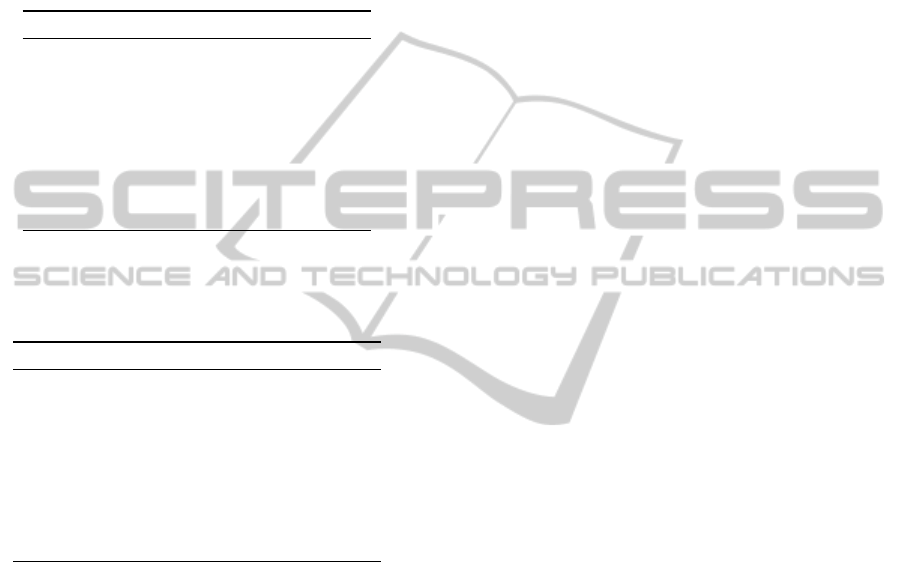
the size of the tomatoes is modeled and incorporated
as regularization terms, leading to better robustness.
It is supposed that the operator selects, at the end of
the process for each image, the ellipse correspond-
ing to the best elliptic estimation of the actual contour.
Table 1: Mean (µ) and standard deviation (σ) of D
meanR
and
D
maxR
by comparing ellipse Ell
f 4
with the manual segmen-
tation M. Only the images belonging to category 1 (i.e. with
a low amount of occlusion) have been considered.
N
1
S
µ
D
meanR
σ
D
meanR
µ
D
maxR
σ
D
maxR
S = 1 26 1.72 0.77 5.06 2.76
S = 2 4 1.85 0.46 5.45 1.91
S = 3 21 3.40 2.24 9.79 6.88
S = 4 14 2.73 1.92 7.81 5.71
S = 5 5 4.81 1.30 13.05 3.56
S = 6 0 - - - -
S = 7 25 1.88 0.65 4.81 1.97
S = 8 20 6.07 5.75 15.41 10.61
S = 9 1 5.26 0.00 11.86 0.00
S = 10 5 2.25 0.56 6.59 2.25
Table 2: Mean (µ) and standard deviation (σ) of D
meanR
and
D
maxR
by comparing ellipse Ell
opt
with the manual segmen-
tation M. Only the images belonging to category 1 (i.e. with
a low amount of occlusion) have been considered.
N
1
S
µ
D
meanR
σ
D
meanR
µ
D
maxR
σ
D
maxR
S = 1 26 1.34 0.68 3.76 2.21
S = 2 4 1.57 0.4 4.43 0.88
S = 3 21 2.87 2.05 8.4 6.58
S = 4 14 2.2 1.77 6.28 5.34
S = 5 5 4.54 1.14 12.44 3.46
S = 6 0 - - - -
S = 7 25 1.7 0.49 4.61 1.62
S = 8 20 5.4 4.88 14.9 10.37
S = 9 1 5.24 0 11.86 0
S = 10 5 1.75 0.36 4.4 1.25
The segmentation of tomatoes is a challenging
task due to the presence of occlusion and variation
in contrast. In order to evaluate the robustness of the
proposed algorithm, the entire image set was divided
into three categories based on the amount of occlu-
sion. For the images with an acceptable level of occlu-
sion, good results were obtained with an average vari-
ation in D
meanR
less than 6%. Also, the low standard
deviation for D
meanR
indicates the robustness of the
proposed algorithm. Good results with D
meanR
< 10%
were obtained on 44% of the images which contain a
significant amount of occlusion.
For the moment, it has been assumed that an oper-
ator manually selects one ellipse as the final segmen-
tation. In future work, we wish to provide automati-
cally the best representation of the tomato. Also, in
some images, the position of the tomato is not de-
tected correctly due to the presence of other toma-
toes nearby. This could be improved by updating the
position of the tomato globally by considering also
the movement of adjacent tomatoes. One possible
improvement for the active contour model is to re-
strict the size of the reference ellipse, as there is little
growth between two consecutive images.
ACKNOWLEDGEMENTS
This work is partially supported by European Re-
gional Development Fund (ERDF).
REFERENCES
Aggelopoulou, A., Bochtis, D., Fountas, S., Swain, K.,
Gemtos, T., and Nanos, G. (2011). Yield prediction
in apple orchards based on image processing. Journal
of Precision Agriculture, 12:448–456.
Aitkenhead, M., Dalgetty, I., Mullins, C., McDonald, A.,
and Strachan, N. (2003). Weed and crop discrimi-
nation using image analysis and artificial intelligence
methods. Computers and Electronics in Agriculture,
39(3):157 – 171.
Charmi, M., Ghorbel, F., and Derrode, S. (2009). Using
Fourier-based shape alignment to add geometric prior
to snakes. In ICASSP, pages 1209–1212.
Du, C. and Sun, D. (2006). Learning techniques used in
computer vision for food quality evaluation: a review.
Journal of Food Engineering, 72(1):39 – 55.
Fischler, A. and Bolles, C. (1981). Random sample consen-
sus: a paradigm for model fitting with applications to
image analysis and automated cartography. Commu-
nications of the ACM, 24(6):381–395.
Foulonneau, A., Charbonnier, P., and Heitz, F. (2006).
Affine-invariant geometric shape priors for region-
based active contours. IEEE Transactions on Pattern
Analysis and Machine Intelligence, 28(8):1352–1357.
Jayas, D., Paliwal, J., and Visen, N. (2000). Review pa-
per (automation and emerging technologies): Multi-
layer neural networks for image analysis of agricul-
tural products. Journal of Agricultural Engineering
Research, 77(2):119 – 128.
Kass, M., Witkin, A., and Terzopoulos, D. (1988). Snakes:
Active contour models. International Journal of Com-
puter Vision, 1(4):321–331.
Lee, W. S., Slaughter, D. C., and Giles, D. K. (1999).
Robotic weed control system for tomatoes. Precision
Agriculture, 1:95–113.
Mkhabela, M., Bullock, P., Raj, S., Wang, S., and Yang,
Y. (2011). Crop yield forecasting on the Canadian
prairies using MODIS NDVI data. Agricultural and
Forest Meteorology, 151(3):385 – 393.
Narendra, V. G. and Hareesh, K. S. (2010). Prospects of
computer vision automated grading and sorting sys-
tems in agricultural and food products for quality eval-
uation. International Journal of Computer Applica-
tions, 1(4):1–9.
ICPRAM2014-InternationalConferenceonPatternRecognitionApplicationsandMethods
410