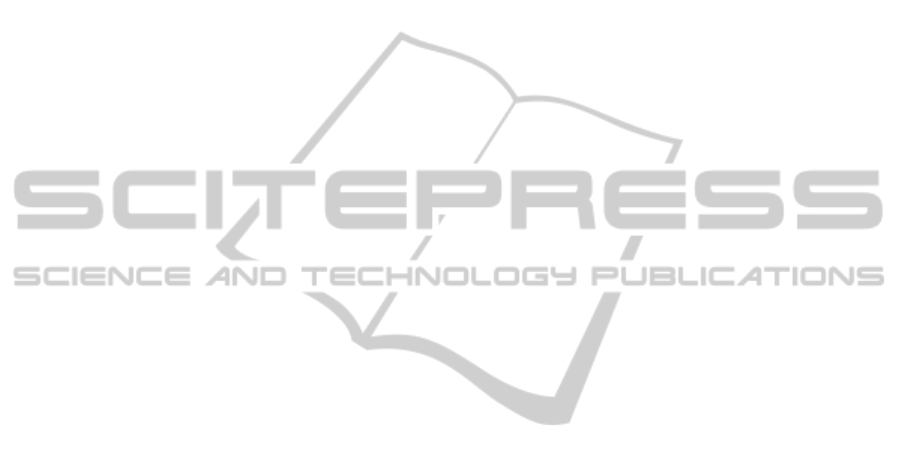
REFERENCES
Auffarth, B., Gutierrez-Galvez, A. and Marco, S., 2011.
Statistical analysis of coding for molecular properties
in the olfactory bulb. Frontiers in systems
neuroscience, 5, pp. 62.
Breitling, R., Armengaud, P., Amtmann, A. and Herzyk,
P., 2004. Rank products: a simple, yet powerful, new
method to detect differentially regulated genes in
replicated microarray experiments. FEBS letters,
573(1-3), pp. 83-92.
Falasconi, M., Gutierrez-Galvez, A., Leon, M., Johnson,
B.A. and MARCO, S., 2012. Cluster analysis of rat
olfactory bulb responses to diverse odorants. Chemical
senses, 37(7), pp. 639-653.
Fonollosa, J., Gutierrez-Galvez, A. and Marco, S., 2012.
Quality coding by neural populations in the early
olfactory pathway: analysis using information theory
and lessons for artificial olfactory systems. PloS one,
7(6), pp. e37809.
Hallem, E. A. and Carlson, J. R., 2006. Coding of odors
by a receptor repertoire. Cell, 125(1), pp. 143-160.
Hong, F., Breitling, R., Mcentee, C. W., Wittner, B. .,
Nemhauser, J. L. and Chory, J., 2006. RankProd: a
bioconductor package for detecting differentially
expressed genes in meta-analysis. Bioinformatics
(Oxford, England), 22(22), pp. 2825-2827.
Johnson, B. A. and Leon, M., 2007. Chemotopic odorant
coding in a mammalian olfactory system. The Journal
of comparative neurology, 503(1), pp. 1-34.
Johnson, B. A., Woo, C. C., Hingco, E. E., Pham, K. L.
and Leon, M., 1999. Multidimensional chemotopic
responses to n-aliphatic acid odorants in the rat
olfactory bulb. The Journal of comparative neurology,
409(4), pp. 529-548.
Leon, M. and Johnson, B. A., 2003. Olfactory coding in
the mammalian olfactory bulb. Brain research.Brain
research reviews, 42(1), pp. 23-32.
Ma, L., Qiu, Q., Gradwohl, S., Scott, A., Yu, E. Q.,
Alexander, R., Wiegraebe, W. and Yu, C. R., 2012.
Distributed representation of chemical features and
tunotopic organization of glomeruli in the mouse
olfactory bulb. Proceedings of the National Academy
of Sciences of the United States of America, 109(14),
pp. 5481-5486.
Malnic, B., Hirono, J., Sato, T. and Buck, L.B., 1999.
Combinatorial receptor codes for odors. Cell, 96(5),
pp. 713-723.
Meister, M. and Bonhoeffer, T., 2001. Tuning and
topography in an odor map on the rat olfactory bulb.
The Journal of neuroscience: the official journal of the
Society for Neuroscience, 21(4), pp. 1351-1360.
Mori, K., Takahashi, Y. K., Igarashi, K. M. and
Yamaguchi, M., 2006. Maps of odorant molecular
features in the Mammalian olfactory bulb.
Physiological Reviews, 86(2), pp. 409-433.
Pollard, K. S., Dudoit, S. and Van Der Laan, M. J., 2005.
Multiple Testing Procedures: the multtest Package and
Applications to Genomics. In: V. Carey, W. Huber, R.
Irizarry and S. Dudoit Spinger., eds, Bioinformatics
and Computational Biology Solutions Using R and
Bioconductor. Gentleman.
Smit, S., Van Breemen, M. J., Hoefsloot, H. C., Smilde,
A. K., Aerts, J. M. and De Koster, C. G., 2007.
Assessing the statistical validity of proteomics based
biomarkers. Analytica Chimica Acta, 592(2), pp. 210-
217.
Soucy, E. R., Albeanu, D. F., Fantana, A. L., Murthy, V.
N. and Meister, M., 2009. Precision and diversity in an
odor map on the olfactory bulb. Nature neuroscience,
12(2), pp. 210-220.
Takahashi, Y. K., Kurosaki, M., Hirono, S. and Mori, K.,
2004. Topographic representation of odorant
molecular features in the rat olfactory bulb. Journal of
neurophysiology, 92(4), pp. 2413-2427.
Yekutieli, D. and Benjamini, Y., 1999. Resampling-based
false discovery rate controlling multiple test
procedures for correlated test statistics. Journal of
Statistical Planning and Inference, 82(1–2), pp. 171-
196.
IdentificationofMolecularPropertiesCodingAreasinRat'sOlfactoryBulbbyRankProducts
387