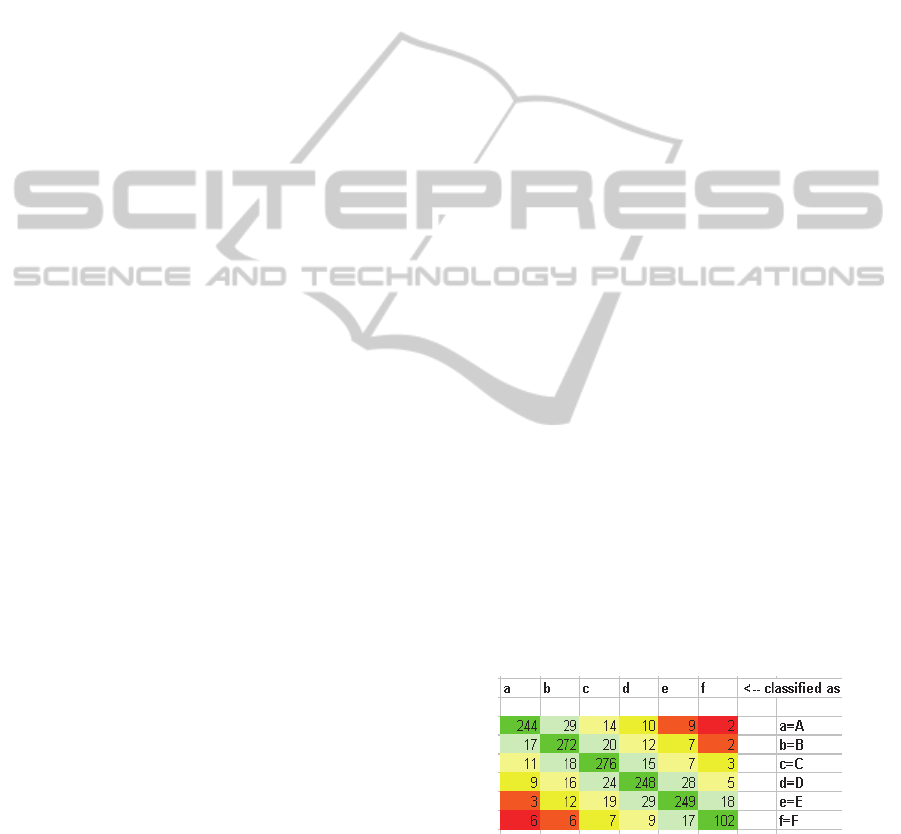
have been tried with varying success. Some well-
known schemes and their representations include:
ID3 which uses decision trees (Quinlan, 1986),
FOIL which uses rules (Quinlan, 1990), PROTOS
which is a case-based classifier (Porter et al., 1990),
and the instance-based learners IB1-IB5 (Aha et al.,
1991); (Aha, 1992). These schemes have
demonstrated excellent classification accuracy over
a large range of domains.
In this work we will use the entropy as a distance
measure which provides a unified approach to
dealing with these problems. Specifically we will
use the K-Star (Cleary and Trigg, 1995), an
instance-based learner which uses such a measure.
Instance-based learners classify an instance by
comparing it to a database of pre-classified
examples. The fundamental assumption is that
similar instances will have similar classifications.
The question lies in how to define “similar instance”
and “similar classification”.
Nearest neighbour algorithms (Cover and Hart,
1967) are the simplest of instance-based learners.
They use certain domain specific distance functions
to retrieve the single most similar instance from the
training set. The classification of the retrieved
instance is given as the classification for the new
instance. Edited nearest neighbour algorithms (Hart,
1968); (Gates, 1972) are selective and in these
instances are stored in the database and used in
classification. The k nearest neighbours of the new
instance are retrieved and whichever class is
predominant amongst them is given as the new
instance's classification. A standard nearest
neighbour classification is the same as a k-nearest
neighbour classifier for which k=1.
One of the advantages of the approach we are
following here is that both real attributes and
symbolic attributes can be dealt with together within
the same framework.
For K-Star we have to choose values for the
blending parameter. The behaviour of the distance
measure as these parameters change is very
interesting. Consider the probability function for
symbolic attributes as “s” changes. With a value of
“s” close to 1, instances with a symbol different to
the current one will have a very low transformation
probability, while instances with the same symbol
will have a high transformation probability. Thus the
distance function will exhibit nearest neighbour
behaviour. As “s” approaches 0, the transformation
probability directly reflects the probability
distribution of the symbols, thus favouring symbols
which occur more frequently. This behaviour is
similar to the default rule for many learning schemes
which is simply to take whichever classification is
most likely (regardless of the new instance's attribute
values). As “s” changes, the behaviour of the
function varies smoothly between these two
extremes. The distance measure for real valued
attributes exhibits the same properties.
So, the K-Star algorithm chooses a value for
“blending” (mentioned “s” parameter) by selecting a
number between n0 and N. Thus selecting n0 gives a
nearest neighbour algorithm and choosing N gives
equally weighted instances. For convenience the
number is specified by using the “blending
parameter” b, which varies from b= 0% (for n0) and
b=100% for N, with intermediate values interpolated
linearly.
We think of the selected number as a “sphere of
influence”, specifying how many of the neighbours
should be considered important (although there is
not a harsh cut off at the edge of the sphere—more
of a gradual decreasing in importance).
The underlying technique solves the smoothness
problem and we believe contributes strongly to its
good overall performance. The underlying theory
also allows clean integration of both symbolic and
real valued attributes and a principled way of
dealing with missing values (in this case symbolic
attributes were used).
2.2.2 Attribute Selection
For attribute selection, all five minutes windows
were labelled to defined six groups: “A” for the first
30 minutes driving, “B” for the next 30 minutes, and
so on until “F” for the last 30 minutes.
So, based on the WEKA (Waikato Environment
for Knowledge Analysis, version 3.6.6) tool and the
previously mentioned K-Star classifier, 15
parameters are shown to have the best confusion
matrix to classify all five minutes windows:
Figure 7: Confusion matrix.
The following figure shows the summary and the
detailed accuracy for each group, using “10-fold
cross validation” method for validation:
HeartRateVariability-KnowingMoreaboutHRVAnalysisandFatigueinTransportStudies
111