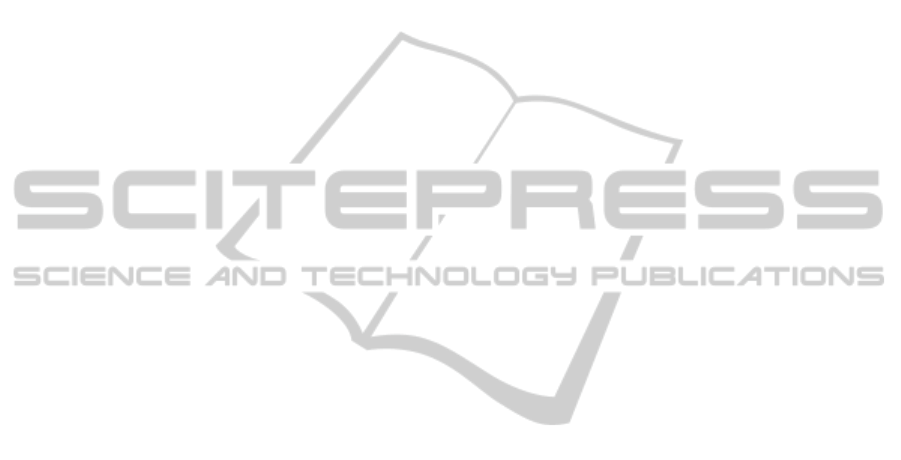
and synthesis of laboratory research. Psychological
Bulletin, 130(3):355–391.
Fairclough, S. H. (2009). Fundamentals of physiological
computing. Interacting with Computers, 21:133–145.
Healey, J. and Picard, R. (2005). Detecting Stress During
Real-World Driving Tasks Using Physiological Sen-
sors. IEEE Transactions on Intelligent Transportation
Systems, 6(2):156–166.
Hellhammer, J. and Schubert, M. (2012). The physiological
response to Trier Social Stress Test relates to subjec-
tive measures of stress during but not before or after
the test. Psychoneuroendocrinology, 37(1):119–24.
Hewig, J., Schlotz, W., Gerhard, F., Breitenstein, C.,
Luerken, A., and Naumann, E. (2008). Associations
of the cortisol awakening response (CAR) with corti-
cal activation asymmetry during the course of an exam
stress period. Psychoneuroendocrinology, 33:83–89.
Hogervorst, M. A., Brouwer, A.-M., and Vos, W. K.
(2013). Physiological Correlates of Stress in Indi-
viduals About to Undergo Eye Laser Surgery. In 5th
IEEE International Conference on Affective Comput-
ing and Intelligent Interaction, Geneva, Switzerland.
IEEE Computer Society Press.
Khosrowabadi, R. and Quek, C. (2011). A Brain-Computer
Interface for classifying EEG correlates of chronic
mental stress. In Proceedings of International Joint
Conference on Neural Networks 2011 (IJCNN), vol-
ume 138632, pages 757–762.
Kirchner, W. K. (1958). Age differences in short-term re-
tention of rapidly changing information. Journal of
Experimental Psychology, 55(4):352–358.
Kirschbaum, C., Pirke, K.-M., and Hellhammer, D. H.
(1993). The ”Trier Social Stress Test”: a Tool for
Investigating Psychobiological Stress Responses in a
Laboratory Setting. Neuropsychobiology, 28:76–81.
Krout, R. E. (2007). Music listening to facilitate relaxation
and promote wellness: Integrated aspects of our neu-
rophysiological response to music. The Arts in Psy-
chotherapy, 34:134–141.
Kusserow, M., Amft, O., and Troster, G. (2012). Monitoring
Stress-arousal in the Wild - Measuring the Intangible.
IEEE Pervasive Computing, 12(2):28 – 37.
Lewis, R. S., Weekes, N. Y., and Wang, T. H. (2007).
The effect of a naturalistic stressor on frontal EEG
asymmetry, stress, and health. Biological Psychology,
75:224–239.
Loggia, M. L., Juneau, M., and Bushnell, M. C. (2011).
Autonomic responses to heat pain: Heart rate, skin
conductance, and their relation to verbal ratings and
stimulus intensity. Pain, 152(3):592–8.
Regehr, C., Glandy, D., and Pitts, A. (2013). Interventions
to reduce stress in university students: A review and
meta-analysis. Journal of Affective Disorders, 148:1–
11.
Reinhardt, T., Schmahl, C., W
¨
ust, S., and Bohus, M. (2012).
Salivary cortisol, heart rate, electrodermal activity and
subjective stress responses to the Mannheim Multi-
component Stress Test (MMST). Psychiatry research,
198(1):106–11.
Renard, Y., Lotte, F., Gibert, G., Congedo, M., Maby, E.,
Delannoy, V., Bertrand, O., and L
´
ecuyer, A. (2010).
OpenViBE: An Open-Source Software Platform to
Design, Test, and Use Brain-Computer Interfaces in
Real and Virtual Environments. Presence: Teleopera-
tors and Virtual Environments, 19(1):35–53.
Riera, A., Soria-Frisch, A., Albajes-Eizagirre, A., Ci-
presso, P., Grau, C., Dunne, S., and Ruffini, G.
(2012). Electro-physiological data fusion for stress
detection. Studies in health technology and informat-
ics, 181:228–32.
Selye, H. (1936). A syndrome produced by diverse nocuous
agents. Nature, 138:32.
Sinha, R., Talih, M., Malison, R., Cooney, N., Anderson,
G.-M., and Kreek, M.-J. (2003). Hypothalamic-
pituitary-adrenal axis and sympatho-adreno-
medullary responses during stress-induced and
drug cue-induced cocaine craving states. Psychophar-
macology, 170:62–72.
Spielberger, C. D., Gorsuch, R. L., and Lushene, R. E.
(1970). Manual for the State-Trait Anxiety Inventory.
Consulting Psychologists Press, Palo Alto.
Szalma, J. L. and Teo, G. W. L. (2012). Spatial and tem-
poral task characteristics as stress: A test of the dy-
namic adaptability theory of stress, workload, and per-
formance. Acta Psychologica, 139:448–471.
Taniguchi, K., Nishikawa, A., Sugino, T., Aoyagi, S., Seki-
moto, M., Takiguchi, S., Okada, K., Monden, M., and
Miyazaki, F. (2009). Method for Objectively Eval-
uating Psychological Stress Resulting when Humans
Interact with Robots. In Kulyukin, V. A., editor, Ad-
vances in Human-Robot Interaction. InTech China.
Tops, M., van Peer, J. M., Wester, A. E., Wijers, A. A., and
Korf, J. (2006). State-dependent regulation of corti-
cal activity by cortisol: An EEG study. Neuroscience
Letters, 404:34–39.
van den Broek, E. L. and Westerink, J. H. D. M. (2012).
Biofeedback Systems for Stress Reduction - Towards
a Bright Future for a Revitalized Field. In Interna-
tional Conference on Health Informatics (HEALTH-
INF 2012), pages 499–504.
PhyCS2014-InternationalConferenceonPhysiologicalComputingSystems
106