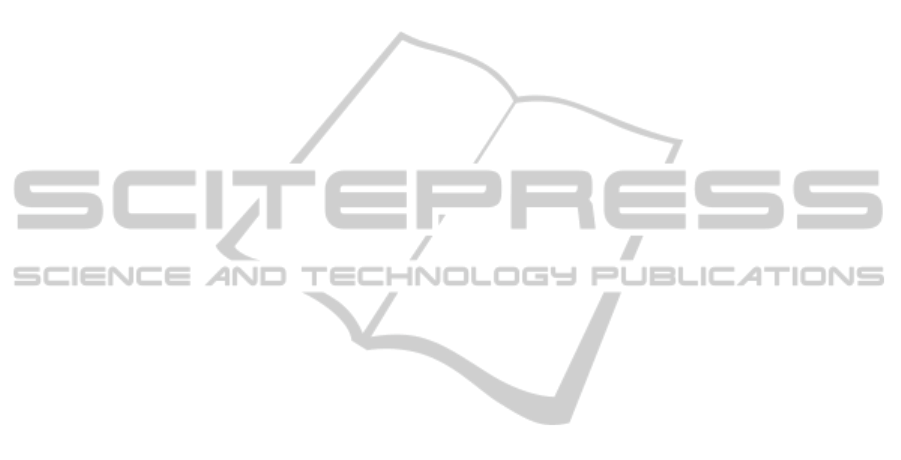
4 DISCUSSION
This paper showed, based on a multiscale simulation
model and data on one representative tremor patient,
that the supraspinal oscillations that mediate tremor
are a common cortical projection to the motor neuron
pool, as indirectly suggested in previous works com-
puting EEG-EMG coherence. Furthermore it puts
forward, based on physiologically plausible simula-
tions, that the presence of a common tremor drive to
the motor neuron pool may cause abnormally large
motor unit synchronization, as largely hypothesized
for tremor. Experimental results in one representa-
tive ET patient suggest that this abnormally large syn-
chronization may be systematically found in patients,
which would indirectly prove the existence of a strong
common synaptic input related to tremor.
It must be noticed that the coherence values found
in the model were notably larger than those observed
in the patient. We believe that the reason for this is
(at least) twofold. First, in the model the tremor was a
narrowband colored noise that was directly projected
to all motor neurons in the pool, which implies that it
neglects the spectral distortion that interneurons intro-
duce in the transmission of supraspinal oscillations,
given their nonlinear response (Gerstner and Kistler,
2002) (Negro and Farina, 2011). Second, in the model
the central (supraspinal) tremor was directly avail-
able, while for the case of the patient we had to as-
sess its contribution from the EEG. Indeed, the am-
plitude spectrum of the EEG recorded in this patient
did not shown a peak at the tremor frequency, oppo-
site to the clear contribution observed in the case of
the model. Remarkably, low coherence values are re-
ported in other works studying EEG-EMG coherence
in tremor patients (Raethjen et al., 2007) (Volkmann
et al., 1996) (Timmermann et al., 2003).
As to motor neuron synchronization, for the
model we showed that if a strong common tremor in-
put was projected to the entire motor neuron popula-
tion, the motor unit spike trains exhibited abnormally
synchronization. Interestingly, synchronization was
remarkably large for all tremor frequencies and lev-
els of concurrent voluntary contraction. In the case
of the patient, we observed that motor unit synchro-
nization as computed with the CIS was more than
two times larger than previously reported for healthy
counterparts. Thus, given the well-established causal
relationship between synchronization and common
synaptic inputs (Kirkwood and Sears, 1978) we con-
sider this as a further proof of the existence of a com-
mon cortical input to the motor neuron pool related to
tremor.
ACKNOWLEDGEMENTS
This work has been funded by the EU Commission
through grants EU-FP7-2007-224051 (TREMOR)
and EU-FP7-2011-287739 (NeuroTREMOR).
REFERENCES
Baker, J. R., Davey, N. J., Ellaway, P. H., and Friedland,
C. L. (1992). Short-term synchrony of motor unit dis-
charge during weak isometric contraction in parkin-
son’s disease. Brain, 115 Pt 1:137–154.
Benito-Le
´
on, J. and Louis, E. D. (2006). Essential tremor:
emerging views of a common disorder. Nature clinical
practice. Neurology, 2(12):666–78;.
Christakos, C. N., Erimaki, S., Anagnostou, E., and Anasta-
sopoulos, D. (2009). Tremor-related motor unit firing
in parkinson’s disease: implications for tremor gene-
sis. J Physiol, 587(Pt 20):4811–4827.
Das Gupta, A. (1963). Paired response of motor units dur-
ing voluntary contraction in parkinsonism. J Neurol
Neurosurg Psychiatry, 26:265–268.
Deuschl, G., Bain, P., and Brin, M. (1998). Consensus state-
ment of the movement disorder society on tremor. ad
hoc scientific committee. Mov Disord, 13 Suppl 3:2–
23.
Deuschl, G., Raethjen, J., Lindemann, M., and Krack, P.
(2001). The pathophysiology of tremor. Muscle &
Nerve, 24:716–735.
Dideriksen, J., Enoka, R., and Farina, D. (2011). A model
of the surface electromyogram in pathological tremor.
IEEE Trans Biomed Eng.
Dietz, V., Hillesheimer, W., and Freund, H. J. (1974). Corre-
lation between tremor, voluntary contraction, and fir-
ing pattern of motor units in parkinson’s disease. J
Neurol Neurosurg Psychiatry, 37(8):927–937.
Elble, R. J. and Deuschl, G. (2009). An update on essential
tremor. Neurol Neurosci Reports, 9:273–7.
Elek, J. M., Dengler, R., Konstanzer, A., Hesse, S.,
and Wolf, W. (1991). Mechanical implications of
paired motor unit discharges in pathological and vol-
untary tremor. Electroencephalogr Clin Neurophysiol,
81(4):279–283.
Ellaway, P. H. (1978). Cumulative sum technique and
its application to the analysis of peristimulus time
histograms. Electroencephalogr Clin Neurophysiol,
45(2):302–304.
Farina, D., Holobar, A., Merletti, R., and Enoka, R. M.
(2010). Decoding the neural drive to muscles from
the surface electromyogram. Clin Neurophysiol,
121(10):1616–1623.
Farina, D. and Merletti, R. (2004). Estimation of av-
erage muscle fiber conduction velocity from two-
dimensional surface emg recordings. J Neurosci
Methods, 134(2):199–208.
Fuglevand, A. J., Winter, D. A., and Patla, A. E. (1993).
Models of recruitment and rate coding organization in
motor-unit pools. J Neurophysiol, 70(6):2470–2488.
MotorUnitPropertiesandUnderlyingDeterminantsinPathologicalTremor
137