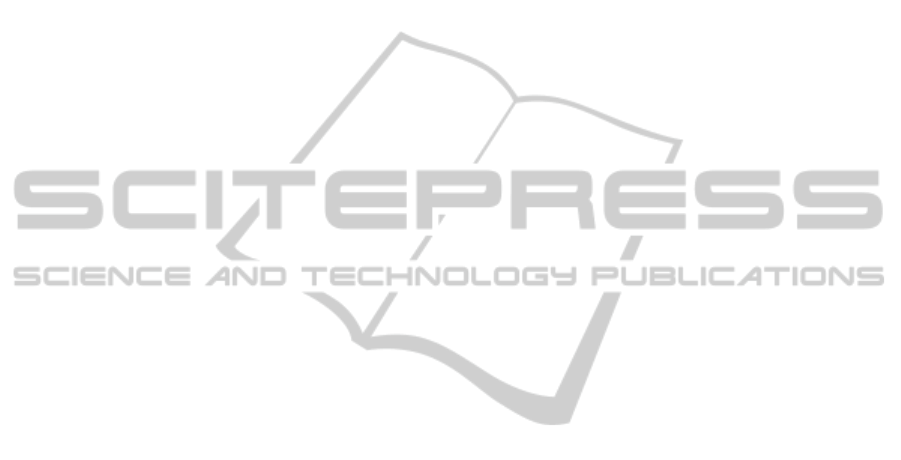
different. Given the lack of formalized leadership
hierarchies in most virtual organizations, leadership
at its most basic level is the ability to influence
others. Influence tactics can thus be viewed as a
vital–if not necessary–tool for members to get their
way in network issues (Greer and Jehn, 2009). This
dilemma–working at eye level without the formal
authority to give directives but getting work done
together at the same time–requires any form of
informal influence behavior. In the absence of
leadership alternatives, mutual influence is thus
beside joint decisions and collective processes not
only tolerated but often the only leadership
instrument available.
REFERENCES
Abele, S. (2011). Social interaction in cyberspace, Social
construction with few constraints. In Z. Birchmeier, B.
Dietz-Uhler & G. Stasser (Eds.), Strategic Uses of
Social Technology. An Interactive Perspective of
Social Psychology (pp. 84-107). New York:
Cambridge University Press.
Barbuto, J. E. & Moss, J. A. (2006). Dispositional Effects
in Intra-Organizational Influence Tactics: A Meta-
Analytic Review. Journal of Leadership &
Organizational Studies, 12(3), 30-48.
Barry, B. & Fulmer, I. S. (2004). The medium and the
message: The adaptive use of communication media in
dyadic influence. Academy of Management Review,
29, 272-292.
Blickle, G. (2003). Convergence of agents' and targets'
reports on intraorganizational influence attempts.
European Journal of Psychological Assessment, 19(1),
40-53.
Byrne, B. M. (2001). Structural Equation Modeling With
Amos: Basic Concepts, Applications, and
Programming. Mahwah, NJ: Erlbaum.
Campbell, D. T. & Fiske, D. W. (1959). Convergent and
discriminant validation by the multitrait-multimethod
matrix. Psychological Bulletin, 56, 81-105.
Driskell, J. E., Radtke, P. H. & Salas, E. (2003). Virtual
teams: Effects of technological mediation on team
performance. Group Dynamics: Theory, Research and
Practice, 7, 297–323.
Elron, E. & Vigoda-Gadot, E. (2006). Influence and
political processes in cyberspace: The case of global
virtual teams. International Journal of Cross-Cultural
Management, 6(3), 295-317.
Ferris, G., Hochwarter, W., Douglas, C., Blass, F.,
Kolodinsky, R. & Treadway, D. (2002). Research in
Personnel and Human Resources Management (pp.
65-127). Stanford: Elsevier.
Greer, L. L. & Jehn, K. A. (2009). Follow me: Strategies
used by emergent leaders in virtual organizations.
International Journal of Leadership Studies, 5, 102-
120.
Hu, L. & Bentler, P. M. (1999). Cutoff criteria for fit
indexes in covariance structure analysis: Conventional
criteria versus new alternatives. Structural Equation
Modeling, 6, 1-55.
Janneck, M., Staar, H. (2011). Playing Virtual Power
Games: Micro-political Processes in Inter-
organizational Networks. International Journal of
Social and Organizational Dynamics in Information
Technology, pp. 46-66.
Kline, R. B. (2005). Principles and practice of structural
equation modeling. New York: Guilford.
Latané, B., Liu, J. H., Nowak, A., Bonevento, M. &
Zheng, L. (1996). Distance Matters: Physical Space
and Social Impact. Personality & Social Psychology
Bulletin, 21, 795-805.
Marsh, H. W., Ballah, J. R. & MacDonald, R. (1988).
Goodness-of-fit indices in confirmatory factor
analysis: The effect of sample size. Psychological
Bulletin, 88, 245-258.
Okdie, B. M. & Guadagno, R. E. (2008). Social Influence
and Computer Mediated Communication. In K. St.
Amant & S. Kelsey (Eds.), Handbook of Research on
Computer Mediated Communication (pp. 477-491).
Hershey, PA: IGI Global.
Okdie, B. M., Guadagno, R. E., Bernieri, F. J., Geers, A. J.
& Mclarney-Vesotski, A. R. (2011). Getting to know
you: Face-to-face vs. online interactions. Computers in
Human Behavior, 27, 153-159.
Pitt, J., Kamara, L., Sergot, M. & Artikis, A. (2005).
Formalization of a voting protocol for virtual
organizations. In Proceedings of the Fourth
international Joint Conference on Autonomous Agents
and Multiagent Systems (pp. 373-380). New York:
ACM.
Sussman, L., Adams, A., Kuzmits, F. & Raho, L., (2002).
Organizatonial politics: tactics, channels, and
hierarchical roles. Journal of business ethics. 40(4),
313-331.
Tidwell, L. C. & Walther, J. B. (2002). Computer-
mediated communication effects on disclosure,
impressions, and interpersonal evaluations. Human
Communication Research, 3, 317-348.
Travica, B. (2005). Virtual organization and electronic
commerce. SIGMIS Database 36(3), 45–68.
Wilson, E. (2003). Perceived effectiveness of
interpersonal persuasion strategies in computer-
mediated communication. Computers in Human
Behavior, 19(5), 537-552.
Yukl, G. & Falbe, C. M. (1990). Influence tactics and
objectives in upward, downward and lateral influence
attempts. Journal of Applied Psychology, 75(2), 132-
140.
Self-otherAgreementonInfluenceAttemptsinVirtualOrganizations-DoAgentsandPeersSeeEyetoEye?
559