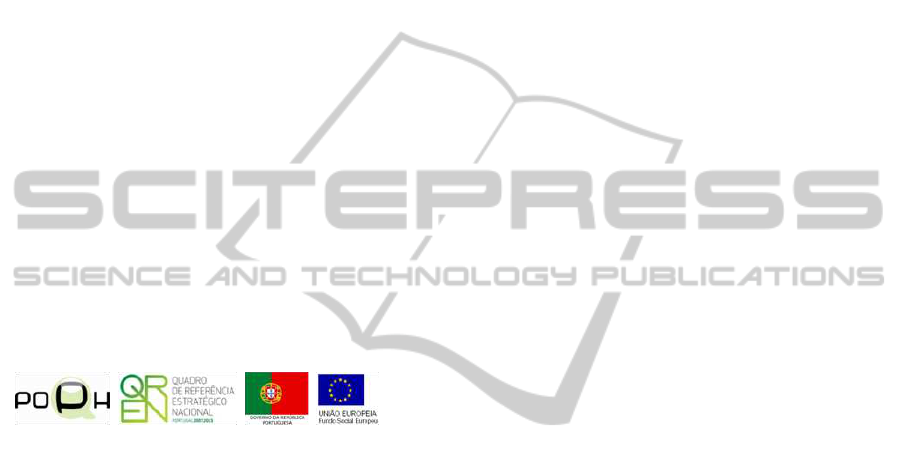
the disorganization of the process.
Further work must be developed to understand
data distribution and its variability in the plots entropy
vs. energy. A second feature to be explored in these
plots is the slope of a mathematical equation repre-
senting each data set. To know how fast and earlier in
time the curve starts to rise can reveal new useful in-
formation. These information may bring new insights
in the study of snoring and OSAS.
ACKNOWLEDGEMENTS
A special acknowledge to the sleep technicians Clara
Santos, Conceic¸˜ao Travassos, Liliana Sousa, L´ucia
Batata, Mafalda Ferreira and Mar´ılia Rodrigues for
their support in the development of this work.
There is, also, an acknowledgement to be done to
all the patients who helped the development of this
work. Without their authorization to collect data it
would be impossible this work achieve its goal.
The development of this work was supported by
the Portuguese Foundation Fundac¸˜ao para a Ciˆencia
e a Tecnologia through the reference identification
SFRH/BD/66442/2009.
REFERENCES
Banno, K. and Kryger, M. H. (2007). Sleep apnea: Clinical
investigations in humans. Sleep Medicine, 8(4):400–
426.
Barkoukis, T. J. and Avidan, A. Y. (2007). Review of Sleep
Medicine. Butterworth-Heinemann, Philadelphia, 2nd
edition.
Beck, R., Odeh, M., Oliven, A., and Gavriely, N. (1995).
The acoustic properties of snores. Eur Respir J,
8(12):2120–2128.
Fiz, J., Abad, J., Jan, R., Riera, M., Maanas, M. A., Cami-
nal, P., Rodenstein, D., and Morera, J. (1996). Acous-
tic analysis of snoring sound in patients with simple
snoring and obstructive sleep apnoea. Eur Respir J,
9(11):23652370.
Grunstein, R. R., Hedner, J., and Grote, L. (2001). Treat-
ment options for sleep apnoea. Drugs, 61(2):237–251.
Herzog, M., Khnel, T., Bremert, T., Herzog, B., Hosemann,
W., and Kaftan, H. (2009). The impact of the mi-
crophone position on the frequency analysis of snor-
ing sounds. Eur Arch Otorhinolaryngol, 266(8):1315–
1322.
Launois, S. H., Ppin, J.-L., and Lvy, P. (2007). Sleep ap-
nea in the elderly: A specific entity? Sleep Medicine
Reviews, 11(2):87–97.
Leger, D., Bayon, V., Laaban, J. P., and Philip, P. (2012).
Impact of sleep apnea on economics. Sleep Medicine
Reviews, 16(5):455–462.
Pevernagie, D., Aarts, R. M., and Meyer, M. (2010).
The acoustics of snoring. Sleep Medicine Reviews,
14(2):131–144.
Shneerson, J. M. (2005). Sleep Medicine: A Guide to Sleep
and its Disorders. Blackwell Publishing, Malden, 2nd
edition.
Spicuzza, L., Leonardi, S., and Rosa, M. (2009). Pedi-
atric sleep apnea: Early onset of the syndrome? Sleep
Medicine Reviews, 13(2):111–122.
Wittmann, V. and Rodenstein, D. O. (2004). Health care
costs and the sleep apnea syndrome. Sleep Medicine
Reviews, 8(4):269–279.
Yadollahi, A. and Moussavi, Z. M. K. (2006). A ro-
bust method for heart sounds localization using lung
sounds entropy. IEEE Transactions on Biomedical
Engineering, 53(3):497–502.
Ye, L., Pien, G. W., and Weaver, T. E. (2009). Gender differ-
ences in the clinical manifestation of obstructive sleep
apnea. Sleep Medicine, 10(10):1075–1084.
Zhang, J., Ser, W., Yu, J., and Zhang, T. (2009). A
novel wheeze detection method for wearable monitor-
ing systems. In 2009 International Symposium on In-
telligent Ubiquitous Computing and Education, pages
331–334.
Zoom (2012). H4n. Zoom Corporation.
http://www.zoom.co.jp/products/h4n.
SnoringAnalysisonFullNightRecordingsbasedintheEnergyandEntropyinPSGBasalStudies
227