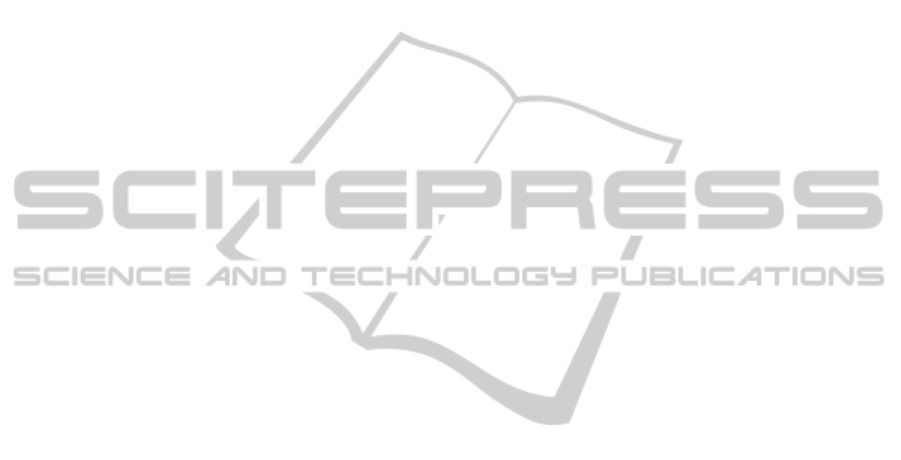
Amelung, B., & Viner, D. (2006). Mediterranean Tourism:
Exploring the Future with the Tourism Climatic Index.
Journal of Sustainable Tourism, 14: 349-366.
Amelung, B., Nicholls, S., & Viner, D. (2007).
Implications of Global Climate Change for Tourism
Flows and Seasonality. Journal of Travel Research 45:
285-296.
Bigano, A., Hamilton, J. M., & Tol, R. S. J. (2006). The
impact of climate holiday destination choice. Climatic
Change, 76, 389-406.
Bigano. A, Hamilton, J. M., Maddison, D. J., & R. S. J.
Tol (2006). Predicting tourism flows under climate
change an editorial comment on Gössling and Hall
(2006). Climatic Change, 79:175-180.
Breiling, M., & Charamza, P. (1999). The impact of global
warming on winter tourism and skiing: a regionalised
model for Austrian snow conditions. Regional
Environmental Change, 1: 4-14.
Bujosa, A., & Rosselló, J. (2013). Climate change and
summer mass tourism: the case of Spanish domestic
tourism. Climatic Change 117: 363 - 375.
CIA, Central Intelligence Agency (2012). The World
Factbook. Accessed 19th June 2012 at: https://
www.cia.gov/library/publications /the-world-fact
book/fields/2012.html
Crouch, G. I. (1994). The Study of International Tourism
Demand: A Survey of Practice Journal of Travel
Research, 32: 41-55.
Elsasser, H., & Messerli, P. (2001). The vulnerability of
the snow industry in the Swiss Alps. Journal of
Mountain Research and Development, 21: 335-339.
Englebert, E. T., McDermott, C., & Kleinheinz, G. T.
(2008). Effects of the nuisance algae, Cladophora, on
Escherichia coli at recreational beaches in Wisconsin.
Science of the Total Environment, 404, 10-17.
Eugenio-Martín, J. L., & Campos-Soria, J. A. (2010).
Climate in the region of origin and destination choice
in outbound tourism demand. Tourism Management,
31: 744 -751
Eugenio-Martín, J. L., (2003). Modelling determinants of
tourism demand as a five-stage process: A discrete
choice methodological approach. Tourism and
Hospitality Research, 4(4):341-354.
Goh, C. (2012). Exploring impact of climate on tourism
demand. Annals of Tourism Research 39: 1859-1883.
Gössling, S., & Hall, C. (2006). Uncertainties in predicting
tourist flows under scenarios of climate change.
Climatic Change, 79: 163-173.
