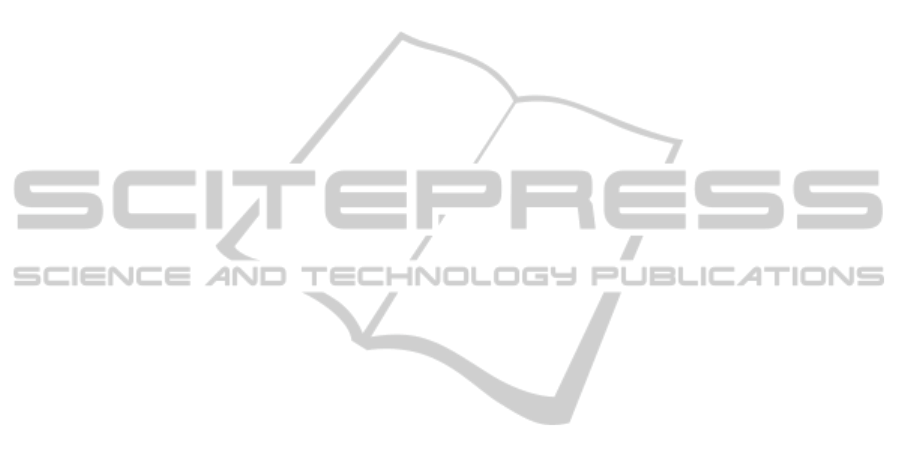
Optimal Experience: Psychological Studies of Flow in
Consciousness (pp. 15-35). Cambridge: Cambridge
University Press.
Csikszentmihalyi, M. (1990). Flow the Psychology of
Optimal Experience. New York: Harper & Row.
Csikszentmihalyi, M., & Csikszentmihalyi, I.S. (1988).
(Eds.), Optimal Experience: Psychological Studies of
Flow in Consciousness. Cambridge: Cambridge
University Press.
Csikszentmihalyi, M., & Larson, R. (1987). Validity and
reliability of the experience-sampling method. Journal
of Nervous & Mental Disease, 175, 525-536.
Csikszentmihalyi, M., Larson, R., & Prescott, S. (1977).
The ecology of adolescent experience. Journal of
Youth and Adolescence, 6, 281–294.
Csikszentmihalyi, M., & LeFevre, J. (1989). Optimal
experience in work and leisure. Journal of Personality
and Social Psychology, 56, 815-822.
De Freitas, S., & Neumann, T. (2009). The use of
‘exploratory learning’ for supporting immersive
learning in virtual environments. Computers &
Education, 52(2), 343-352.
Finneran, C. M., & Zhang, P. (2002). The challenges of
studying flow within a computer-mediated
environment. Paper presented at the Eighth Americas
Conference on Information Systems, Dallas, TX.
Ghani, J. (1995). Flow in human computer interactions:
test of a model. In J. Carey (Ed.), Human Factors in
Information Systems: Emerging Theoretical Bases (pp.
291-311). Norwood, New Jersey: Ablex Publishing
Corp.
Hoffman, D. L., & Novak, T. P. (1996). Marketing in
hypermedia computer-mediated environments:
conceptual foundations. Journal of Marketing, 60,
July, 50–68.
Konradt, U., Filip, R., & Hoffman, S. (2003). Flow
experience and positive affect during hypermedia
learning. British Journal of Educational Technology,
34 (3), 309-327.
Larson, R. (1988). Flow and writing. In M.
Csikszentmihalyi & I. S. Csikszentmihalyi, (Eds.),
Optimal Experience: Psychological Studies of Flow in
Consciousness (pp. 150-171). Cambridge: Cambridge
University Press.
Liao, L. (2006). A flow theory perspective on learner
motivation and behavior in distance education.
Distance Education, 27(1), 45-62.
Liu, C-C., Cheng, Y-B., Huang, C-W. (2011). The effect
of stimulation games on the learning of computational
problem solving. Computers & Education, 57(3),
1907-1918.
Massimini, F., & Carli, M. (1988). The systematic
assessment of flow in daily experience. In M.
Csikszentmihalyi & I. S. Csikszentmihalyi (Eds.),
Optimal experience: Psychological studies of flow in
consciousness (pp. 266–287). Cambridge, New York:
Cambridge University Press.
Novak, T. P., Hoffman, D. L., & Yung, Y. (2000).
Measuring the customer experience in online
environments: a structural modeling approach.
Marketing Science, 19(1), 22-42.
O’Broin, D., & Clarke, S. (2006). Inka: using flow to
enhance the mobile learning experience. In P. Isaias,
P. Kommers, & I. Arnedillo Sánchez, (Eds.),
Proceedings of the IADIS International Conference
Mobile Learning 2006 (Dublin, July 14 – 16, 2006),
139-146.
Pearce, J. M., Ainley, M. & Howard, S. (2005). The ebb
and flow of online learning. Computers in Human
Behavior, 21(5), 245-255.
Ryu, H., & Parsons, D. (2012). Risky business or sharing
the load? – Social flow in collaborative mobile
learning. Computers & Education, 58(2), 707-720.
Sato, I. (1988). Bosozoku: flow in Japanese motorcycle
gangs. In M. Csikszentmihalyi & I.S.
Csikszentmihalyi, (Eds.), Optimal Experience:
Psychological Studies of Flow in Consciousness (pp.
92-117). Cambridge: Cambridge University Press.
Shernoff, D. J., Csikszentmihalyi, M., Schneider, B., &
Steele Shernoff, E. (2003). Student engagement in
high school classrooms from the perspective of flow
theory. School Psychology Quarterly, 18(2), 158-176.
Shin, N. (2006). Online learner’s ‘flow’ experience: an
empirical study. British Journal of Educational
Technology, 37(5), pp 705-720.
Steuer, J. (1991). Defining Virtual Reality: Dimensions
Determining Telepresence. Journal of
Communication, 42(4), 73-93.
Voiskounsky, A. E. (2008). Flow experience in
cyberspace: Current studies and perspectives. In A.
Barak (Ed.), Psychological aspects of cyberspace:
Theory, research, applications (70-101). New York:
Cambridge University Press.
Weber, R., Tamborini, R., Westcott-Baker, A., Kantor, B.
(2009) Theorizing Flow and Media Enjoyment as
Cognitive Synchronization of Attentional and Reward
Networks. Communication Theory, 19, 397-422.
Webster, J., Trevino, L. K., and Ryan, L. (1993). The
dimensionality and correlates of flow in human-
computer interaction. Computers in Human Behavior
9(4), 411-426.
CSEDU2013-5thInternationalConferenceonComputerSupportedEducation
468