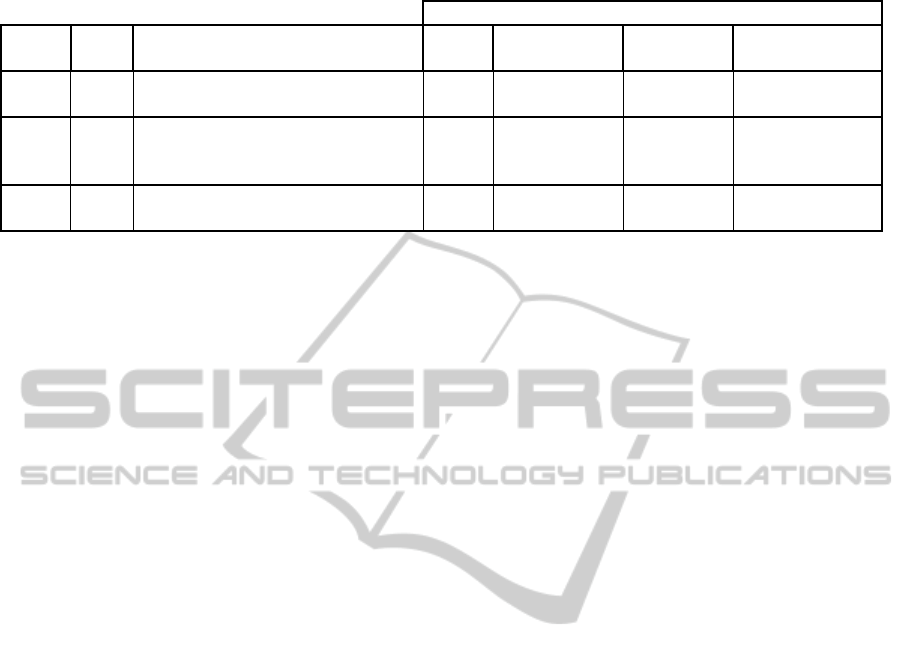
Table 6: Demographic aspects in each Profile.
Demographic aspects
Profile /
Cluster
Factors DQ Characteristics Gender Age Level of Studies Type of Organization
1 3 Novelty, Timeliness, Relevancy, Precision Female Between 45 and 55
Vocational
Training
Education
Other
2 1
Scope, Reliability, Validity, Traceability,
Compliance, Specialization, Efficiency,
Effectiveness
< 25 High School
Industrial-
commercial-financial
3 2
Flexibility, Applicability, Value-added,
Usefulness
Male
Between 25 and 45
> 55
University
Postgraduate
Services
importance on the fact that data are oriented towards
a destination community (Applicability), and users
between 25 and 35 years of age consider it relevant
that the data satisfy the users’ needs (Usefulness).
This paper also describes the criteria needed to
establish guidelines that will allow Web portal
designers and developers to discover which DQ
characteristics are most important for ‘Commercial
Interaction’ Web portal users according to their user
profiles.
As future work we shall analyse the other types
of Web portals, and the guidelines for the designers
and developers will be programmed into a free
software tool that will be available for use.
ACKNOWLEDGEMENTS
This research has been funded by the following project:
GEODAS-BC project (Ministerio de Economía y
Competitividad and Fondo Europeo de Desarrollo
Regional FEDER, TIN2012-37493-C03-01).
REFERENCES
Cappiello, C, Francalanci, C and Pernici, B., 2004, 'Data
quality assessment from the user´s perspective', in
Proceeding on International Workshop on Information
Quality in Information Systems (IQIS2004), Paris,
France. ACM, pp. 68-73.
Durndell, A and Haag, Z., 2002, 'Computer self efficacy,
computer anxiety, attitudes towards the Internet and
reported experience with the Internet, by gender, in an
East European sample', Computer in Human Behavior,
vol. 18, pp. 521-535.
Hupfer, ME and Detlor, B., 2006, 'Gender and Web
information seeking: A self-concept orientation
model.', Journal of the American Society for
Information Science and Technology, vol. 57, no. 8,
pp. 1105-1115.
Katerattanakul, P and Siau, K., 1999, 'Measuring
Information Quality of Web Sites: Development of an
Instrument', in 20th International Conference on
Information System, pp. 279-285.
Kitchenham, B and Pfleeger, S., 2002, 'Principles of
survey research part 2: designing a survey', SIGSOFT:
Software Engineering Note, vol. 27, no. 1, pp. 18-20.
Komathi, M and Maimunah, I., 2009, 'Influence of gender
role on Internet usage pattern at home among
academicians', The Journal of International Social
Research, vol. 2, no. 8.
Lenhart, A, Purcell, K, Smith, A and Zickuhr, K., 2010,
'Social Media and Mobile Internet Use Among Teens
and Young Adults', Pew Internet and American Life
Project, http://pewinternet.org/Reports/2010/Social-
Media-and-Young-Adults.aspx.
Moraga, C, Moraga, M, Calero, C and Caro, A., 2009,
'SQuaRE-Aligned Data Quality Model for Web
Portals', in 9th International Conference on Quality
Software (QSIC 2009), pp. 117-122.
Şahin, C., 2011, 'An analysis of Internet addiction levels
of individuals according to various variables', TOJET:
The Turkish Online Journal of Educational
Technology, vol. 10, no. 4, pp. 60-66.
Shanks, G and Corbitt, B., 1999, 'Understanding Data
Quality: Social and Cultural Aspects', in 10th
Australasian Conference on Information Systems,
Wellington, New Zealand, pp. 785-797.
Strong, D, Lee, Y and Wang, R., 1997, 'Data Quality in
Context', Communications of the ACM, vol. 40, no. 5,
pp. 103-110.
Wang, RY and Strong, DM., 1996, 'Beyong accuracy:
What data quality means to data consumers', Journal
of Management Information Systems, vol. 12, no. 4,
pp. 5-34.
WEBIST2013-9thInternationalConferenceonWebInformationSystemsandTechnologies
446