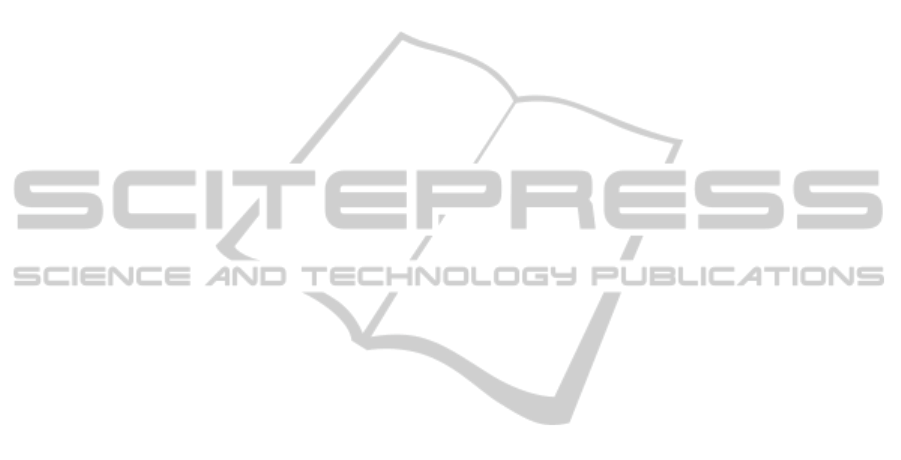
the time, the order is delivered. Otherwise, the final
customer’s demand is transferred to increase the
“Retailer stockout” variable. Based on the inventory
position, forecasted demand and lead time of this
stage, the retailer’s replenishment orders will be sent
to the distributor through the “Orders to distributor”
variable. The output of “On order products
(retailer)” is “Products delivered to retailer” which is
affected by the lead time and introduces a delay into
the arrival of products. The delay is considered to be
pure and not exponential, that means that the arrival
of products at the warehouse happens exactly after
the period defined in the “Retailer lead time”
variable.
At the distributor level, if the orders are not met
by the required date, they will be served when the
distributor has enough stock available. The proposed
model considered the orders not delivered on time as
backlogged orders and they were included in the
daily firm orders. The flow of information and
material at the distributor level undergoes the same
transformations as the previous level except the case
of backlogged orders that do not exist in the retailing
level.
The service and delivery policies for backlogged
or delayed orders at the manufacturer level follow
the same formulation as the distributor level. The
manufacturer has a predefined daily capacity, so it
can only manufacture the amount of units the factory
is capable of.
The demand forecasts are calculated during each
period based on a simple exponential smoothing
technique.
In the proposed model, the total cost is calculated
based on the unit cost, the amount of products
delivered including delivered backlogged orders,
products manufactured for the manufacturer level
and products purchased for the other two stages. It is
assumed that the distributor is the member
responsible for all the transportations taking place in
the supply chain. Therefore, the variables of
“transportation revenue” and the “transportation
cost” are included in this level. It should be
mentioned that a variable to calculate the total
revenue of each stage is not defined separately, but it
is calculated inside the formula of the profit variable.
In order to test the validity of the model, three
different tests including direct extreme conditions
test, dimentional consistency test and direct structure
test, by comparing the model equations with
available knowledge in the literature, were
conducted.
The main characteristics of the model including
model parameters and assumptions are as follows:
It is possible to serve only one part of the
order when the whole order is not available.
Inventory management is performed applying
inventory review policy.
The raw materials used for manufacturing are
considered to be available all the time.
Simulation takes place over 365 periods.
The stock of the initial inventory for the
manufacturer level is 10 units and for both,
distributor and retailer, are 5 units.
The manufacturing capacity is 25 units per
period.
The manufacturing lead time is 8 periods and
the manufacturing lead time to the distributor
and then to the retailer are 2 and 1 periods,
respectively.
The adjust factor for forecasting is equal to 2.
The pattern selected for the number of
customers entering the retail store per period
corresponds to a normal distribution with the
μ = 25 and σ = 9.
Since a customer who enters the store may
leave without buying an item, a binomial
distribution was selected to calculate the
probability that a customer will prefer a SKU
in the retail store.
Actual customer’s demand is calculated by
multiplication of the number of customers
entering the retail store and the probability
that a customer will buy an item after
entering the store.
Number of color, style and size varieties of
products are 5, 12 and 10, respectively.
3 RISK ASSESSMENT
As mentioned previously, this paper studies the
effect of demand variability and the risk of delay on
the supply chain performance of an apparel industry.
The cumulative cost in each stage of the supply
chain is used as the performance measure.
3.1 Risk of Delay
In order to investigate the impact of delay, four
different scenarios have been considered. In the
Main scenario there is no increase in the lead time of
any of the stages; in LT1 there are 6 periods of time
increase only in the lead time of manufacturer; in
LT2 there are 5 periods of time increase only in the
lead time of distributor; and in LT3 there are 2 units
of time increase only in the lead time of the retailer.
Figures 1 to 3 depict the results of the simulation. It
ICORES2013-InternationalConferenceonOperationsResearchandEnterpriseSystems
344