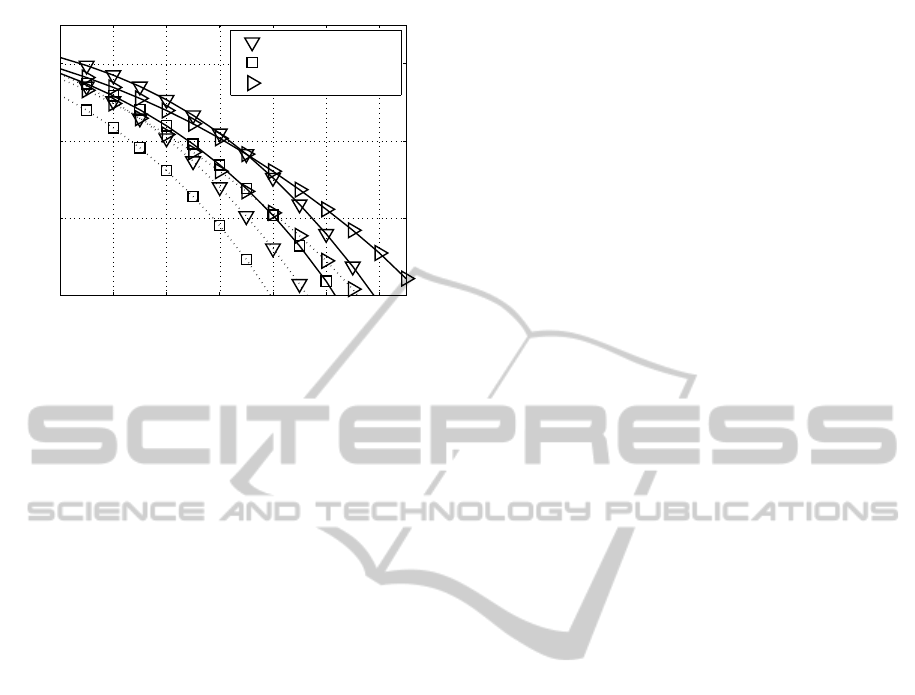
12 14 16 18 20 22 24
10
−8
10
−6
10
−4
10
−2
10 ·lg(E
s
/N
0
) (indB) →
bit-error rate →
no Correlation
weak Correlation
strong Correlation
Figure 14: BER with optimal PA (dotted line) and without
PA (solid line) when using the (64,4,0, 0 QAM transmis-
sion mode and transmitting 8 bit/s/Hz over frequency non-
selective (4×4) MIMO channels with different degrees of
correlation.
taining predominant (strong and weak) layers and in-
fer the MIMO channel behaviour. The best perfor-
mance is obtained when all CCDF coincide (are the
same) or are quite close. CCDF curve dispersion re-
veals the existence of predominant layer lowering the
MIMO performance. Additionally, in order to miti-
gate correlation effects the investigation has analyzed
the effect of bit and transmit power allocation along
the various MIMO layers as techniques for improv-
ing channel performance even in the presence of an-
tennas correlation. Regarding the power allocation,
a basic technique has been applied in order to obtain
the same quality along the different activated layers,
i.e., the same SNR at each detector. This technique
allows obtaining a higher performance. Moreover, bit
loading has been studied through the description of
some profiles (transmission modes) dealing to differ-
ent constellation per layer (bit per symbol interval)
but maintaining the overall transmission rate. A re-
markable conclusion is that activating all the MIMO
layers not necessarily provides the best performance
as highlighted in the results where the transmission
modes (64,4,0, 0) and (16,16, 0,0) present the best
performance. In order to highlight the importance of
this fact the probability of using each transmission
mode was analyzed and the previous conclusion was
remarked.
REFERENCES
Ahrens, A. and Lange, C. (2008). Modulation-Mode and
Power Assignment in SVD-equalized MIMO Sys-
tems. Facta Universitatis (Series Electronics and En-
ergetics), 21(2):167–181.
Cano-Broncano, F., Benavente-Peces, C., Ahrens, A.,
Ortega-Gonzalez, F. J., and Pardo-Martin, J. M.
(2013). Analysis of MIMO Systems with Transmitter-
Side Antennas Correlation. In International Con-
ference on Pervasive and Embedded Computing
and Communication Systems (PECCS), Barcelona
(Spain).
Haykin, S. S. (2002). Adaptive Filter Theory. Prentice Hall,
New Jersey.
Kalet, I. (1987). Optimization of Linearly Equalized
QAM. IEEE Transactions on Communications,
35(11):1234–1236.
K¨uhn, V. (2006). Wireless Communications over MIMO
Channels – Applications to CDMA and Multiple An-
tenna Systems. Wiley, Chichester.
Lee, W.-Y. (1973). Effects on Correlation between two Mo-
bile Radio Base-Station Antennas. IEEE Transactions
on Vehicular Technology, 22(4):130–140.
Mutti, C. and Dahlhaus, P. (2004). Adaptive Power Load-
ing for Multiple-Input Multiple-Output OFDM Sys-
tems with Perfect Channel State Information. In Joint
COST 273/284 Workshop on Antennas and Related
System Aspects in Wireless Communications, pages
93–98, Gothenburg.
Oestges, C. (2006). Validity of the Kronocker Model for
MIMO Correlated Channels. In Vehicular Technology
Conference, volume 6, pages 2818–2822, Melbourne.
Proakis, J. G. (2000). Digital Communications. McGraw-
Hill, Boston.
Salz, J. and Winters, J. H. (1994). Effect of Fading Correla-
tion on adaptive Arrays in digital Mobile Radio. IEEE
Transactions on Vehicular Technology, 43(4):1049–
1057.
Shiu, D., Foschini, G., Gans, M., and Kahn, J. (2000). Fad-
ing Correlation and its Effect on the Capacity of Mul-
tielement Antenna Systems. IEEE Transactions on
Communications, 48(3):502–513.
Wong, C. Y., Cheng, R. S., Letaief, K. B., and Murch, R. D.
(1999). Multiuser OFDM with Adaptive Subcarrier,
Bit, and Power Allocation. IEEE Journal on Selected
Areas in Communications, 17(10):1747–1758.
Zhou, Z., Vucetic, B., Dohler, M., and Li, Y. (2005). MIMO
Systems with Adaptive Modulation. IEEE Transac-
tions on Vehicular Technology, 54(5):1073–1096.
WINSYS2013-InternationalConferenceonWirelessInformationNetworksandSystems
232