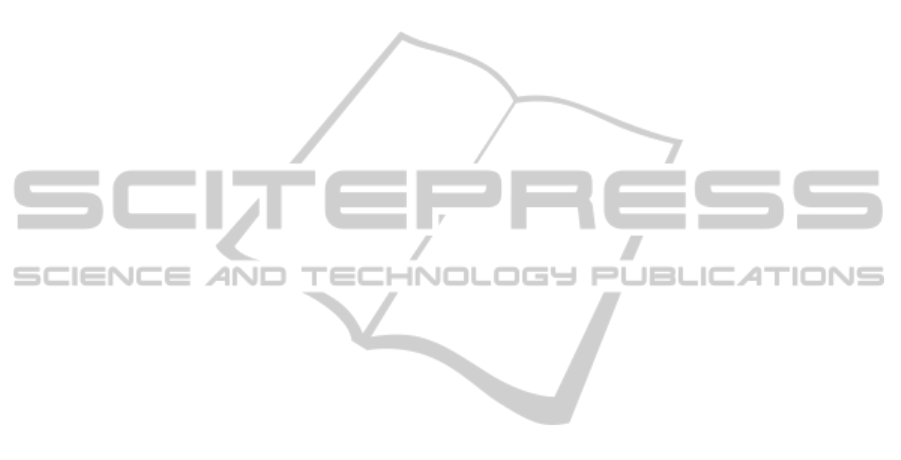
Finally, reliability and accuracy of analysis (X34)
was a positively affected criteria and stakeholders’
satisfaction (X33) was a negatively affected criterion
in factor F6. The key criterion of factor F6 was
found to be “reliability and accuracy of analysis
(X34)”.
5 CONCLUSIONS
Nowadays, various types of enterprise systems (ES)
have been used by organizations to enhance
competitive advantage through data integration and
analysis in real environment. Due to this fact that
these systems are presented as one of the integral
part of organizational decision making process,
evaluating BI for enterprise systems and determining
the importance-level of each intelligent tools is so
important to create decision support environment for
managers in decision-making process. In this study,
after reviewing on prior BI evaluation model, by
considering BI in viewpoint of system-enabler, an
evaluation model based on hybrid model containing
fuzzy logic and DEMATEL technique was
developed. In here, fuzzy DEMATEL method was
fully described. Based on proposed method, the
factors and criteria were assessed through expert
committee, all responses were aggregated and
finally, the total relation matrix of each factor was
acquired. Then, with considering expert opinions,
the threshold values for each factor were determined
in order to identifying significant relationship
between criteria of each factor and removing
insignificant relationships. Here, a new evaluation
model was developed using hybrid concept to assess
importance-level and influence level of each criteria.
Furthermore, the key criterions of each factor were
determined in terms of intelligence for enterprise
system. So, further researches are needed to rich
cause and effects model by gathering universal data.
Applying other MCDM methods in a fuzzy
environment to arranging BI evaluation criteria, and
comparing the results of these methods is also
recommended for future research.
REFERENCES
Alter, S. 2004. A Work System View Of Dss In Its Fourth
Decade. Decision Support Systems, 38, 319-327.
Bucher, T., Gericke, A. & Sigg, S. 2009. Process-Centric
Business Intelligence. Business Process Management
Journal, 15, 408-429.
Büyüközkan, G. & Çifçi, G. 2012. A Novel Hybrid Mcdm
Approach Based On Fuzzy Dematel, Fuzzy Anp And
Fuzzy Topsis To Evaluate Green Suppliers. Expert
Systems With Applications, 39, 3000-3011.
Chang, T. H. & Wang, T. C. 2009. Using The Fuzzy
Multi-Criteria Decision Making Approach For
Measuring The Possibility Of Successful Knowledge
Management. Information Sciences, 179, 355-370.
Cheng, H., Lu, Y.-C. & Sheu, C. 2009. An Ontology-
Based Business Intelligence Application In A
Financial Knowledge Management System. Expert
Systems With Applications, 36, 3614-3622.
Chou, Y. C., Sun, C. C. & Yen, H. Y. 2011. Evaluating
The Criteria For Human Resource For Science And
Technology (Hrst) Based On An Integrated Fuzzy Ahp
And Fuzzy Dematel Approach. Applied Soft
Computing.
Doumpos, M. & Zopounidis, C. 2010. A Multicriteria
Decision Support System For Bank Rating. Decision
Support Systems, 50, 55-63.
Duan, Y., Ong, V. K., Xu, M. & Mathews, B. 2011.
Supporting Decision Making Process With “Ideal”
Software Agents–What Do Business Executives
Want? Expert Systems With Applications.
Fontela, E. & Gabus, A. 1976. The Dematel Observer.
Battelle Institute, Geneva Research Center.
Ghazanfari, M., Jafari, M. & Rouhani, S. 2011. A Tool To
Evaluate The Business Intelligence Of Enterprise
Systems. Scientia Iranica.
Gunasekaran, A. & Ngai, E. W. T. 2012. Decision Support
Systems For Logistics And Supply Chain
Management. Decision Support Systems, 52, 777-778.
Hu, H. Y., Chiu, S. I., Cheng, C. C. & Yen, T. M. 2011.
Applying The Ipa And Dematel Models To Improve
The Order-Winner Criteria: A Case Study Of
Taiwan’s Network Communication Equipment
Manufacturing Industry. Expert Systems With
Applications, 38, 9674-9683.
Isik, O., Jones, M. C. & Sidorova, A. 2011. Business
Intelligence (Bi) Success And The Role Of Bi
Capabilities. Intelligent Systems In Accounting,
Finance And Management.
Kimball, R. & Ross, M. 2002. The Data Warehouse
Toolkit: The Complete Guide To Dimensional
Modelling. New York Ua.
Kristianto, Y., Gunasekaran, A., Helo, P. & Sandhu, M.
2012. A Decision Support System For Integrating
Manufacturing And Product Design Into The
Reconfiguration Of The Supply Chain Networks.
Decision Support Systems, 52, 790-801.
Lee, Y. C., Li, M. L., Yen, T. M. & Huang, T. H. 2010.
Analysis Of Adopting An Integrated Decision Making
Trial And Evaluation Laboratory On A Technology
Acceptance Model. Expert Systems With Applications,
37, 1745-1754.
Li, S. T., Shue, L. Y. & Lee, S. F. 2008. Business
Intelligence Approach To Supporting Strategy-Making
Of Isp Service Management. Expert Systems With
Applications, 35, 739-754.
Lin, Y. H., Tsai, K. M., Shiang, W. J., Kuo, T. C. & Tsai,
C. H. 2009. Research On Using Anp To Establish A
ICEIS2014-16thInternationalConferenceonEnterpriseInformationSystems
462