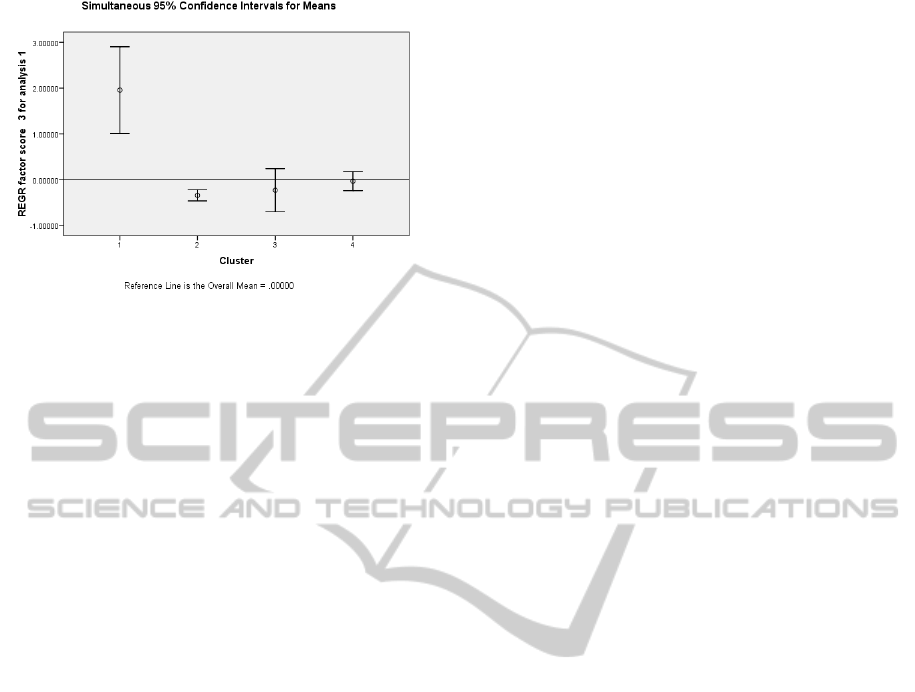
Figure 4: Factor 3 group means.
Both, cluster 1 and 2 consisted mainly of South-
Asian students whereas cluster 1 was more active
than cluster 2. Since both share the same cultural
background other reasons must exist why there is a
difference. In a further step the executive student’s
educational background, age group, current location
(domestic or expat), and job were analysed. It was
found that in the more active cluster 1 there were
significantly more SA working abroad as expats or
either having their own business or planning to have
and were on average 4 years younger. In most cases
the first two attributes exclude each other; either a
person is working as expat for a company or is
running his/her own business domestically. Whereas
one can easily understand that a business owner’s
intrinsic motivation to study may be higher than a
normal employee, the fact that expats perform better
is not that obvious. Their job and family demands
are usually higher than those working in their home
country. Therefore, one would expect that they have
less time to study. Possible reasons why they
perform better could be: rigid time management,
high motivation, and fewer distractions. Many of
Cluster 1 (Indian) students were working in the
Middle East especially UAE. Combined with the
fact that they are younger and therefore likely to be
more career-oriented may make them better
performing students. Whether a student just started
or was close to the end of the programme made
another significant difference. Students had to take
16 courses plus a Master Thesis in form of a project.
Because the analysed course was an elective it could
be taken as 5
th
course earliest and as late as 16
th
.
More experienced SA students having at least done
10 courses performed significantly better than
students taking it as 5
th
until 9
th
. It seems that there is
a learning curve and maybe a motivational push
toward the end of the programme to improve the
final GPA.
4 CONCLUSIONS
We demonstrated that cultural differences in e-
learning behaviour, assessment grade components,
and peer assessment exist. A major issue in e-
learning is whether the trend will be to greater
convergence or more divergence (Edmundson,
2006). Greater convergence would mean e-learners
worldwide are becoming more similar. More
divergence would signify that e-learners are more
likely to be significantly different (Blanchard and
Allard, 2010). This study provided support for the
divergence trend. One size will not fit all. Course
design will need to be more adaptive not more
generalisable. Unfortunately most e-learning courses
have been designed by Westerners, including the
analysed courses at the Singaporean university.
However, the fastest-growing markets are non-
Western: China and India.
5 IMPLICATIONS
Make students aware that there is a cultural bias.
Encourage EA to take the lead in group assignments.
Encourage SA to pull their weight in group
assignments.
Make students aware that viewing the organizing
tool reflects uncertainty avoidance but does not give
a better grade.
Stress DB assessment criteria to EU to achieve
more substance and less quantity.
Encourage students working as expats or
planning to start their own business to share their
experiences with others and serve as role model.
Further research in form of longitudinal studies
(Goda & Mine, 2011) should take the behavioural
changes over time into account as well as the impact
of foreign exposure such as working as expat.
REFERENCES
Blanchard, E. G., Allard, D. (2010). Handbook of
Research on Culturally Aware Information
Technology: Perspectives and Models. Hershey, PA:
Information Science Publishing.
Creswell, J. W. (2009). Mapping the field of mixed
methods research, Journal of Mixed Methods
Research, 3(2): 95-108.
Edmundson, A. (2006). Globalized E-Learning Cultural
Challenges, IGI Global Information Science
Publishing, Hershey: PA, U.S.A.
CSEDU2013-5thInternationalConferenceonComputerSupportedEducation
534