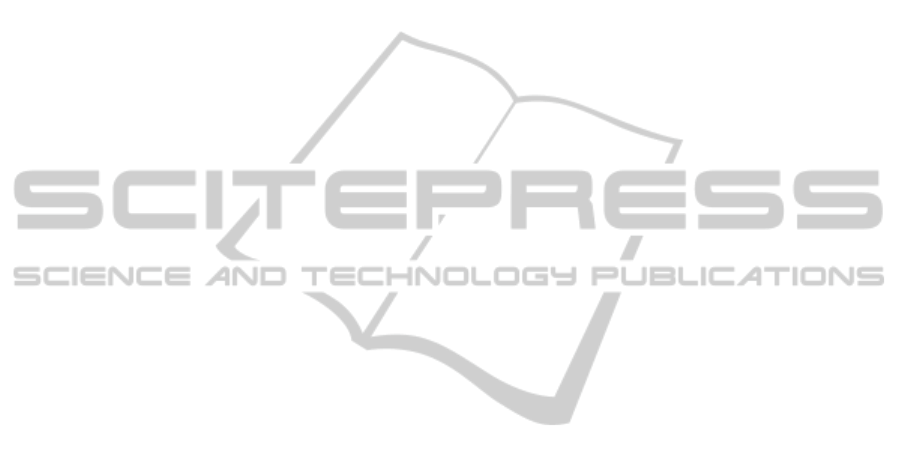
to these mission environments; therefore it is
important to state that these models and the previous
considerations could be effectively tailored and
applied also in relation to civil scenarios where new
investment (i.e. industries and infrastructures) have
to be plan over a domestic region or a district as
well as during promotional campaign in marketing
initiatives.
2 SIMULATION OBJECTIVES
Therefore the international context in unstable areas
introduce a good motivation to investigate these
population models (i.e.country reconstruction
operations); currently there it is expected that these
models could be very useful for evaluating
alternative planning considering risk, opportunities,
times, resources costs over a complex and stochastic
framework, vice versa the predictive capability of
these simulators is still pretty limited due to the
high degree of uncertainty affecting human elements
and the high influence of specific spot events
(Bruzzone and Massei, 2010). Due to these
considerations, the proposed agent-driven
simulations are devoted to conduct experimental
analysis and decision support by providing reliable
estimations and useful risk analysis, but not the of
the exact time and location of a new riot; indeed
these events are generated usually by an ignition
factor that is highly unpredictable (i.e. a single
phrase or shot in a specific moment).
Considering the proposed context it is evident
that nowadays military mission environments,
especially within countries characterized by different
cultures, society organization and changeable
political situations, require a new approach to
tactical and strategic operations which not only
appreciates military engagements, but also
relationship between civil population, military forces
as well as community evolution and interest groups.
The problem of this analysis is that there are not
universally accepted simulation models and that the
human behaviour modifiers (HBM) are very difficult
to be represented; in addition it is even necessary to
create models of specific operations that are
currently not covered by the existing simulators in
order to take of Civil-Military Cooperation,
INFOPS, PSYOPS as well as of psychological
consequences over population during mission
execution; therefore some existing model/simulator
is currently taking into account these not-kinetic
operations, but usually it is just a qualitative on/off
parameters or a manual script affecting the scenario
evolution; this obviously don’t allow to consider the
complex dynamic of the interaction among different
interest groups that is the basis for situation
evolution.
3 APPLICATION FRAMEWORK
AND PROPOSED APPROACH
The simulator should consider for instance that
digging a well within an area could generate positive
effects on some part of population (i.e. people hired
to carried out the work, owner of the land) as well as
negative effects on other ones (i.e. opposite clan
respect well owner, opposite political party respect
that one involved); these actions generated direct
impact on element of the population living in the
area as well as on the their related interest group and
in addition produce a cascade effect on all the social
networks among people and interest groups. In
addition if due to weather conditions and/or lack of
resource the well constructions result to be affected
by delays this could produce negative impact on the
people that expect the completion to get benefits of
this asset.
All these elements as well as the cascade of
effects could result positive or negative with a strong
influence due to the dynamically evolving
relationships among people and interest groups and
also due to the importance of the specific actions,
the cultural background and the communications
(Seck et al., 2005).
Indeed the diffusion in the region and among the
people and interest groups of the effects of the
actions is modelled based on communications over
different supports (face to face, media, phones) and
considering specific factors; therefore these
communications introduces attenuation factors and
delays; due to the computational workload (i.e. in
our case 300’000 people and 60 interest groups) the
cascade effect could slow down simulation on single
workstations, for this reason it is possible to run the
simulation with correct diffusion models or by
considering that the diffusion happen with fixed
stochastic delays along each single operation phase
(this reduces of drastically the events to be
considered); considering multiple actions on going
concurrently and the main interest to measure final
effects this simplification resulted acceptable,
therefore if computation power is available it is
possible to run the simulator using more correct
models.
In the proposed models it was required to model
these elements and to create a simulation able to
SocialLayersandPopulationModelsDirectedbyIntelligentAgentsforEstimatingtheImpactofOperationsand
Investments
413