Gössling, S., Bredberg, M., Randow, M., Sandström, E., &
Svensson, P. (2006) Tourist Perceptions of Climate
Change: A Study of International Tourists in Zanzibar.
Current Issues in Tourism, 9: 419-435
Gössling, S., Lindén, O., Helmersson, J., Liljenberg, J., &
Quarm, S. (2007). Diving and global environmental
change: a Mauritius case study. In B. Garrod & S.
Gössling (Eds.), New frontiers in marine tourism.
Amsterdam: Elsevier.
Gössling, S., Scott, D., Hall, C. M., Ceron, J.P., & Dubois,
G. (2012). Uncertainties in predicting tourist flows
under scenarios of climate change. Annals of Tourism
Research, 39: 36-58.
Hamilton, J., & Tol, R.S.J. (2007). The impact of climate
change on tourism in Germany, the UK and Ireland: A
simulation study. Regional Environmental Change, 7:
161-172.
Hamilton, J. M., Maddison, D., & Tol, R.S.J. (2005a).
Climate change and international tourism: A
simulation study. Global Environmental Change, 15:
253-266.
Hamilton, J. M., Maddison, D., and Tol, R.S.J. (2005b).
The effects on climate change on international
tourism. Climate Research, 29: 245-254.
Harrison, S. J., Winterbottom, S.J., and Shephard, C.
(1999). The potential effects of climate change on the
Scottish tourist industry. Tourism Management, 20:
203-211.
Hoegh-Guldberg, O., Mumby, P. J., Hooten, A. J.,
Steneck, R. S., Greenfield, P., Gomez, E., Harvell, D.
R., Sale, P. F., Edwards, A. J., Caldeira, K. Knowlton,
N., Eakin, C. M., Iglesias Prieto, R., Muthiga, N.,
Bradbury, R. H., Dubi, A. & Hatziolos, M. E. (2007).
Coral Reefs Under Rapid Climate Change and Ocean
Acidification. Science, 318: 1737-1742.
IPCC, Intergovernamental Panel on Climate Change
(2007). Fourth Assessment Report: Climate Change
2007 (AR4). Working Group II Report "Impacts,
Adaptation and Vulnerability".
Kulendran, N., & Dwyer, L. (2012). Modeling seasonal
variation in tourism flows with climate variables.
Tourism Analysis, 17: 121-137.
Lancaster, K.L. (1966). A New Approach to Consumer
Theory. Journal of Political Economy, 74(2): 132-157.
Li, G., Song, H., & Witt, S. F. (2005). Recent
developments in econometric modeling and
forecasting. Journal of Travel Research, 44, 82-99.
Lim, C. (1999). A Meta analysis review of International
tourism demand. Journal of Travel Research, 37, 273-
284.
Lin, T.P., & Matzarakis, A. (2011). Tourism climate
.information based on human thermal perception in
Taiwan and Eastern China. Tourism Management, 32:
492-500.
Maddison, D. (2001). In search of warmer climates? The
impact of climate change on flows of British tourist.
Climatic Change, 49: 193-208.
Marcussen, C.H. (2011). Understanding Destination
Choices of German Travelers. Tourism Analysis, 16:
649-662.
Matzarakis, A., Mayer, H. & Iziomon, M. G. (1999).
Applications of a universal thermal index:
Physiological equivalent temperature. International
Journal of Biometeorology 43(2):76–84.
Mieczkowski, Z. (1985). The tourism climatic index: A
method of evaluating world climates for tourism.
Canadian Geographer, 29 : 220-233.
Moreno, A. & Amelung, B. (2009). Climate Change and
Tourist Comfort on Europe's Beaches in Summer: A
Reassessment. Coastal Management, 37: 550-568.
Morgan, R., Gatell, E., Junyent, R., Micallef, A., Ozhan,
E., & Williams, A. T. (2000). An improved user-based
QuantitativeModelsEvaluatingtheEffectClimateChangeEffectsonTourism-StateoftheArt
487