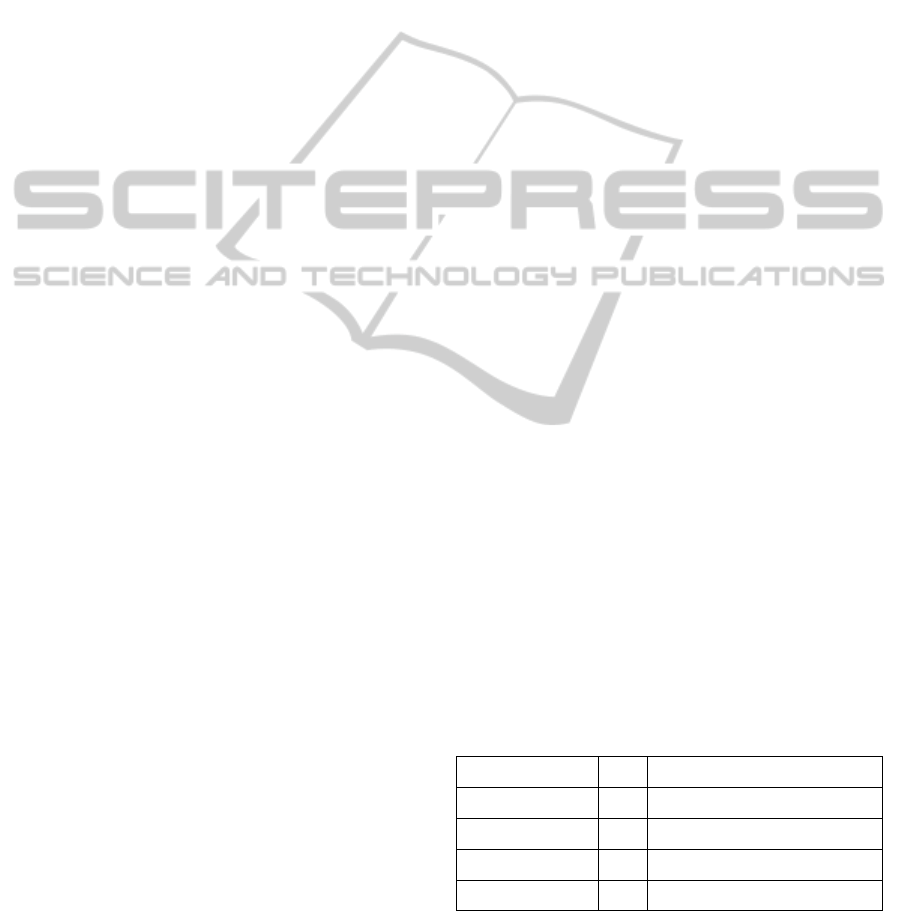
It is certainly the aim of investigation of any
field to initially classify the objects contained within
it but it is the hypothesis of this paper that although
rapes can be organized into types that these will be
far from ‘distinct’. And that the attempt to
discriminate between crimes in this way is likely not
only to be barren but actively misleading in that they
will be forced into mutually exclusive types that will
misrepresent their complexity; a perspective arrived
at after many years of research by one of the area’s
foremost investigators
… assigning criminals or crimes to one of a
limited number of ‘types’ will always be a gross
oversimplification. (Canter, 2000)
Canter and his associates who are identified with
Investigative Psychology have published numerous
studies (Hakkanen et al., 2004); (Santilla et al., 2003)
on sexual assault, homicide and other serious crimes
but have been unable in any of them to construct a
satisfactory typology with even the most relaxed
rules of assignment (Salfati and Canter, 1999).
Grubin is obliged to propose a 256 element
taxonomy in which many of the elements are
redundant, a classification system in which many if
not most of the elements will never occur cannot be
satisfactory. The assumption of the crisp set
paradigm in this research appears to be the cause of
the problems relating to these difficulties. This can
be illustrated by a simple description of a crime such
as ‘a very violent assault on a middle-aged woman
by a young man’ which cannot be properly expressed
in terms of crisp sets. It can lead to either the
misallocation of fundamentally different offences to
the same place or to crimes that bear strong
resemblances to each other being regarded as entirely
unconnected, a phenomenon referred to as linkage
blindness (Egger, 1990) of which researchers are
fully aware but have been unable to address.
In the analysis of serious crime, particularly
sexual offences, there are two computer databases
that dominate: the Violent Criminal Apprehension
Program (ViCAP) introduced by the F.B.I and the
Violent Crime Linkage System (ViCLAS) first
developed by the Royal Canadian Mounted Police
(RCMP). ViCAP is used predominantly in the USA
while ViCLAS is employed throughout most of
Europe .Both systems are essentially repositories of
criminal records that analysts search using their
training and expertise in order to link offences. This
is achieved employing straightforward Boolean
searches on groups of variables deemed to be
significant. There has been no decision support
system devised to assist in this task and no attempt
has been made to incorporate the results from
psychological research into crime linkage.
2 DATA
We have been fortunate in being successful in
obtaining data on 574 serious sexual offences from
the Serious Crimes Analysis Section of the U.K
National Policing Improvement Agency. We have
excluded those offences that do not relate to serial
stranger rapes, by which we mean a set of rapes
committed by a single individual unknown to the
victim. This results in a much narrower dataset (n =
112), development set n = 83, test = 29). The
development set consisted of 28 series, mean length
2.96, while the test set comprised 11 series with a
mean length of 2.64.
The dataset made available contained 22 single
or ‘one off’ stranger rapes which allowed variants on
the set to be constructed that could be regarded as
more realistic in that they contained a mixture of
both serial and individual offences. In the first
instance these were added to the 29 offences in Test
Set 1 to produce Test Set 2 (n = 51). By using this
set of crimes the effect of a substantial group (>
40%) of unlinked offences in tests could be
observed. Both Test Set 1 and 2 used the value of
variables derived from Development Set 1. In order
to replicate the development of a crime database
over time the entire set of 112 linked crimes was
used as Development Set 2. As with the other
development set this pool of offences generated its
own value for variables; they were found to be
somewhat different but in line with the first set.
Finally as before and for the same reasons this set
was combined with the 22 single offences to
produce a composite set of 134 linked and unlinked
offences.
Table 1:Development and test datasets.
n=
Development 1 83 83 linked crimes
Test 1 29 29 linked crimes
Test 2 51 29 linked, 22 unlinked
Development 2 112 112 linked crimes
Test 3 134 112 linked crimes, 22 unlinked
ICAART2013-InternationalConferenceonAgentsandArtificialIntelligence
384