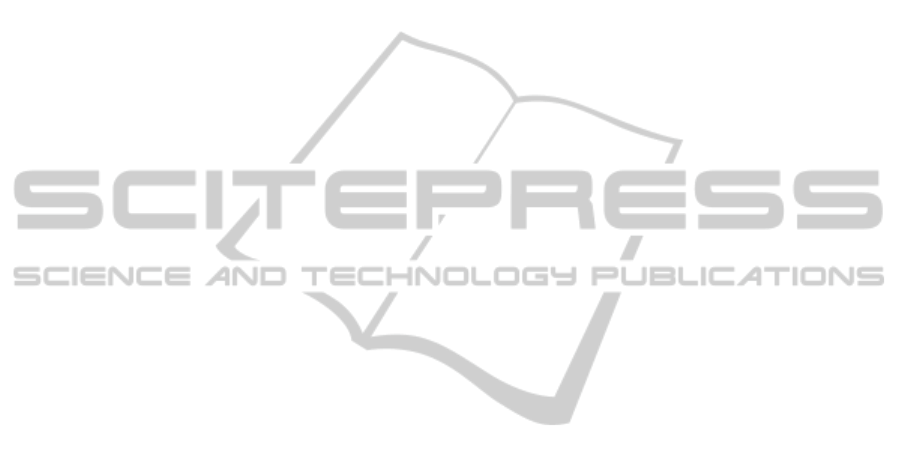
data. Socio-Economic Planning Science, 39, pp. 147–
164.
Farrell, M., 1957. The measurement of productive
efficiency. Journal of the Royal Statistical Society,
120(3), pp. 253-281.
Giuffrida, A., Gravelle, H., 2001. Measuring performance
in primary care: Econometric analysis and DEA.
Applied Economics, 33(2), pp. 163-175.
Gong, B., Sickles, R., 1992. Finite sample evidence on the
performance of stochastic frontiers and data
envelopment analysis using panel data. Journal of
Econometrics, 51, pp. 259-284.
Hollingsworth, B., 2008. The measurement of efficiency
and productivity of health care delivery. Health
Economics, 17(10), pp. 1107–1128.
Ippoliti, R., Falavigna, G., 2012. Efficiency of the medical
care industry: Evidence from the Italian regional
system. European Journal of Operational Research,
217, pp. 643-652.
Jacobs, R., 2001. Alternative methods to examine hospital
efficiency: Data Envelopment Analysis and Stochastic
Frontier Analysis. Health Care Management Science,
4, pp. 103-115.
Katharaki, M., 2008. Approaching the management of
hospital units with an operation research technique:
The Case of thirty two Greek Obstetric and
Gynaecology Public Units. Health Policy, 85(1),
pp.19-31.
Katharakis, G., Katostaras, T., 2012. SFA vs. DEA for
measuring healthcare efficiency: A systematic review.
Health Policy, (under review).
Lee, R., Bott, M., Gajewski, B., Taunton, R., 2009.
Modelling Efficiency at the Process Level: An
Examination of the Care Planning Process in Nursing
Homes. Health Services Research, 44(1), pp. 15–32.
Linna, M., 1998. Measuring hospital cost efficiency with
panel data models. Health Economics, 7, pp. 415-427.
McDonald, J., 2009. Using least squares and tobit in
second stage DEA analyses. European Journal of
Operational Research, 197, pp. 792–8.
Meeusen, W., Van den Broeck, J., 1977. Efficiency
estimation from Cobb-Douglas production function
with composed error. International Economic Review,
18, pp. 435-444.
Minvielle, E., Dervaux, B., Retbi, A., Aegerter, Ph.,
Boumendil, A., Guincestre, M., Tenaillon, A., Guidet,
B., 2005. Culture, Organizations, and Management in
Intensive Care: Construction and Validation of
Multidimensional Questionnaire. Journal of Critical
Care, 20(2), pp. 126-38.
Minvielle, E., Phillipe, A., Dervaux, B., 2008. Assessing
Organizational Performance in Intensive Care Units: A
French Experience. Journal of Critical Care, 23, pp.
236-44.
Mortimer, D., 2002. A Systematic Review of Direct DEA
vs SFA/DFA Comparisons, Working Paper 136,
Centre for Health and Evaluation, Australia.
Mutter, L., Rosko, D., Greene, H., Wilson, W., 2011.
Translating frontiers into practice: taking the next
steps toward improving hospital efficiency. Medical
Care Research and Review, 68(1), pp. 35-195.
Nedelea, C., Fannin, J., 2012. Efficiency Analysis of Rural
Hospitals: Parametric and Semi-parametric
Approaches. In Southern Agricultural Economics
Association Annual Meeting, 4-7 February 2005,
Birmingham, Alabama.
Newhouse, J., 1994. Frontier estimation: how useful a tool
for health economics? Journal of Health Economics,
13, pp. 317-322.
Ondrich, J., Ruggiero, J., 2001. Efficiency measurement in
the stochastic frontier model. European Journal of
Operational Research, 129, pp. 434-442.
Prochazkova, J., 2011. Efficiency of Hospitals in the Czech
Republic: DEA & SFA Applications. Ph.D. Faculty of
Social Sciences, Institute of Economic Studies,
Charles University in Prague.
Rosko, D., Mutter, L., 2011. What have we learned from
the application of stochastic frontier analysis to U.S.
hospitals? Medical Care Research and Review, 68(1),
pp. 75-100.
Simar, L., Wilson, P., 1998. Sensitivity analysis of
efficiency scores: How to bootstrap in nonparametric
frontier models. Management Science. 44, pp. 49–61.
Simar, L., Wilson, P., 1999. Estimating and bootstrapping
Malmquist indices. European Journal of Operational
Research, 115, pp. 459–471.
Simar, L., Wilson, P., 2000a. A General methodology for
bootstrapping in non-parametric frontier models.
Journal of Applied Statistics, 27, pp. 779–802.
Simar, L., Wilson, P., 2000b. Statistical inference in
nonparametric frontier models: The state of the art.
Journal of Productivity Analysis, 13, pp. 49–78.
Simar, L., Wilson, P., 2007. Estimation and inference in
two-stage, semi-parametric models of production
processes. Journal of Econometrics, 136, pp. 31-64.
Wilson, P., 2010. FEAR 1.15 User’s Guide. Available at:
<http://www.clemson.edu/economics/faculty/wilson/S
oftware/FEAR/FEAR-1.15/fear-user-guide.pdf>
[Accessed 25 July 2012].
AnEmpiricalComparisonofDEAandSFAMethodtoMeasureHospitalUnits'Efficiency
103