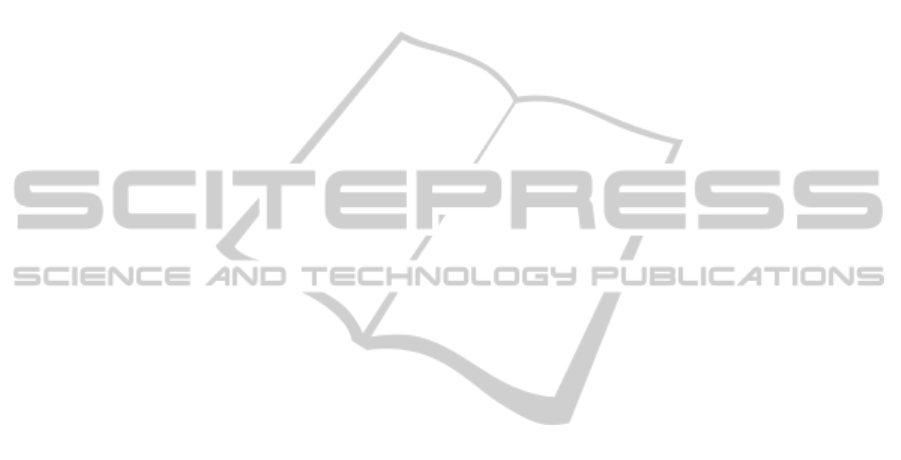
Bates, C. et al., 2011. Overprescription of postoperative
narcotics: a look at postoperative pain medication
delivery, consumption and disposal in urological
practice. The Journal of urology, 185(2), pp.551–555.
Brownstein, J. S. et al., 2010. Geographic information
systems and pharmacoepidemiology: using spatial
cluster detection to monitor local patterns of
prescription opioid abuse. Pharmacoepidemiology and
drug safety, 19(6), pp.627–637.
Butler, S. F. et al., 2007. Internet surveillance: content
analysis and monitoring of product-specific internet
prescription opioid abuse-related postings. The
Clinical journal of pain, 23(7), pp.619–628.
Centers for Disease Control and Prevention, 2012.
Prescription Drug Abuse and Overdose: Public Health
Perspective. Available at: www.cdc.gov/primarycare/
materials/opoidabuse/docs/pda-phperspective-508.pdf
(Accessed May 3, 2013).
Compton, W. M. & Volkow, N. D., 2006. Major increases
in opioid analgesic abuse in the United States:
concerns and strategies. Drug and alcohol
dependence, 81(2), pp.103–108.
Crum, R. M., 2005. Epidemiology of opioid use, abuse,
and dependence. The treatment of opioid dependence,
p.43.
Dasgupta, N., Mandl, K. D. & Brownstein, J. S., 2009.
Breaking the news or fueling the epidemic? Temporal
association between news media report volume and
opioid-related mortality. PloS one, 4(11), p.e7758.
Drug Enforcement Administration, 2012. DEA’s Fifth
National Prescription Drug Take-Back Day Results in
Another Big Haul. News Release. Available at:
www.justice.gov/dea/docs/results_final.pd (Accessed
May 3, 2013).
Fishbain, D.A. et al., 2008. What Percentage of Chronic
Nonmalignant Pain Patients Exposed to Chronic
Opioid Analgesic Therapy Develop Abuse/Addiction
and/or Aberrant Drug-Related Behaviors? A
Structured Evidence-Based Review. Pain Medicine,
9(4), pp.444–459.
Food and Drug Administration, 2013. Extended-release
(ER) and long-acting (LA) opioid analgesics risk
evaluation and mitigation strategy (REMS), Available
at:
http://www.fda.gov/drugs/drugsafety/informationby-
drugclass/ucm163647.htm (Accessed May 3, 2013).
Gilson, A., 2012. Personal Communication: Findings of
Madison WI Drug Take-Back Day.
Gosin, M., Marsiglia, F. F. & Hecht, M. L., 2003.
Keepin’it REAL: a drug resistance curriculum tailored
to the strengths and needs of pre-adolescents of the
southwest. Journal of Drug Education, 33(2), pp.119–
142.
Hall, A. J. et al., 2008. Patterns of abuse among
unintentional pharmaceutical overdose fatalities.
JAMA: the journal of the American Medical
Association, 300(22), pp.2613–2620.
Levy, M. S., 2007. An exploratory study of OxyContin use
among individuals with substance use disorders.
Journal of psychoactive drugs, 39(3), pp.271–276.
Sterman, J., 2000. Business dynamics: systems thinking
and modeling for a complex world, Boston:
Irwin/McGraw-Hill.
Sterman, J. D., 2006. Learning from evidence in a
complex world. Journal Information, 96(3). Available
at: http://ajph.aphapublications.org/doi/abs/10.2105/
AJPH.2005.066043 (Accessed May 3, 2013).
SAMHSA - Substance Abuse and Mental Health Services
Administration, 2006. Results from the 2005 national
survey on drug use and health: national findings,
Rockville: Department of Health and Human Services.
Available at: http://oas.samhsa.gov/nsduh/2k5nsduh/
2k5results.pdf.
SAMHSA - Substance Abuse and Mental Health Services
Administration, 2012. Results from the 2011 National
Survey on Drug Use and Health: Summary of National
Findings. Available at: http://www.samhsa.gov/
data/2k11/WEB_SR_088/WEB_SR_088.pdf
(Accessed February 26, 2013).
Wakeland, W., Fitzgerald, J. & Haddox, J. D., 2010. Key
data gaps for understanding trends in prescription
opioid analgesic abuse and diversion among chronic
pain patients and nonmedical users. In College on
Problems of Drug Dependence, 72nd Annual
Scientific Meeting. Scottsdale, AZ.
Warner, M. et al., 2011. Drug Poisoning Deaths in the
United States, 1980-2008, Centers for Disease Control
and Prevention.
Winkler, D. et al., 2004. Estimating the relative efficiency
of various forms of prevention at different stages of a
drug epidemic. Socio-Economic Planning Sciences,
38(1), pp.43–56.
Young, A. M. & Havens, J. R., 2012. Transition from first
illicit drug use to first injection drug use among rural
Appalachian drug users: a cross-sectional comparison
and retrospective survival analysis. Addiction, 107(3),
pp.587–596.
SIMULTECH2013-3rdInternationalConferenceonSimulationandModelingMethodologies,Technologiesand
Applications
564